ResNet-SCDA-50 for Breast Abnormality Classification
IEEE/ACM Transactions on Computational Biology and Bioinformatics(2021)
摘要
AbstractAim: Breast cancer is the most common cancer in women and the second most common cancer worldwide. With the rapid advancement of deep learning, the early stages of breast cancer development can be accurately detected by radiologists with the help of artificial intelligence systems. Method: Based on mammographic imaging, a mainstream clinical breast screening technique, we present a diagnostic system for accurate classification of breast abnormalities based on ResNet-50. To improve the proposed model, we created a new data augmentation framework called SCDA (Scaling and Contrast limited adaptive histogram equalization Data Augmentation). In its procedure, we first conduct the scaling operation to the original training set, followed by applying contrast limited adaptive histogram equalisation (CLAHE) to the scaled training set. By stacking the training set after SCDA with the original training set, we formed a new training set. The network trained by the augmented training set, was coined as ResNet-SCDA-50. Our system, which aims at a binary classification on mammographic images acquired from INbreast and MINI-MIAS, classifies masses, microcalcification as “abnormal”, while normal regions are classified as “normal”. Results: We present the first attempt to use the image contrast enhancement method as the data augmentation method, resulting in an averaged 98.55 percent specificity and 92.83 percent sensitivity, which gives our best model an overall accuracy of 95.74 percent. Conclusion: Our proposed method is effective in classifying breast abnormality.
更多查看译文
关键词
Breast cancer, ResNet-50, contrast limited adaptive histogram equalization, classification
AI 理解论文
溯源树
样例
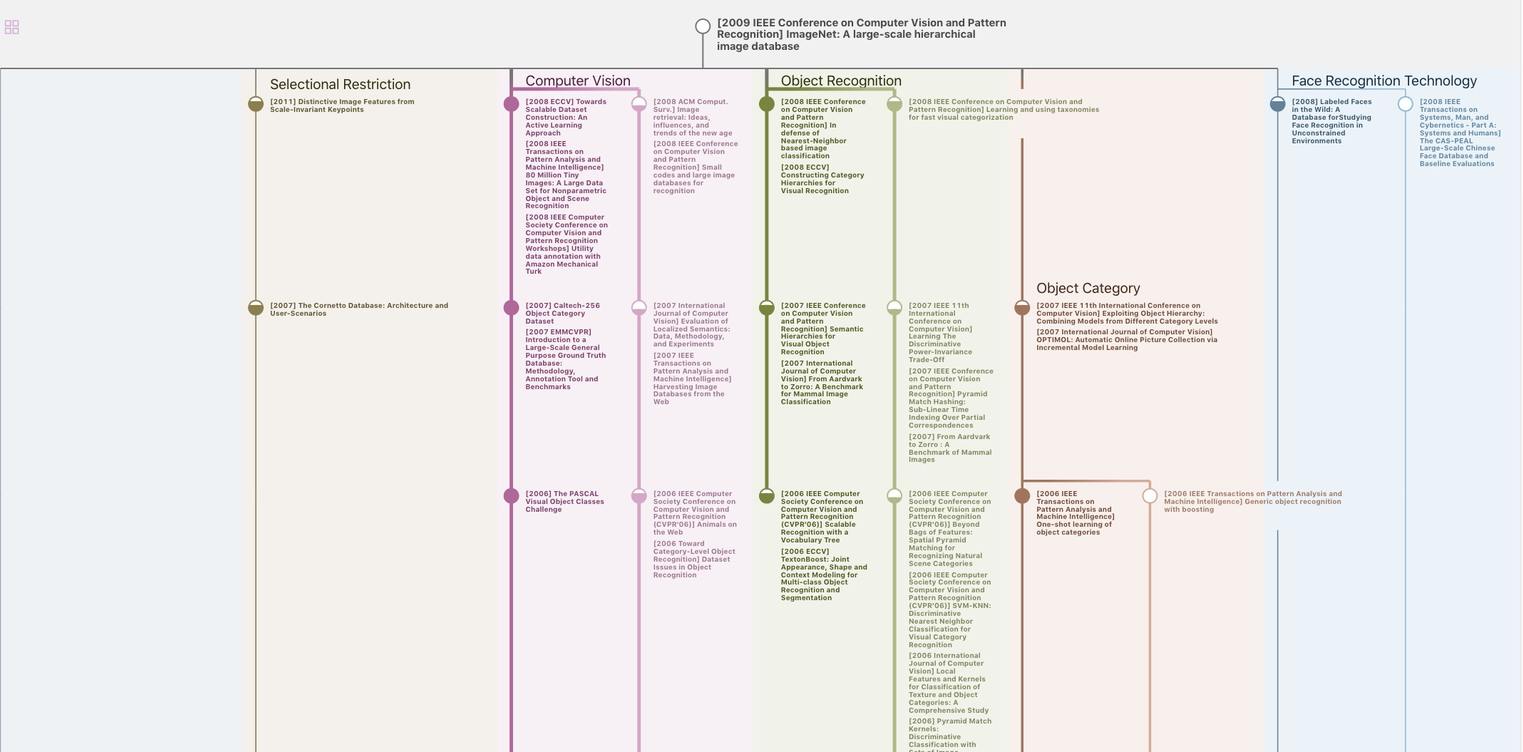
生成溯源树,研究论文发展脉络
Chat Paper
正在生成论文摘要