Robust Large-Margin Learning in Hyperbolic Space
NIPS 2020(2020)
摘要
Recently, there has been a surge of interest in representation learning in hyperbolic spaces, driven by their ability to represent hierarchical data with significantly fewer dimensions than standard Euclidean spaces. However, the viability and benefits of hyperbolic spaces for downstream machine learning tasks have received less attention. In this paper, we present, to our knowledge, the first theoretical guarantees for learning a classifier in hyperbolic rather than Euclidean space. Specifically, we consider the problem of learning a large-margin classifier for data possessing a hierarchical structure. Our first contribution is a hyperbolic perceptron algorithm, which provably converges to a separating hyperplane. We then provide an algorithm to efficiently learn a large-margin hyperplane, relying on the careful injection of adversarial examples. Finally, we prove that for hierarchical data that embeds well into hyperbolic space, the low embedding dimension ensures superior guarantees when learning the classifier directly in hyperbolic space.
更多查看译文
关键词
hyperbolic space,learning,large-margin
AI 理解论文
溯源树
样例
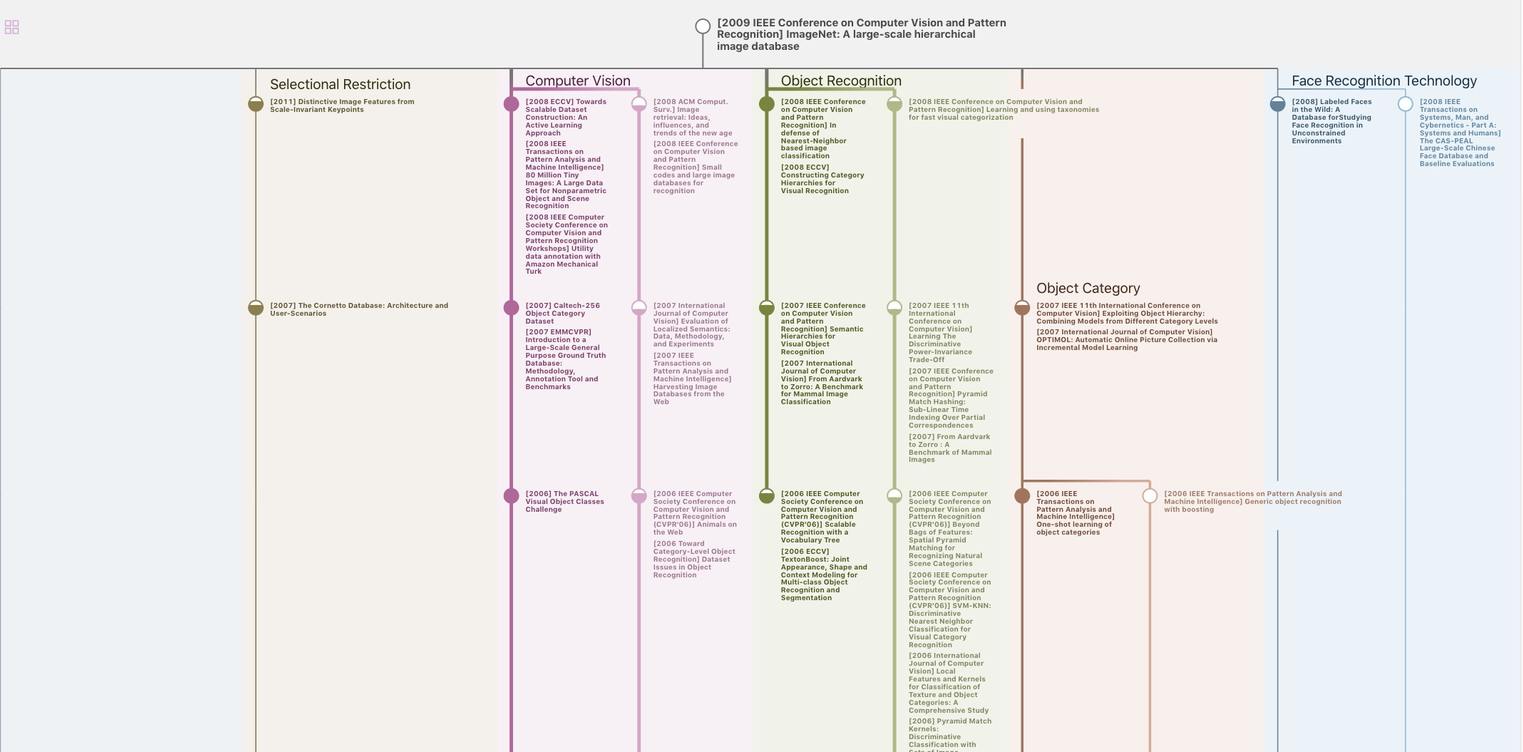
生成溯源树,研究论文发展脉络
Chat Paper
正在生成论文摘要