Detection Of Abnormalities In Electrocardiogram (Ecg) Using Deep Learning
PROCEEDINGS OF THE 13TH INTERNATIONAL JOINT CONFERENCE ON BIOMEDICAL ENGINEERING SYSTEMS AND TECHNOLOGIES, VOL 4: BIOSIGNALS(2020)
摘要
The Electrocardiogram (ECG) cyclic behaviour gives insights on a subject's emotional, behavioral and cardiovascular state, but often presents abnormal events. The noise made during the acquisition, and presence of symptomatic patterns are examples of anomalies. The proposed Deep Learning framework learns the normal ECG cycles and detects its deviation when the morphology changes. This technology is tested in two different settings having an autoencoder as base for learning features: detection of three different types of noise, and detection of six arrhythmia events. Two Convolutional Neural Network (CNN) algorithms were developed for noise detection achieving accuracies of 98.18% for a binary-class model and 70.74% for a multi-class model. The development of the arrhythmia detection algorithm also included a Gated Recurrent Unit (GRU) for grasping time-dependencies reaching an accuracy of 56.85% and an average sensitivity of 61.13%. The process of learning the abstraction of a ECG signal, currently sacrifices the accuracy for higher generalization, better discriminating the presence of abnormal events in ECG than detecting different types of events. Further improvement could represent a major contribution in symptomatic screening. active learning of unseen events and the study of pathologies to support physicians in the future.
更多查看译文
关键词
Electrocardiogram,Signal Processing,Deep Learning,Artificial Intelligence,Arrhythmia Detection,Noise Detection
AI 理解论文
溯源树
样例
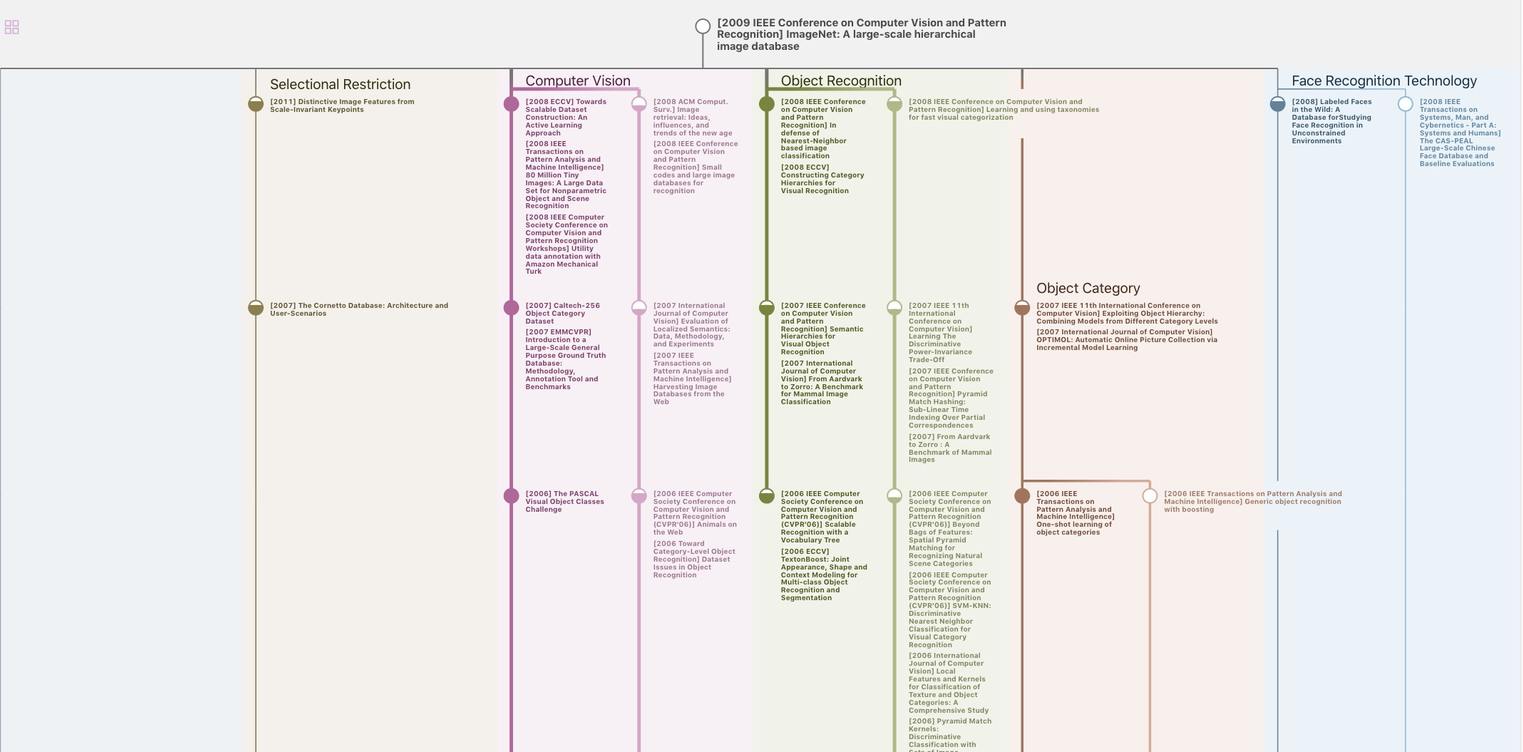
生成溯源树,研究论文发展脉络
Chat Paper
正在生成论文摘要