Incremental Evolution and Development of Deep Artificial Neural Networks.
EuroGP(2020)
摘要
NeuroEvolution (NE) methods are known for applying Evolutionary Computation to the optimisation of Artificial Neural Networks(ANNs). Despite aiding non-expert users to design and train ANNs, the vast majority of NE approaches disregard the knowledge that is gathered when solving other tasks, i.e., evolution starts from scratch for each problem, ultimately delaying the evolutionary process. To overcome this drawback, we extend Fast Deep Evolutionary Network Structured Representation (Fast-DENSER) to incremental development. We hypothesise that by transferring the knowledge gained from previous tasks we can attain superior results and speedup evolution. The results show that the average performance of the models generated by incremental development is statistically superior to the non-incremental average performance. In case the number of evaluations performed by incremental development is smaller than the performed by non-incremental development the attained results are similar in performance, which indicates that incremental development speeds up evolution. Lastly, the models generated using incremental development generalise better, and thus, without further evolution, report a superior performance on unseen problems.
更多查看译文
关键词
deep artificial neural networks,artificial neural networks,neural networks,incremental,evolution
AI 理解论文
溯源树
样例
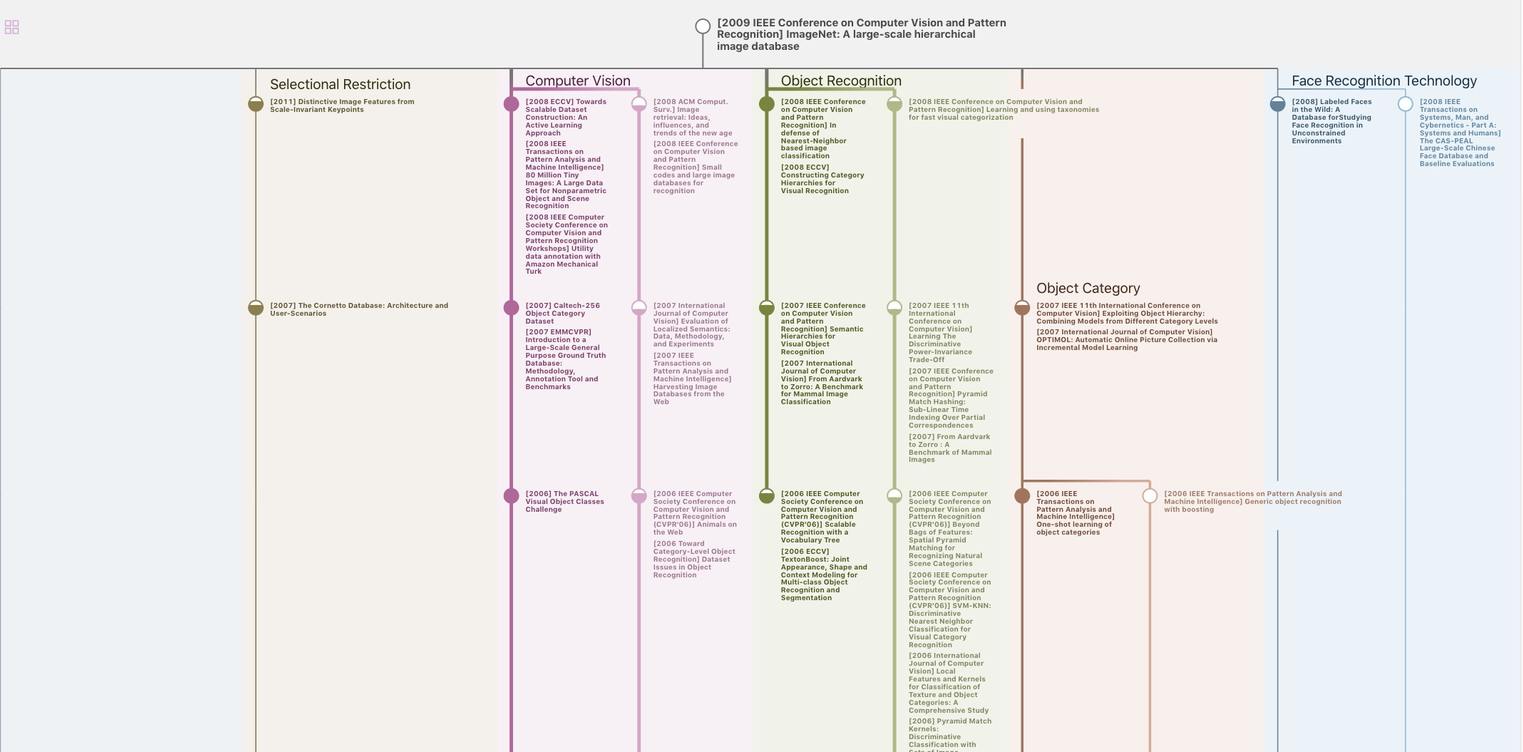
生成溯源树,研究论文发展脉络
Chat Paper
正在生成论文摘要