Sequentially Guided MCMC Proposals for Synthetic Likelihoods and Correlated Synthetic Likelihoods
BAYESIAN ANALYSIS(2023)
摘要
Synthetic likelihood (SL) is a strategy for parameter inference when the likelihood function is analytically or computationally intractable. In SL, the likelihood function of the data is replaced by a multivariate Gaussian density over summary statistics of the data. SL requires simulation of many replicate datasets at every parameter value considered by a sampling algorithm, such as Markov chain Monte Carlo (MCMC), making the method computationally-intensive. We propose two strategies to alleviate the computational burden. First, we introduce an algorithm producing a proposal distribution that is sequentially tuned and made conditional to data, thus it rapidly guides the proposed parameters towards high posterior density regions. In our experiments, a small number of iterations of our algorithm is enough to rapidly locate high density regions, which we use to initialize one or several chains that make use of off-the-shelf adaptive MCMC methods. Our "guided" approach can also be potentially used with MCMC sam-plers for approximate Bayesian computation (ABC). Second, we exploit strategies borrowed from the correlated pseudo-marginal MCMC literature, to improve the chains mixing in a SL framework. Moreover, our methods enable inference for challenging case studies, when the posterior is multimodal and when the chain is initialised in low posterior probability regions of the parameter space, where stan-dard samplers failed. To illustrate the advantages stemming from our framework we consider five benchmark examples, including estimation of parameters for a cosmological model and a stochastic model with highly non-Gaussian summary statistics.
更多查看译文
关键词
Bayesian inference,cosmological parameters,intractable likelihoods,likelihood-free
AI 理解论文
溯源树
样例
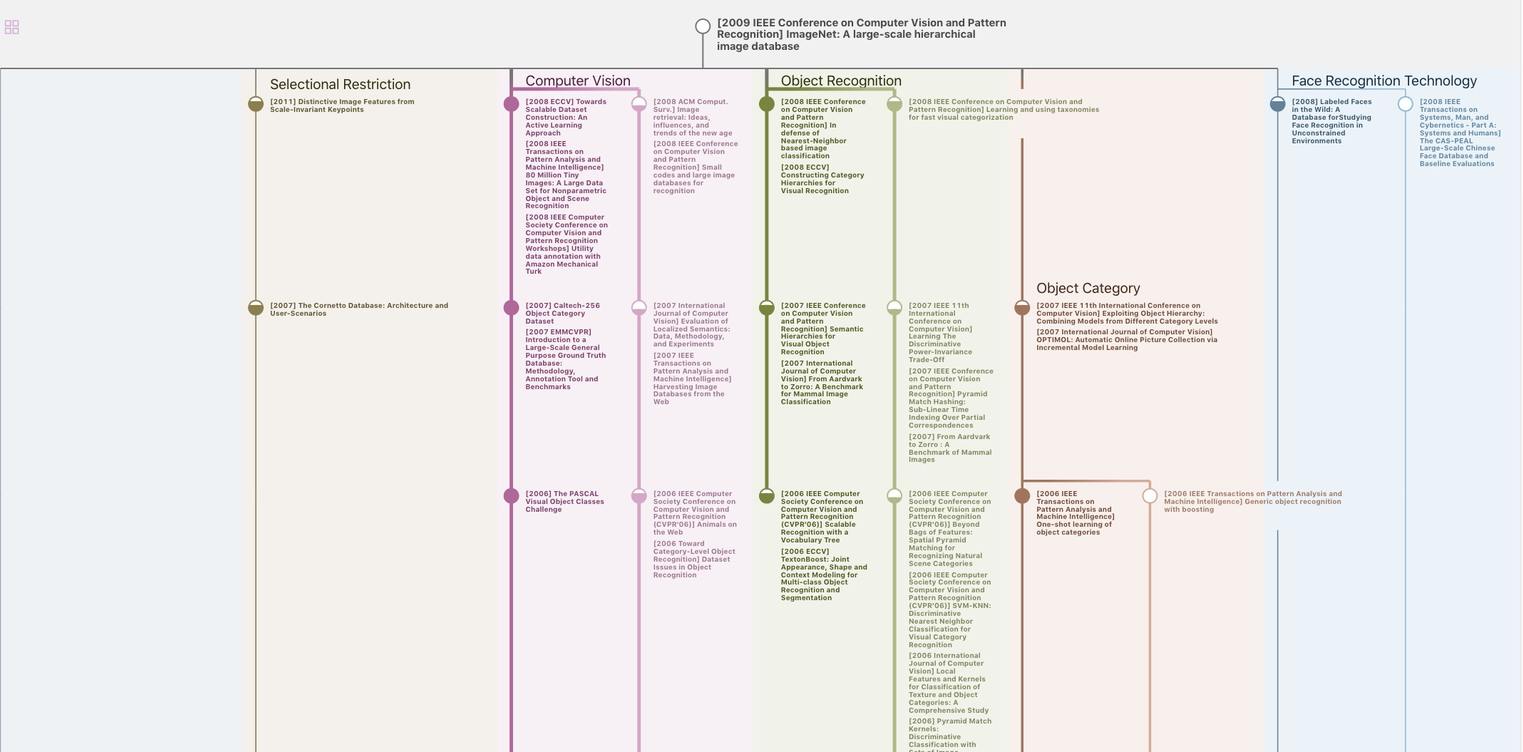
生成溯源树,研究论文发展脉络
Chat Paper
正在生成论文摘要