Guiding Monocular Depth Estimation Using Depth-Attention Volume
European Conference on Computer Vision(2020)
摘要
Recovering the scene depth from a single image is an ill-posed problem that requires additional priors, often referred to as monocular depth cues, to disambiguate different 3D interpretations. In recent works, those priors have been learned in an end-to-end manner from large datasets by using deep neural networks. In this paper, we propose guiding depth estimation to favor planar structures that are ubiquitous especially in indoor environments. This is achieved by incorporating a non-local coplanarity constraint to the network with a novel attention mechanism called depth-attention volume (DAV). Experiments on two popular indoor datasets, namely NYU-Depth-v2 and ScanNet, show that our method achieves state-of-the-art depth estimation results while using only a fraction of the number of parameters needed by the competing methods. Code is available at: https://github.com/HuynhLam/DAV.
更多查看译文
关键词
monocular depth estimation,depth-attention
AI 理解论文
溯源树
样例
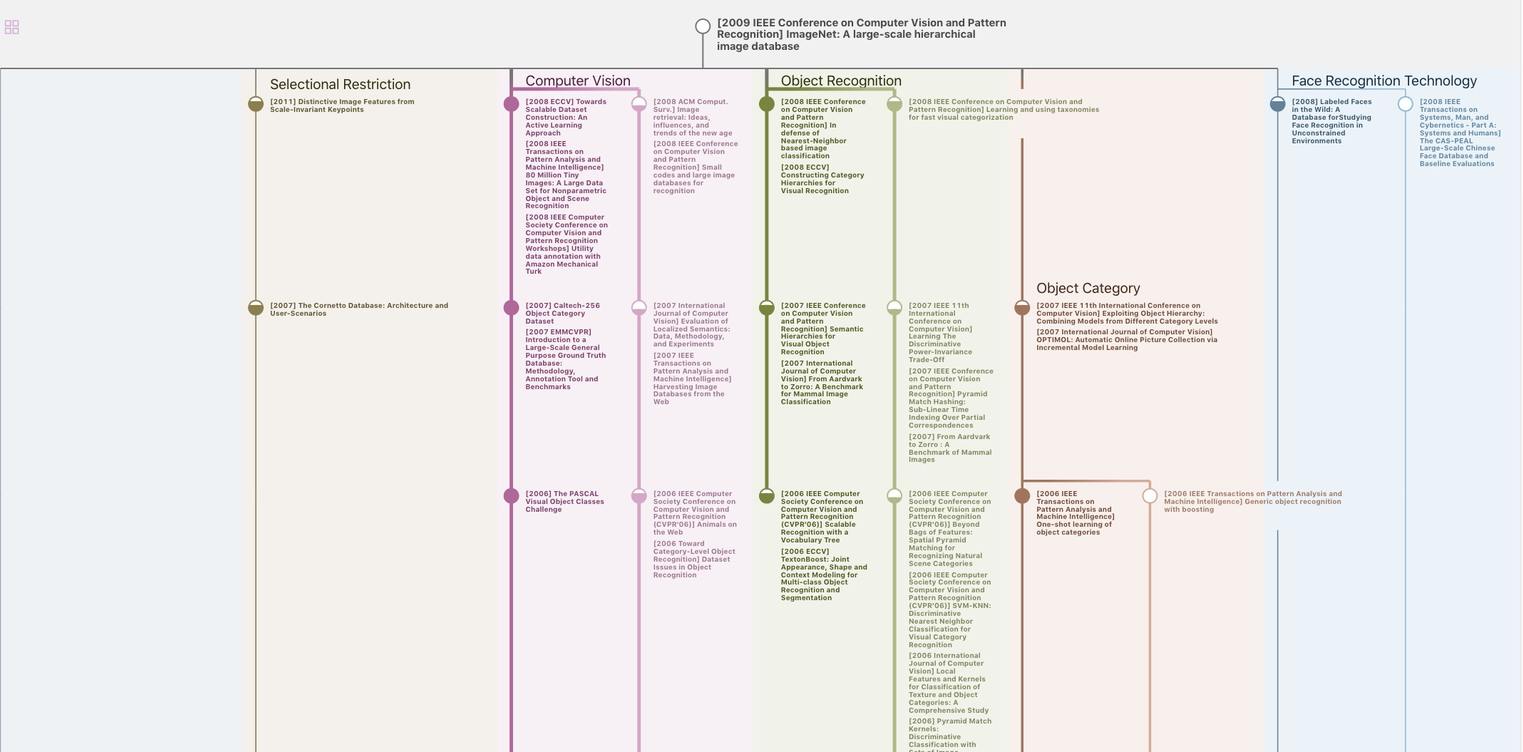
生成溯源树,研究论文发展脉络
Chat Paper
正在生成论文摘要