Multi-Task Learning Via Co-Attentive Sharing For Pedestrian Attribute Recognition
2020 IEEE International Conference on Multimedia and Expo (ICME)(2020)
摘要
Learning to predict multiple attributes of a pedestrian is a multi-task learning problem. To share feature representation between two individual task networks, conventional methods like Cross-Stitch [1] and Sluice [2] network learn a linear combination of features or feature subspaces. However, linear combination rules out the complex interdependency between channels. Moreover, spatial information exchanging is less-considered. In this paper, we propose a novel Co-Attentive Sharing (CAS) module which extracts discriminative channels and spatial regions for more effective feature sharing in multi-task learning. The module consists of three branches, which leverage different channels for between-task feature fusing, attention generation and task-specific feature enhancing, respectively. Experiments on two pedestrian attribute recognition datasets show that our module outperforms the conventional sharing units and achieves superior results compared to the state-of-the-art approaches using many metrics.
更多查看译文
关键词
pedestrian attribute recognition,multitask learning,feature fusing
AI 理解论文
溯源树
样例
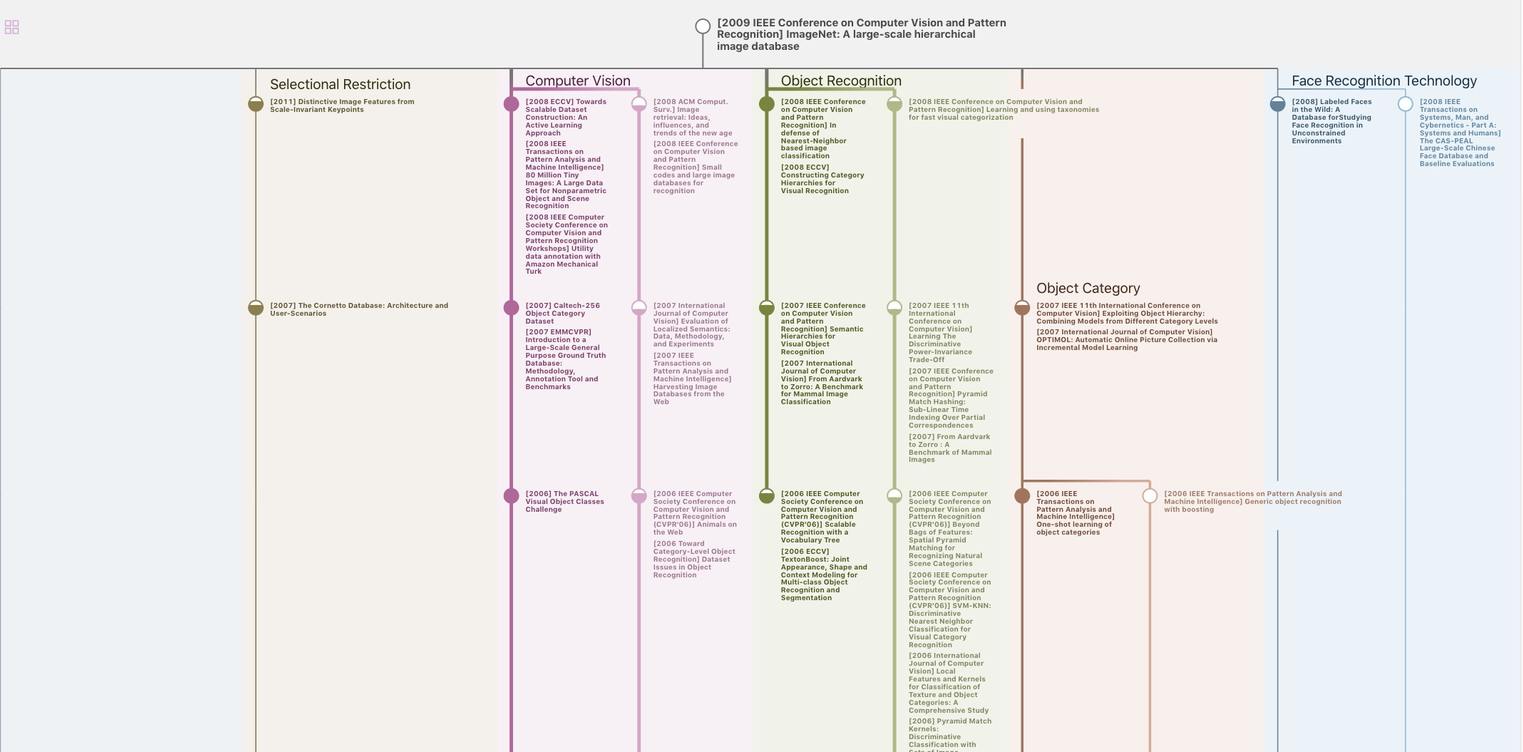
生成溯源树,研究论文发展脉络
Chat Paper
正在生成论文摘要