Robust Self-Supervised Convolutional Neural Network for Subspace Clustering and Classification
arXiv (Cornell University)(2020)
摘要
Insufficient capability of existing subspace clustering methods to handle data coming from nonlinear manifolds, data corruptions, and out-of-sample data hinders their applicability to address real-world clustering and classification problems. This paper proposes the robust formulation of the self-supervised convolutional subspace clustering network ($S^2$ConvSCN) that incorporates the fully connected (FC) layer and, thus, it is capable for handling out-of-sample data by classifying them using a softmax classifier. $S^2$ConvSCN clusters data coming from nonlinear manifolds by learning the linear self-representation model in the feature space. Robustness to data corruptions is achieved by using the correntropy induced metric (CIM) of the error. Furthermore, the block-diagonal (BD) structure of the representation matrix is enforced explicitly through BD regularization. In a truly unsupervised training environment, Robust $S^2$ConvSCN outperforms its baseline version by a significant amount for both seen and unseen data on four well-known datasets. Arguably, such an ablation study has not been reported before.
更多查看译文
关键词
subspace clustering,classification,convolutional neural network,self-supervised
AI 理解论文
溯源树
样例
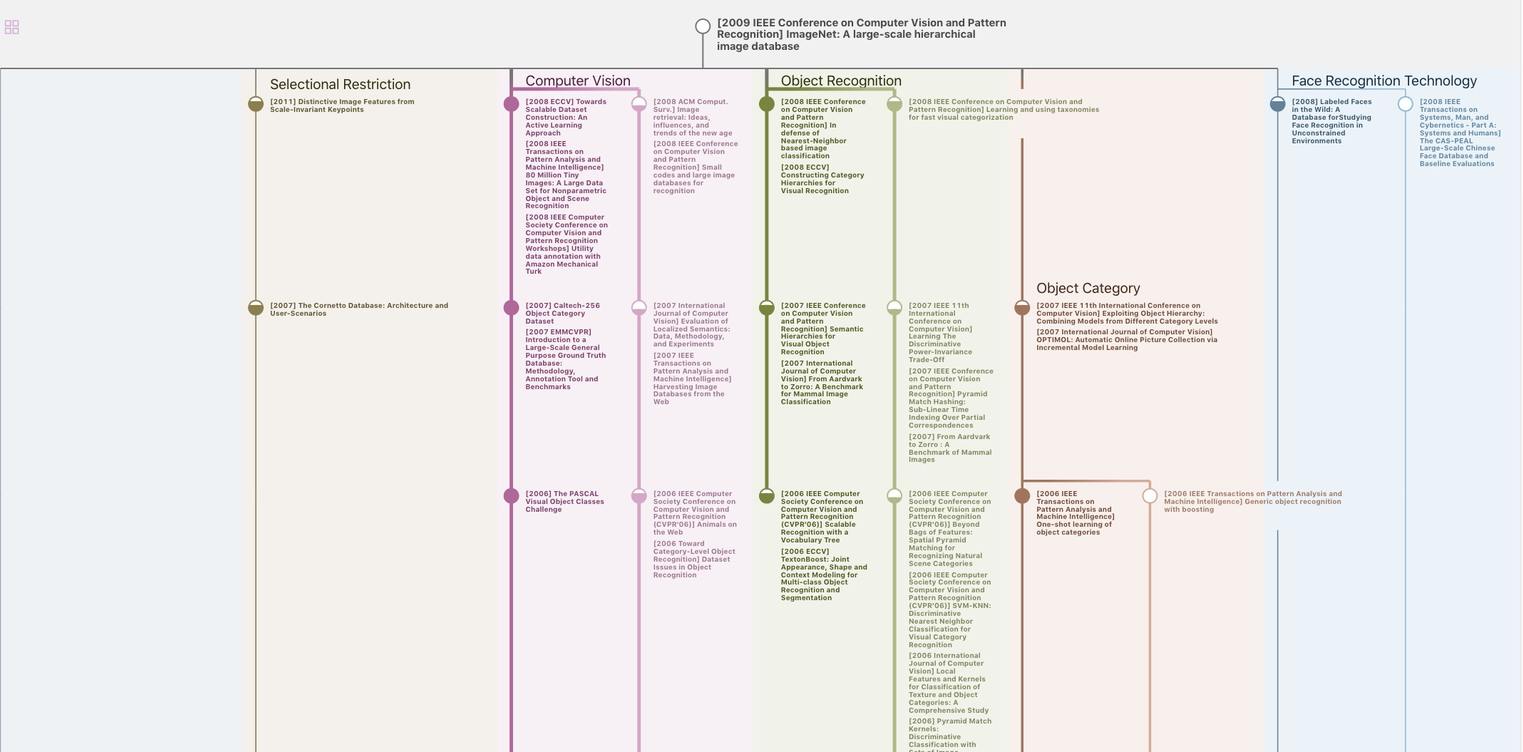
生成溯源树,研究论文发展脉络
Chat Paper
正在生成论文摘要