Spatial Information Guided Convolution For Real-Time Rgbd Semantic Segmentation
IEEE TRANSACTIONS ON IMAGE PROCESSING(2021)
摘要
3D spatial information is known to be beneficial to the semantic segmentation task. Most existing methods take 3D spatial data as an additional input, leading to a two-stream segmentation network that processes RGB and 3D spatial information separately. This solution greatly increases the inference time and severely limits its scope for real-time applications. To solve this problem, we propose Spatial information guided Convolution (S-Conv), which allows efficient RGB feature and 3D spatial information integration. S-Conv is competent to infer the sampling offset of the convolution kernel guided by the 3D spatial information, helping the convolutional layer adjust the receptive field and adapt to geometric transformations. S-Conv also incorporates geometric information into the feature learning process by generating spatially adaptive convolutional weights. The capability of perceiving geometry is largely enhanced without much affecting the amount of parameters and computational cost. Based on S-Conv, we further design a semantic segmentation network, called Spatial information Guided convolutional Network (SGNet), resulting in real-time inference and state-of-the-art performance on NYUDv2 and SUNRGBD datasets.
更多查看译文
关键词
Convolution, Three-dimensional displays, Semantics, Kernel, Geometry, Real-time systems, Image segmentation, Spatial information, receptive field, RGBD semantic segmentation
AI 理解论文
溯源树
样例
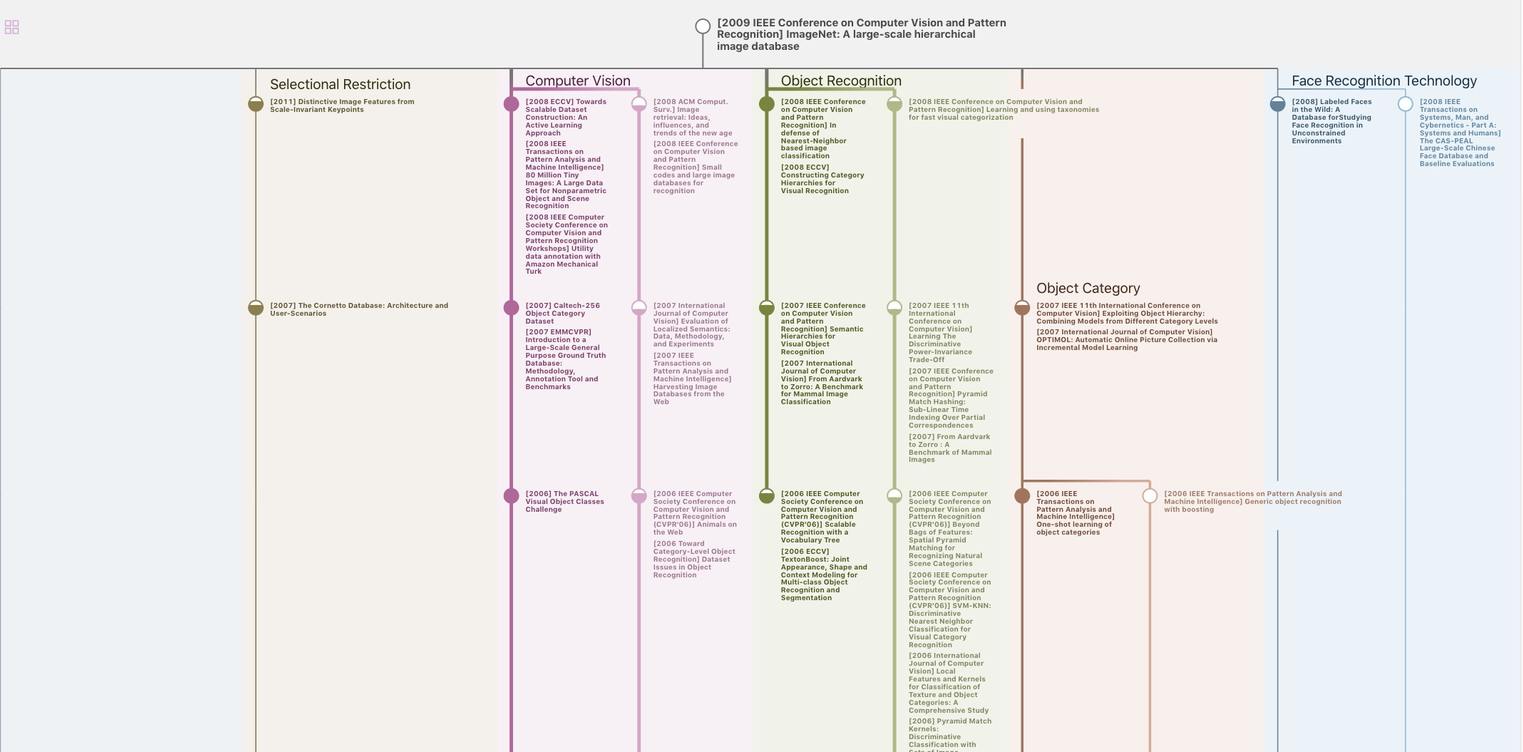
生成溯源树,研究论文发展脉络
Chat Paper
正在生成论文摘要