SPECTER: Document-level Representation Learning using Citation-informed Transformers
arxiv(2020)
摘要
Representation learning is a critical ingredient for natural language processing systems. Recent Transformer language models like BERT learn powerful textual representations, but these models are targeted towards token- and sentence-level training objectives and do not leverage information on inter-document relatedness, which limits their document-level representation power. For applications on scientific documents, such as classification and recommendation, the embeddings power strong performance on end tasks. We propose SPECTER, a new method to generate document-level embedding of scientific documents based on pretraining a Transformer language model on a powerful signal of document-level relatedness: the citation graph. Unlike existing pretrained language models, SPECTER can be easily applied to downstream applications without task-specific fine-tuning. Additionally, to encourage further research on document-level models, we introduce SCIDOCS, a new evaluation benchmark consisting of seven document-level tasks ranging from citation prediction, to document classification and recommendation. We show that SPECTER outperforms a variety of competitive baselines on the benchmark.(1)
更多查看译文
关键词
representation,document-level,citation-informed
AI 理解论文
溯源树
样例
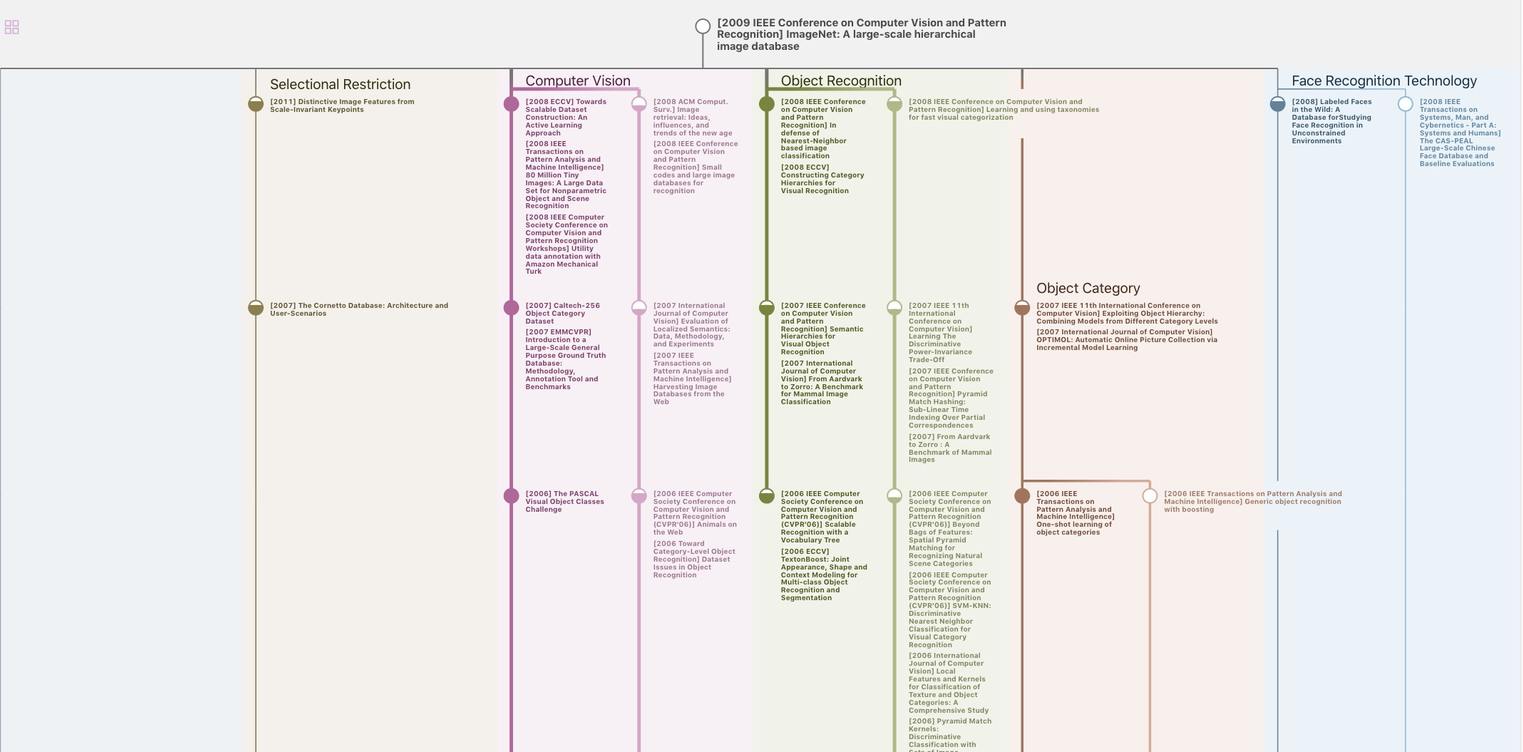
生成溯源树,研究论文发展脉络
Chat Paper
正在生成论文摘要