Footprints and Free Space From a Single Color Image
2020 IEEE/CVF Conference on Computer Vision and Pattern Recognition (CVPR)(2020)
摘要
Understanding the shape of a scene from a single color image is a formidable computer vision task. However, most methods aim to predict the geometry of surfaces that are visible to the camera, which is of limited use when planning paths for robots or augmented reality agents. Such agents can only move when grounded on a traversable surface, which we define as the set of classes which humans can also walk over, such as grass, footpaths and pavement. Models which predict beyond the line of sight often parameterize the scene with voxels or meshes, which can be expensive to use in machine learning frameworks. We introduce a model to predict the geometry of both visible and occluded traversable surfaces, given a single RGB image as input. We learn from stereo video sequences, using camera poses, per-frame depth and semantic segmentation to form training data, which is used to supervise an image-to-image network. We train models from the KITTI driving dataset, the indoor Matterport dataset, and from our own casually captured stereo footage. We find that a surprisingly low bar for spatial coverage of training scenes is required. We validate our algorithm against a range of strong baselines, and include an assessment of our predictions for a path-planning task.
更多查看译文
关键词
single color image,computer vision,augmented reality agents,machine learning,visible surfaces,occluded traversable surfaces,single RGB image,path planning,image to image network,surface geometry,stereo video sequences,camera poses,semantic segmentation,footprints,free space
AI 理解论文
溯源树
样例
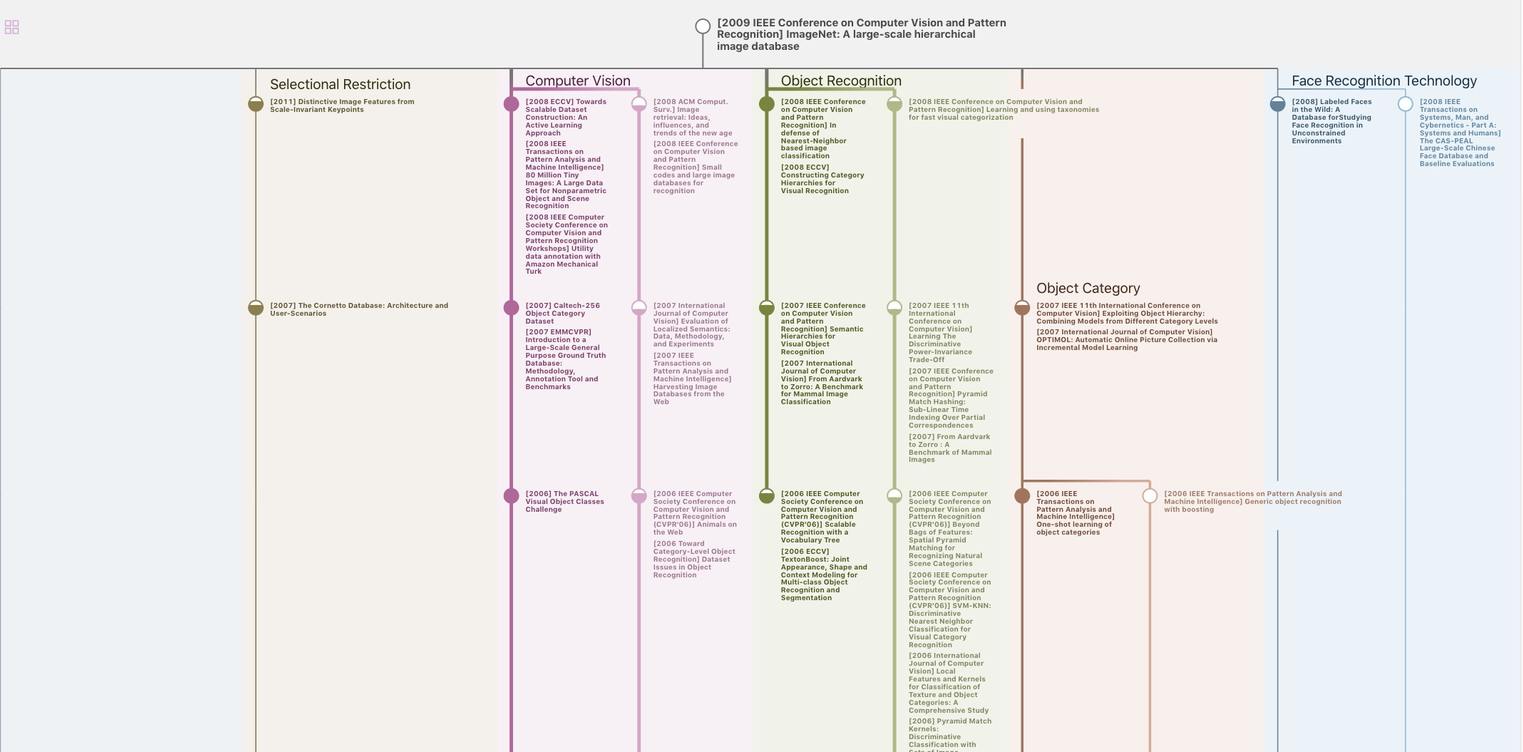
生成溯源树,研究论文发展脉络
Chat Paper
正在生成论文摘要