Estimating a Brain Network Predictive of Stress and Genotype with Supervised Autoencoders
arxiv(2023)
摘要
Targeted stimulation of the brain has the potential to treat mental illnesses. We propose an approach to help design the stimulation protocol by identifying electrical dynamics across many brain regions that relate to illness states. We model multi-region electrical activity as a superposition of activity from latent networks, where the weights on the latent networks relate to an outcome of interest. In order to improve on drawbacks of latent factor modeling in this context, we focus on supervised autoencoders (SAEs), which can improve predictive performance while maintaining a generative model. We explain why SAEs yield improved predictions, describe the distributional assumptions under which SAEs are an appropriate modeling choice, and provide modeling constraints to ensure biological relevance of the learned network. We use the analysis strategy to find a network associated with stress that characterizes a genotype associated with bipolar disorder. This discovered network aligns with a previously used stimulation technique, providing experimental validation of our approach.
更多查看译文
关键词
dimensionality reduction, factor analysis, joint model, neuroscience, supervised autoencoders
AI 理解论文
溯源树
样例
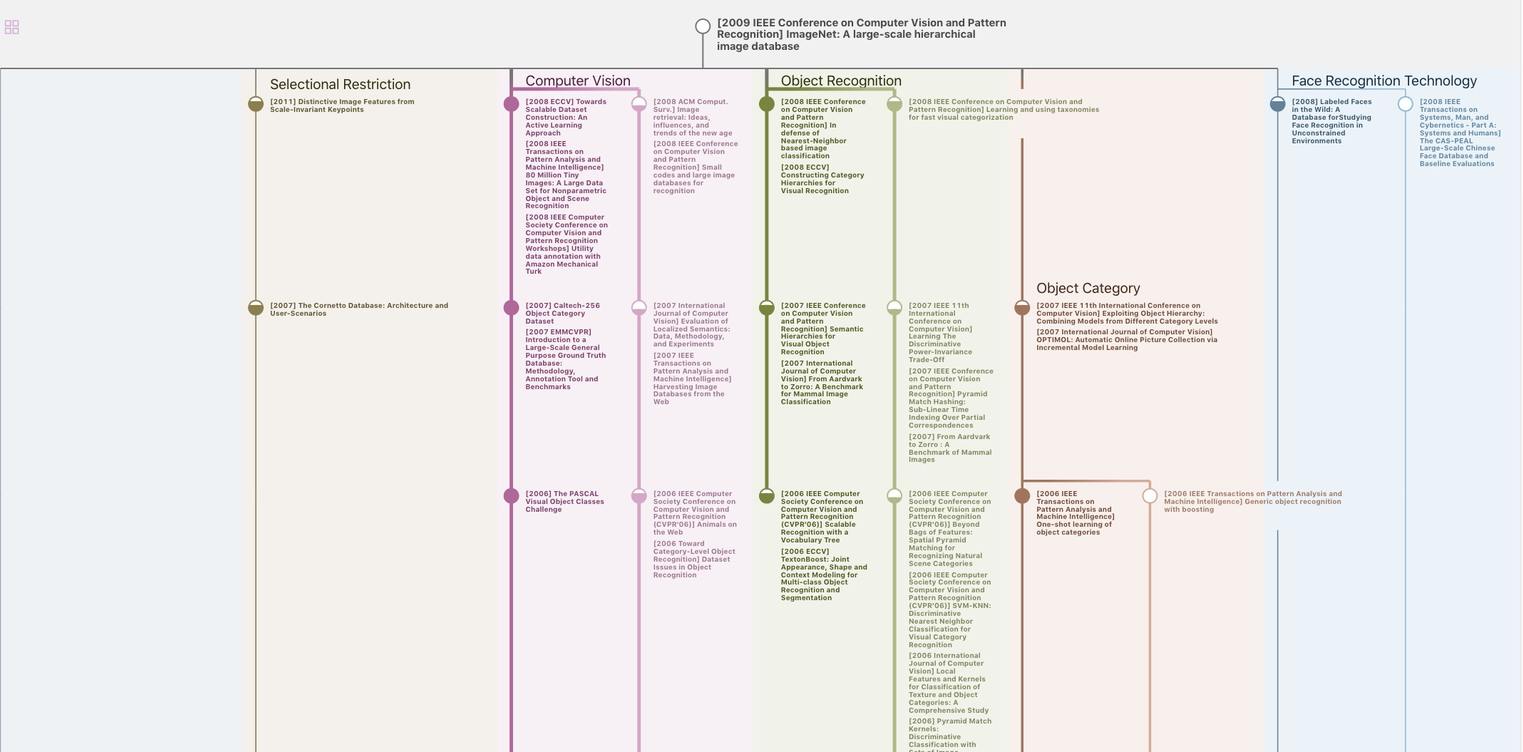
生成溯源树,研究论文发展脉络
Chat Paper
正在生成论文摘要