Top-down machine learning approach for high-throughput single-molecule analysis.
ELIFE(2020)
摘要
Single-molecule approaches provide enormous insight into the dynamics of biomolecules, but adequately sampling distributions of states and events often requires extensive sampling. Although emerging experimental techniques can generate such large datasets, existing analysis tools are not suitable to process the large volume of data obtained in high-throughput paradigms. Here, we present a new analysis platform (DISC) that accelerates unsupervised analysis of single-molecule trajectories. By merging model-free statistical learning with the Viterbi algorithm, DISC idealizes single-molecule trajectories up to three orders of magnitude faster with improved accuracy compared to other commonly used algorithms. Further, we demonstrate the utility of DISC algorithm to probe cooperativity between multiple binding events in the cyclic nucleotide binding domains of HCN pacemaker channel. Given the flexible and efficient nature of DISC, we anticipate it will be a powerful tool for unsupervised processing of high-throughput data across a range of single-molecule experiments.
更多查看译文
关键词
human,molecular biophysics,structural biology
AI 理解论文
溯源树
样例
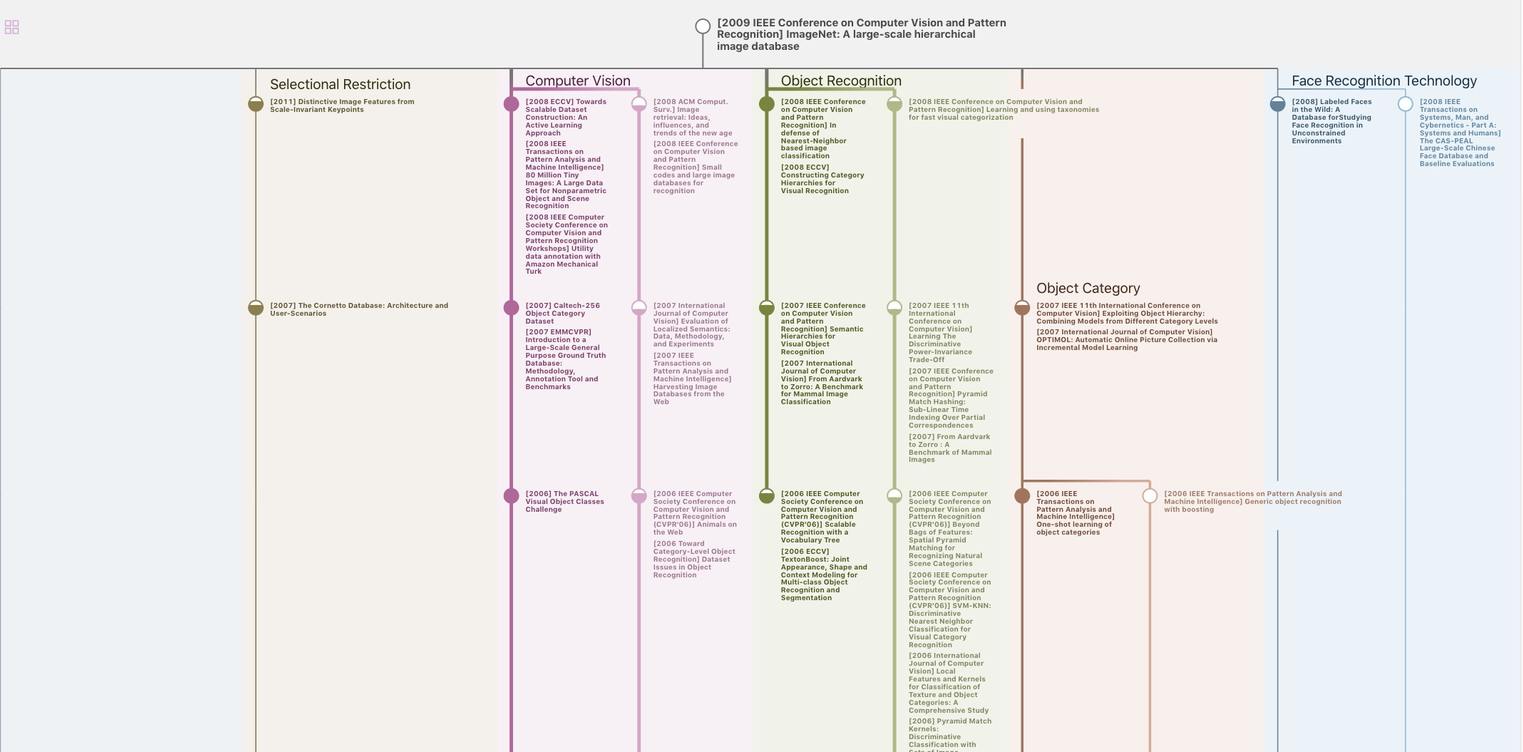
生成溯源树,研究论文发展脉络
Chat Paper
正在生成论文摘要