Towards Automatic Generation of Questions from Long Answers
arxiv(2020)
摘要
Automatic question generation (AQG) has broad applicability in domains such as tutoring systems, conversational agents, healthcare literacy, and information retrieval. Existing efforts at AQG have been limited to short answer lengths of up to two or three sentences. However, several real-world applications require question generation from answers that span several sentences. Therefore, we propose a novel evaluation benchmark to assess the performance of existing AQG systems for long-text answers. We leverage the large-scale open-source Google Natural Questions dataset to create the aforementioned long-answer AQG benchmark. We empirically demonstrate that the performance of existing AQG methods significantly degrades as the length of the answer increases. Transformer-based methods outperform other existing AQG methods on long answers in terms of automatic as well as human evaluation. However, we still observe degradation in the performance of our best performing models with increasing sentence length, suggesting that long answer QA is a challenging benchmark task for future research.
更多查看译文
关键词
automatic generation,questions
AI 理解论文
溯源树
样例
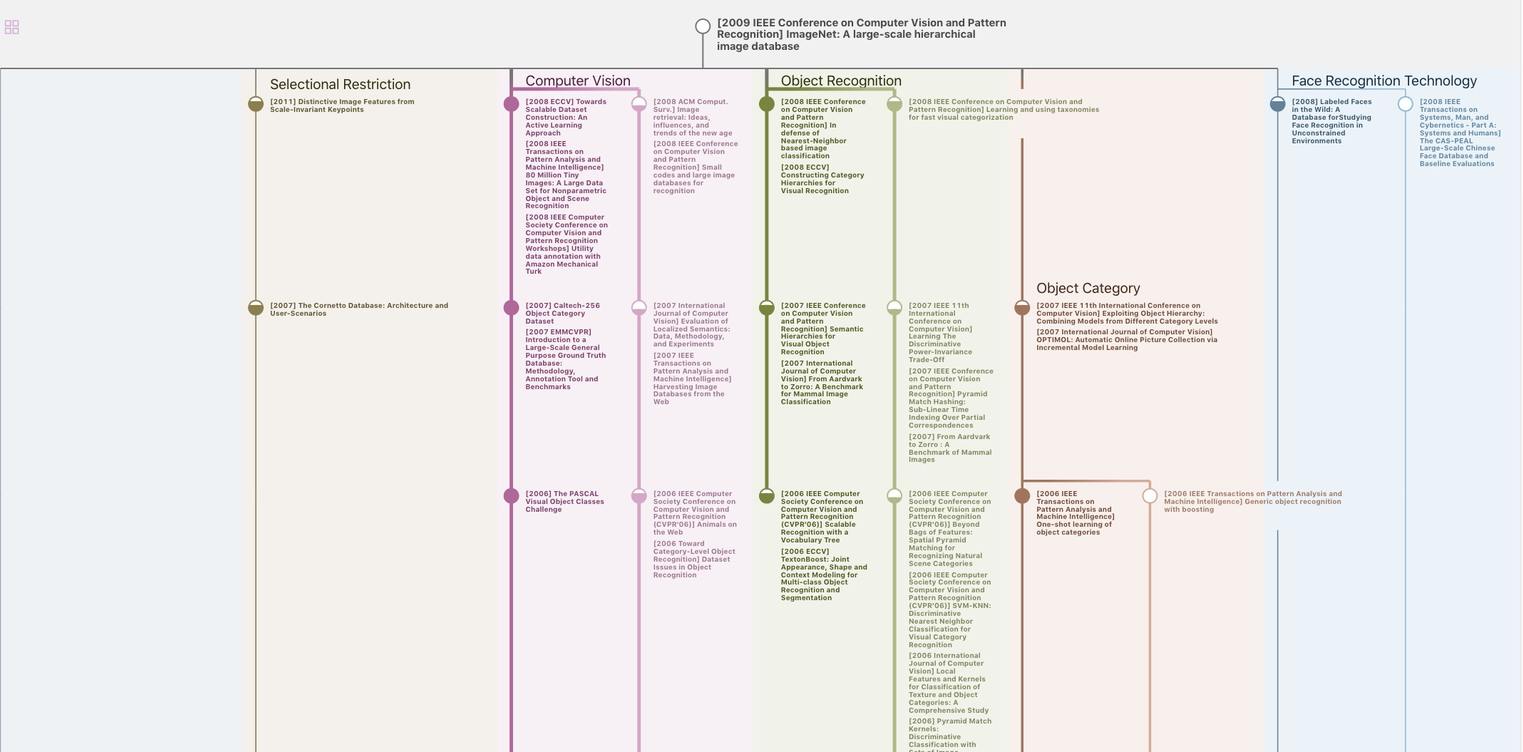
生成溯源树,研究论文发展脉络
Chat Paper
正在生成论文摘要