Self-Supervised Monocular Scene Flow Estimation
CVPR(2020)
摘要
Scene flow estimation has been receiving increasing attention for 3D environment perception. Monocular scene flow estimation -- obtaining 3D structure and 3D motion from two temporally consecutive images -- is a highly ill-posed problem, and practical solutions are lacking to date. We propose a novel monocular scene flow method that yields competitive accuracy and real-time performance. By taking an inverse problem view, we design a single convolutional neural network (CNN) that successfully estimates depth and 3D motion simultaneously from a classical optical flow cost volume. We adopt self-supervised learning with 3D loss functions and occlusion reasoning to leverage unlabeled data. We validate our design choices, including the proxy loss and augmentation setup. Our model achieves state-of-the-art accuracy among unsupervised/self-supervised learning approaches to monocular scene flow, and yields competitive results for the optical flow and monocular depth estimation sub-tasks. Semi-supervised fine-tuning further improves the accuracy and yields promising results in real-time.
更多查看译文
关键词
flow,self-supervised
AI 理解论文
溯源树
样例
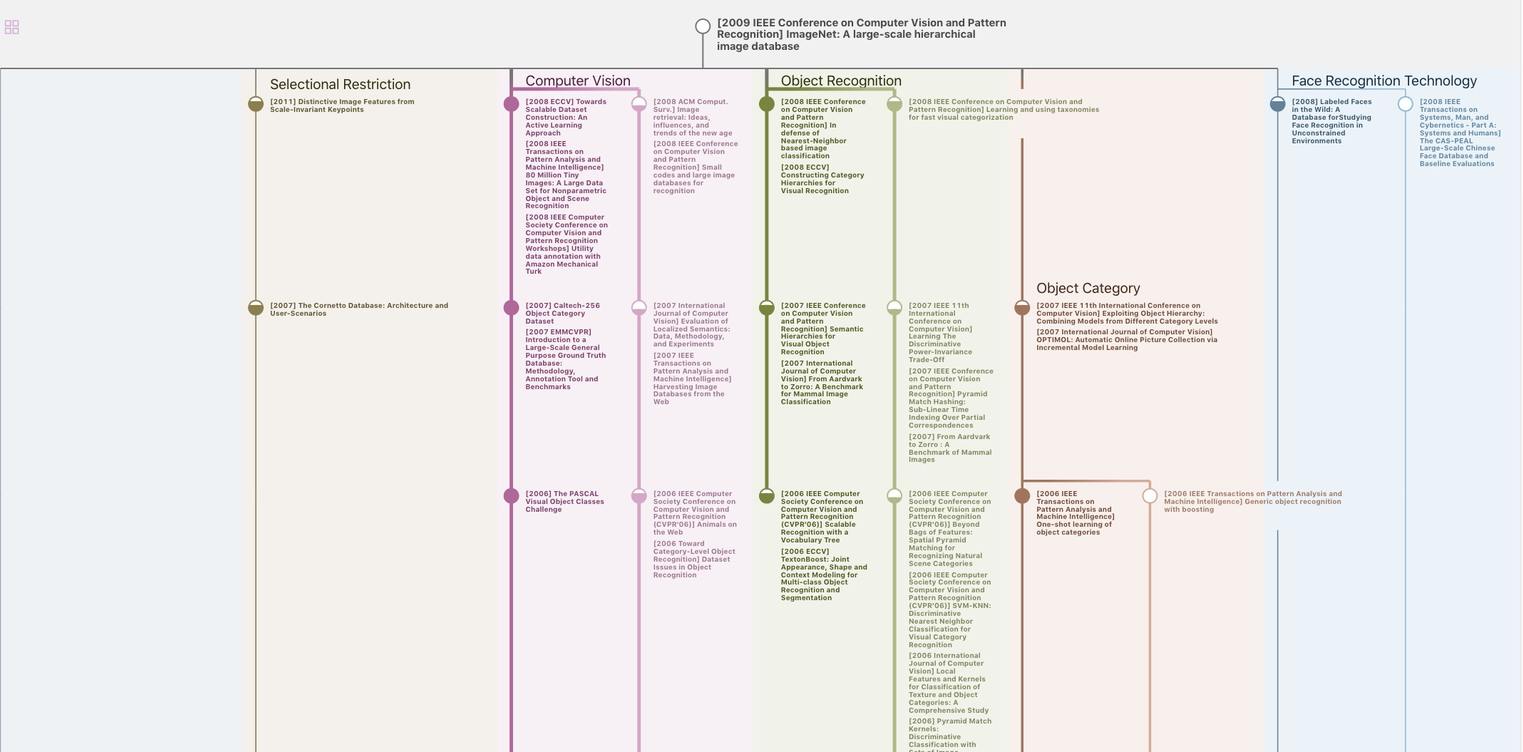
生成溯源树,研究论文发展脉络
Chat Paper
正在生成论文摘要