Visualizing Learning Space In Neural Network Hidden Layers
IVAPP: PROCEEDINGS OF THE 15TH INTERNATIONAL JOINT CONFERENCE ON COMPUTER VISION, IMAGING AND COMPUTER GRAPHICS THEORY AND APPLICATIONS, VOL 3: IVAPP(2020)
摘要
Analyzing and understanding how abstract representations of data are formed inside deep neural networks is a complex task. Among the different methods that have been developed to tackle this problem, multidimensional projection techniques have attained positive results in displaying the relationships between data instances, network layers or class features. However, these techniques are often static and lack a way to properly keep a stable space between observations and properly convey flow in such space. In this paper, we employ different dimensionality reduction techniques to create a visual space where the flow of information inside hidden layers can come to light. We discuss the application of each used tool and provide experiments that show how they can be combined to highlight new information about neural network optimization processes.
更多查看译文
关键词
Machine Learning, Neural Network Visualization, Deep Neural Networks
AI 理解论文
溯源树
样例
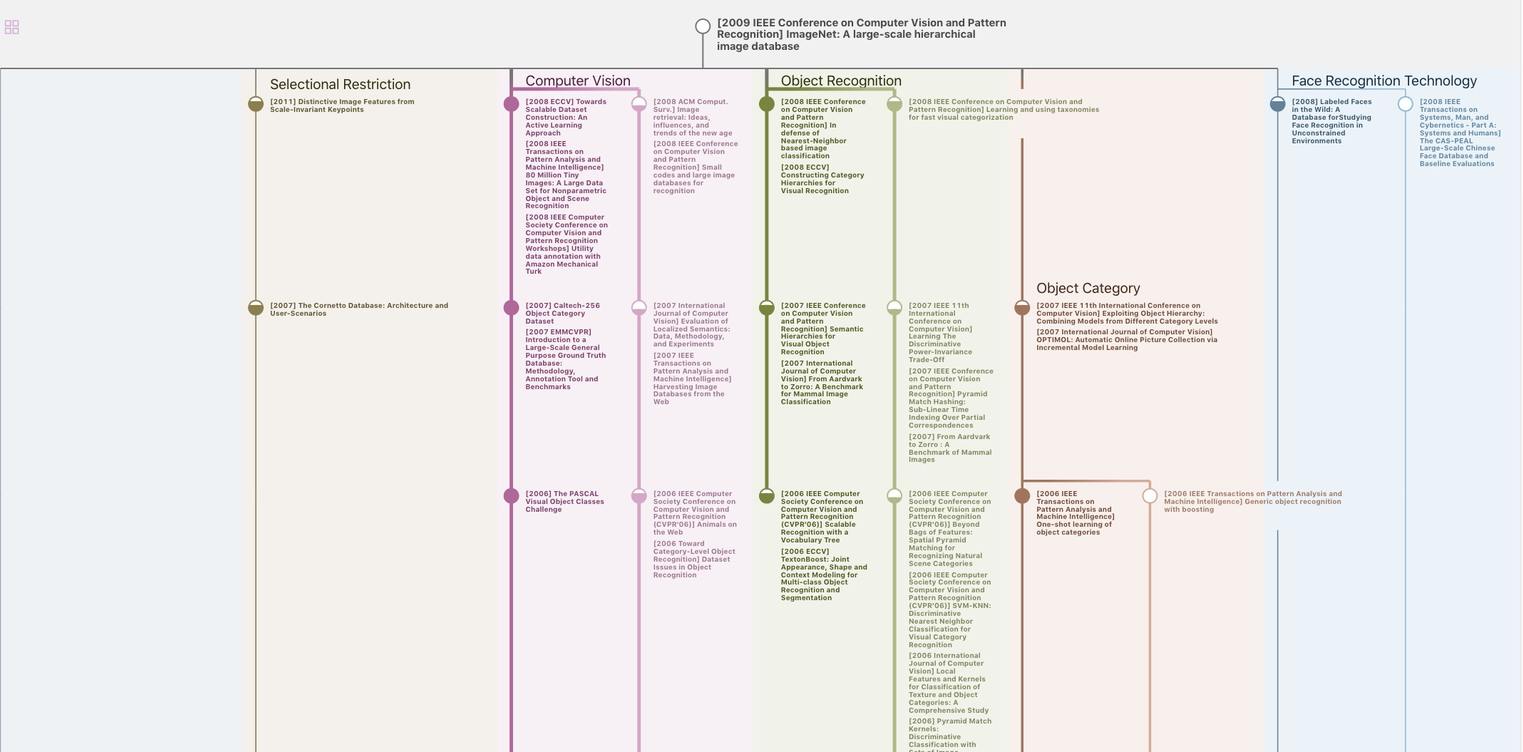
生成溯源树,研究论文发展脉络
Chat Paper
正在生成论文摘要