Multiresolution convolutional autoencoders
Journal of Computational Physics(2023)
摘要
We propose a multi-resolution convolutional autoencoder (MrCAE) architecture that integrates and leverages three highly successful mathematical architectures: (i) multigrid methods, (ii) convolutional autoencoders and (iii) transfer learning. The method provides an adaptive, hierarchical architecture that capitalizes on a progressive training approach for multiscale spatio-temporal data. This framework allows for inputs across multiple scales: starting from a compact (small number of weights) network architecture and low-resolution data, our network progressively deepens and widens itself in a principled manner to encode new information in the higher resolution data based on its current performance of reconstruction. Basic transfer learning techniques are applied to ensure information learned from previous training steps can be rapidly transferred to the larger network. As a result, the network can dynamically capture different scaled features at different depths of the network. The performance gains of this adaptive multiscale architecture are illustrated through a sequence of numerical experiments on synthetic examples and real -world spatial-temporal data.(c) 2022 Elsevier Inc. All rights reserved.
更多查看译文
关键词
Convolutional autoencoder,Multiresolution analysis,Multigrid,Transfer learning,Model scaling,Multi-scale dynamics
AI 理解论文
溯源树
样例
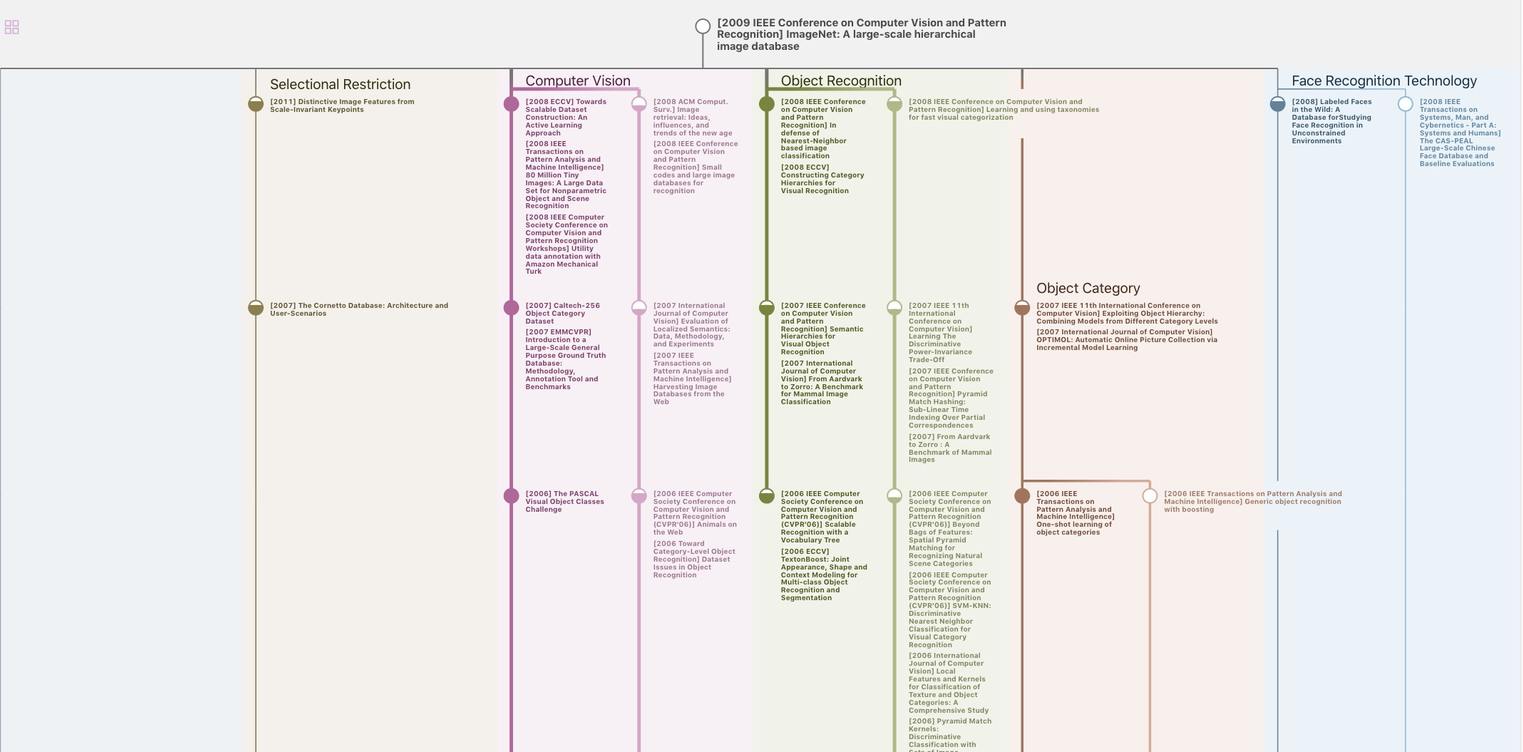
生成溯源树,研究论文发展脉络
Chat Paper
正在生成论文摘要