Salience Estimation with Multi-Attention Learning for Abstractive Text Summarization
arxiv(2020)
摘要
Attention mechanism plays a dominant role in the sequence generation models and has been used to improve the performance of machine translation and abstractive text summarization. Different from neural machine translation, in the task of text summarization, salience estimation for words, phrases or sentences is a critical component, since the output summary is a distillation of the input text. Although the typical attention mechanism can conduct text fragment selection from the input text conditioned on the decoder states, there is still a gap to conduct direct and effective salience detection. To bring back direct salience estimation for summarization with neural networks, we propose a Multi-Attention Learning framework which contains two new attention learning components for salience estimation: supervised attention learning and unsupervised attention learning. We regard the attention weights as the salience information, which means that the semantic units with large attention value will be more important. The context information obtained based on the estimated salience is incorporated with the typical attention mechanism in the decoder to conduct summary generation. Extensive experiments on some benchmark datasets in different languages demonstrate the effectiveness of the proposed framework for the task of abstractive summarization.
更多查看译文
AI 理解论文
溯源树
样例
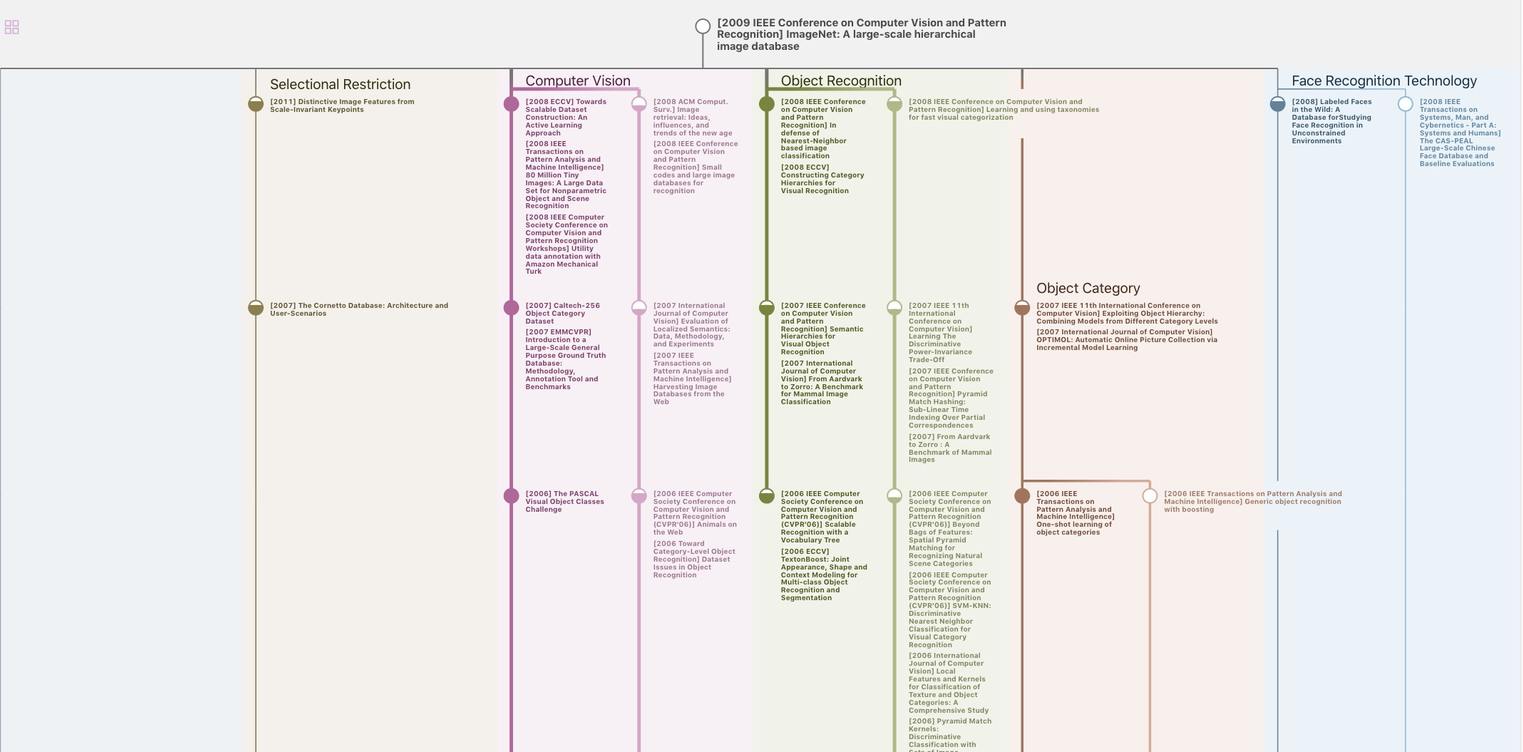
生成溯源树,研究论文发展脉络
Chat Paper
正在生成论文摘要