Self-Adjusting Evolutionary Algorithms for Multimodal Optimization
Genetic and Evolutionary Computation Conference(2022)
摘要
Recent theoretical research has shown that self-adjusting and self-adaptive mechanisms can provably outperform static settings in evolutionary algorithms for binary search spaces. However, the vast majority of these studies focuses on unimodal functions which do not require the algorithm to flip several bits simultaneously to make progress. In fact, existing self-adjusting algorithms are not designed to detect local optima and do not have any obvious benefit to cross large Hamming gaps. We suggest a mechanism called stagnation detection that can be added as a module to existing evolutionary algorithms (both with and without prior self-adjusting schemes). Added to a simple (1+1) EA, we prove an expected runtime on the well-known Jump benchmark that corresponds to an asymptotically optimal parameter setting and outperforms other mechanisms for multimodal optimization like heavy-tailed mutation. We also investigate the module in the context of a self-adjusting (1+ λ ) EA. To explore the limitations of the approach, we additionally present an example where both self-adjusting mechanisms, including stagnation detection, do not help to find a beneficial setting of the mutation rate. Finally, we investigate our module for stagnation detection experimentally.
更多查看译文
关键词
Randomized search heuristics,Self-adjusting algorithms,Multimodal functions,Runtime analysis
AI 理解论文
溯源树
样例
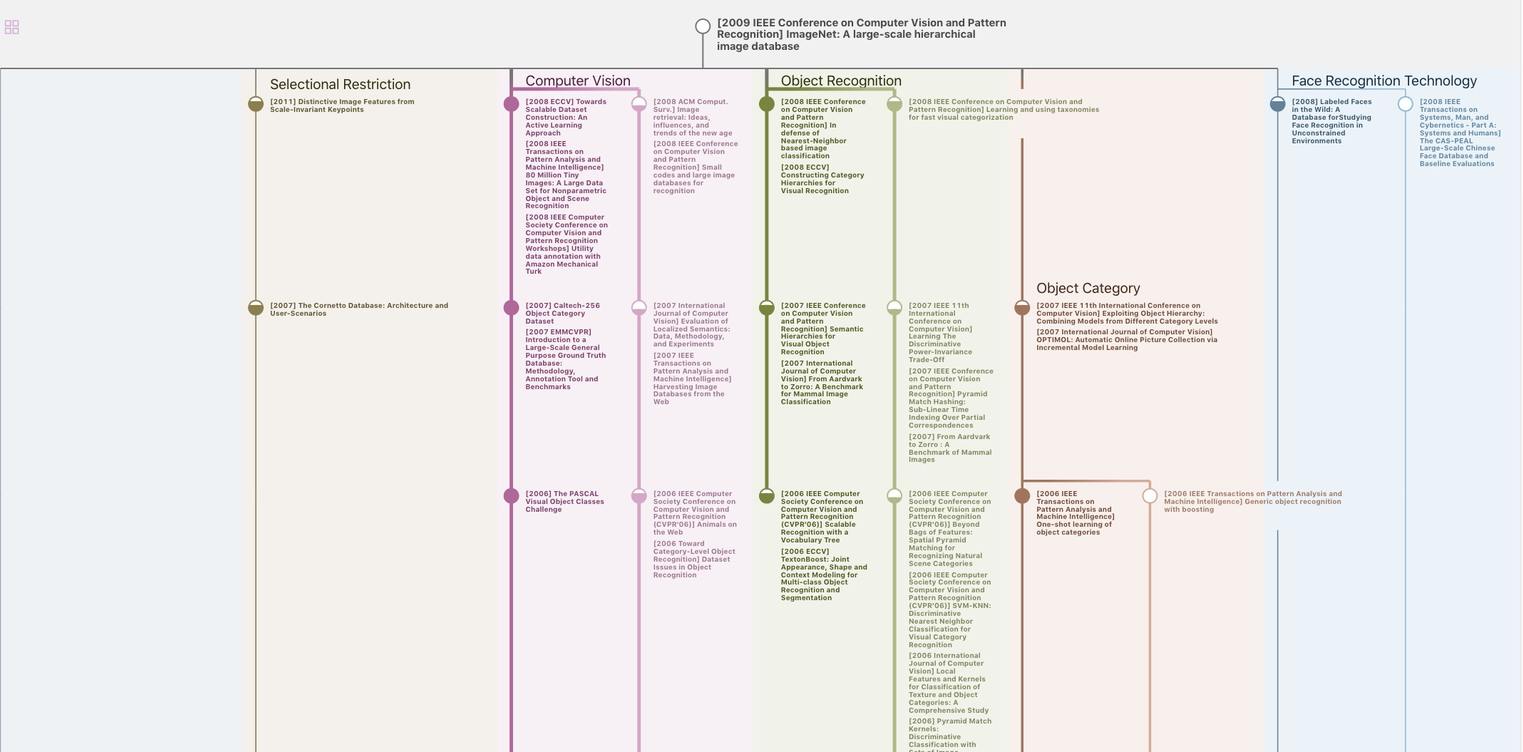
生成溯源树,研究论文发展脉络
Chat Paper
正在生成论文摘要