Reliable Trees: Reliability Informed Recursive Partitioning For Psychological Data
MULTIVARIATE BEHAVIORAL RESEARCH(2021)
摘要
Recursive partitioning, also known as decision trees and classification and regression trees (CART), is a machine learning procedure that has gained traction in the behavioral sciences because of its ability to search for nonlinear and interactive effects, and produce interpretable predictive models. The recursive partitioning algorithm is greedy-searching for the variable and the splitting value that maximizes outcome homogeneity. Thus, the algorithm can be overly sensitive to chance associations in the data, particularly in small samples. In an effort to limit chance associations, we propose and evaluate a reliability-based cost function for recursive partitioning. The reliability-based cost function increases the likelihood of selecting variables that are more reliable, which should have more consistent associations with the outcome of interest. Two reliability-based cost functions are proposed, evaluated through simulation, and compared to the CART algorithm. Results indicate that reliability-based cost functions can be beneficial, particularly with smaller samples and when more reliable variables are important to the prediction, but can overlook important associations between the outcome and lower reliability predictors. The use of these cost functions was illustrated using data on depression and suicidal ideation from the National Longitudinal Survey of Youth.
更多查看译文
关键词
Machine learning, CART, reliability
AI 理解论文
溯源树
样例
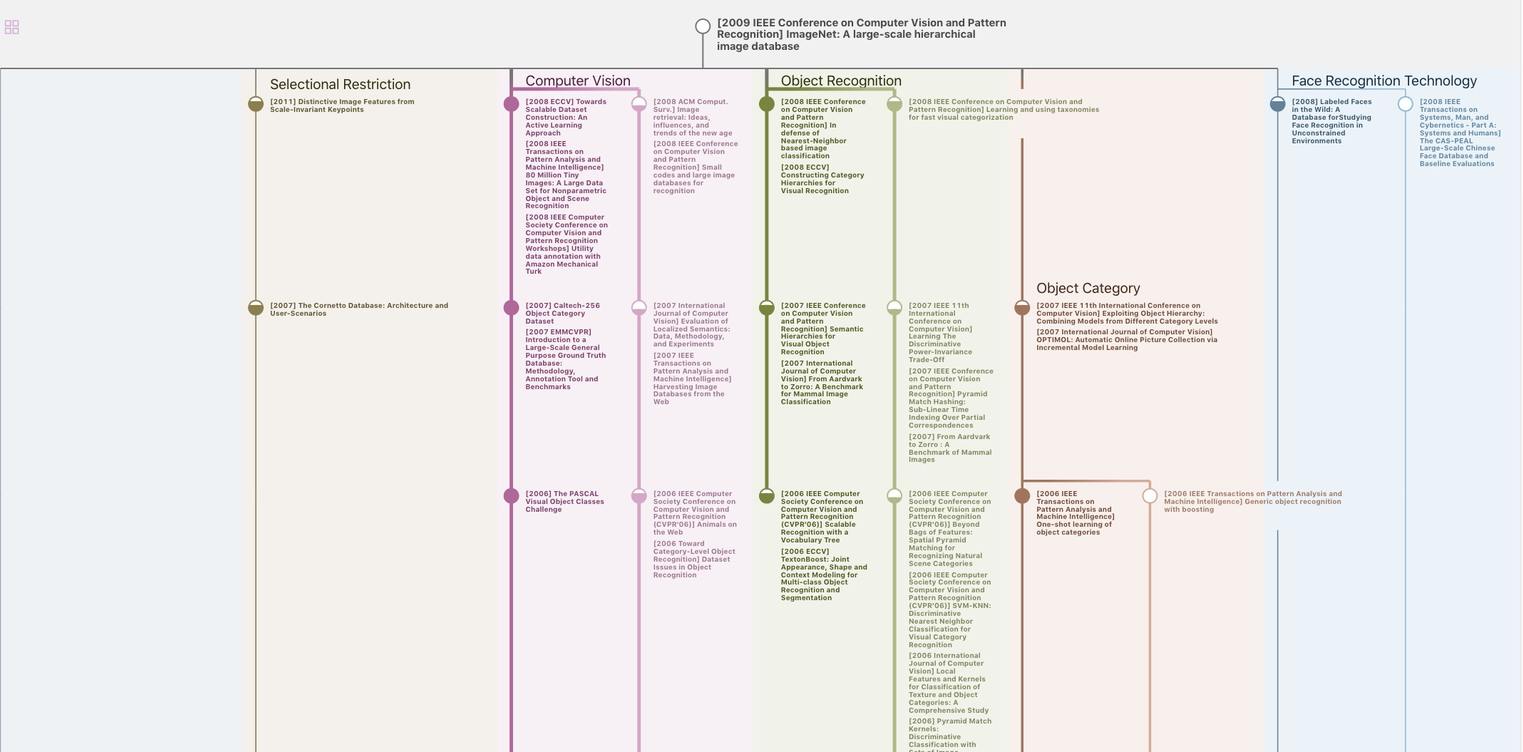
生成溯源树,研究论文发展脉络
Chat Paper
正在生成论文摘要