Frequency-Guided Word Substitutions for Detecting Textual Adversarial Examples
EACL(2021)
摘要
While recent efforts have shown that neural text processing models are vulnerable to adversarial examples, comparatively little attention has been paid to explicitly characterize their effectiveness. To overcome this, we present analytical insights into the word frequency characteristics of word-level adversarial examples for neural text classification models. We show that adversarial attacks against CNN-, LSTM- and Transformer-based classification models perform token substitutions that are identifiable through word frequency differences between replaced words and their substitutions. Based on these findings, we propose frequency-guided word substitutions (FGWS) as a simple algorithm for the automatic detection of adversarially perturbed textual sequences. FGWS exploits the word frequency properties of adversarial word substitutions, and we assess its suitability for the automatic detection of adversarial examples generated from the SST-2 and IMDb sentiment datasets. Our method provides promising results by accurately detecting adversarial examples, with $F_1$ detection scores of up to 93.7% on adversarial examples against BERT-based classification models. We compare our approach against baseline detection approaches as well as a recently proposed perturbation discrimination framework, and show that we outperform existing approaches by up to 15.1% $F_1$ in our experiments.
更多查看译文
AI 理解论文
溯源树
样例
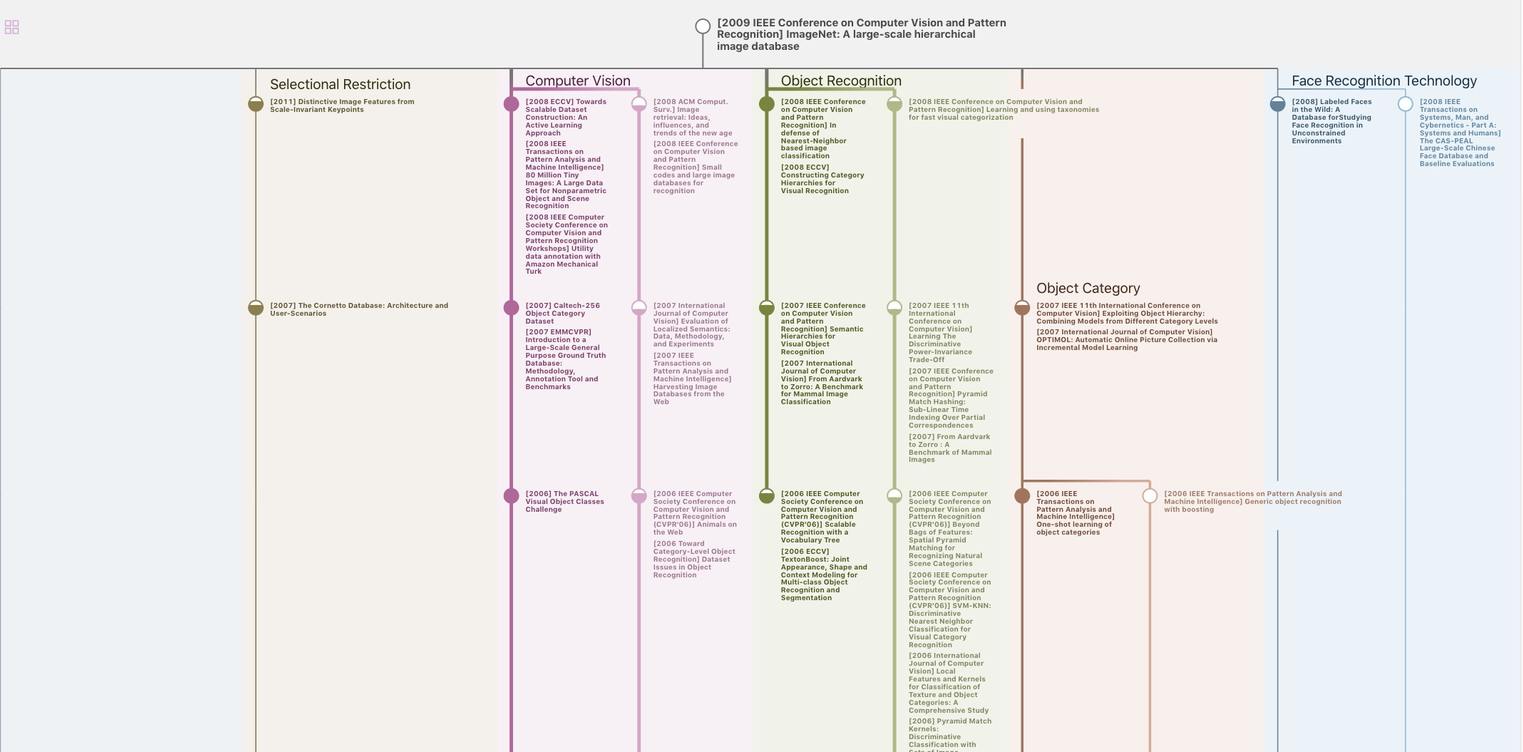
生成溯源树,研究论文发展脉络
Chat Paper
正在生成论文摘要