Prediction of mechanical properties of non-equiatomic high-entropy alloy by atomistic simulation and machine learning
arxiv(2020)
摘要
High-entropy alloys (HEAs) with multiple constituent elements have been extensively studied in the past 20 years due to their promising engineering application. Previous experimental and computational studies of HEAs focused mainly on equiatomic or near equiatomic HEAs. However, there is probably far more treasure in those non-equiatomic HEAs with carefully designed composition. In this study, molecular dynamics (MD) simulation combined with machine learning (ML) methods were used to predict the mechanical properties of non-equiatomic CuFeNiCrCo HEAs. A database was established based on a tensile test of 900 HEA single-crystal samples by MD simulation. We investigated and compared eight ML models for the learning tasks, ranging from shallow models to deep models. It was found that the kernel-based extreme learning machine (KELM) model outperformed others for the prediction of yield stress and Young's modulus. The accuracy of the KELM model was further verified by the large-sized polycrystal HEA samples.
更多查看译文
关键词
molecular dynamics, high-entropy alloy, machine learning, mechanical property
AI 理解论文
溯源树
样例
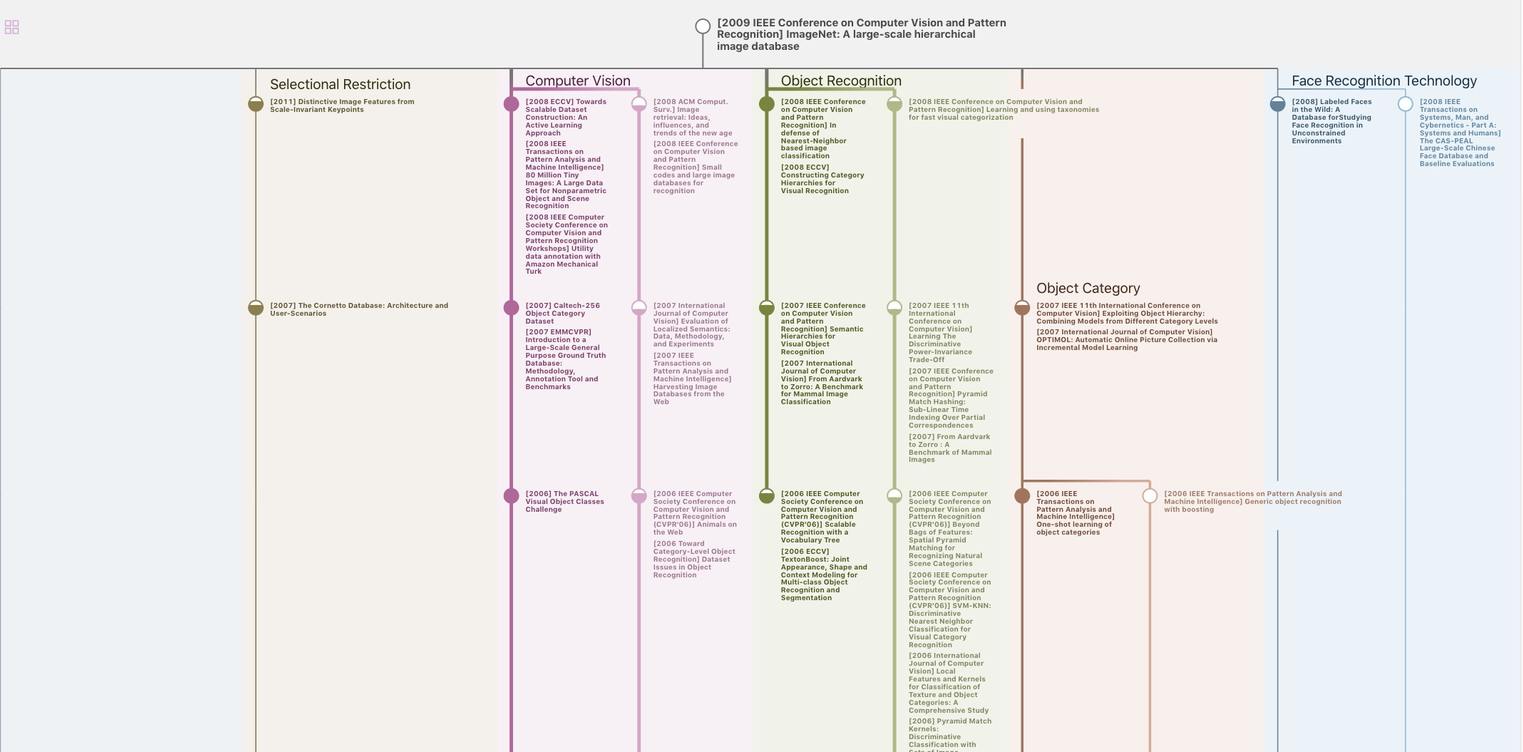
生成溯源树,研究论文发展脉络
Chat Paper
正在生成论文摘要