Cascaded Deep Video Deblurring Using Temporal Sharpness Prior
2020 IEEE/CVF CONFERENCE ON COMPUTER VISION AND PATTERN RECOGNITION (CVPR)(2020)
摘要
We present a simple and effective deep convolutional neural network (CNN) model for video deblurring. The proposed algorithm mainly consists of optical flow estimation from intermediate latent frames and latent frame restoration steps. It first develops a deep CNN model to estimate optical flow from intermediate latent frames and then restores the latent frames based on the estimated optical flow To better explore the temporal information from videos, we develop a temporal sharpness prior to constrain the deep CNN model to help the latent frame restoration. We develop an effective cascaded training approach and jointly train the proposed CNN model in an end-to-end manner. We show that exploring the domain knowledge of video deblurring is able to make the deep CNN model more compact and efficient. Extensive experimental results show that the proposed algorithm performs favorably against state-of-the-art methods on the benchmark datasets as well as real-world videos.
更多查看译文
关键词
deep video deblurring,temporal sharpness prior
AI 理解论文
溯源树
样例
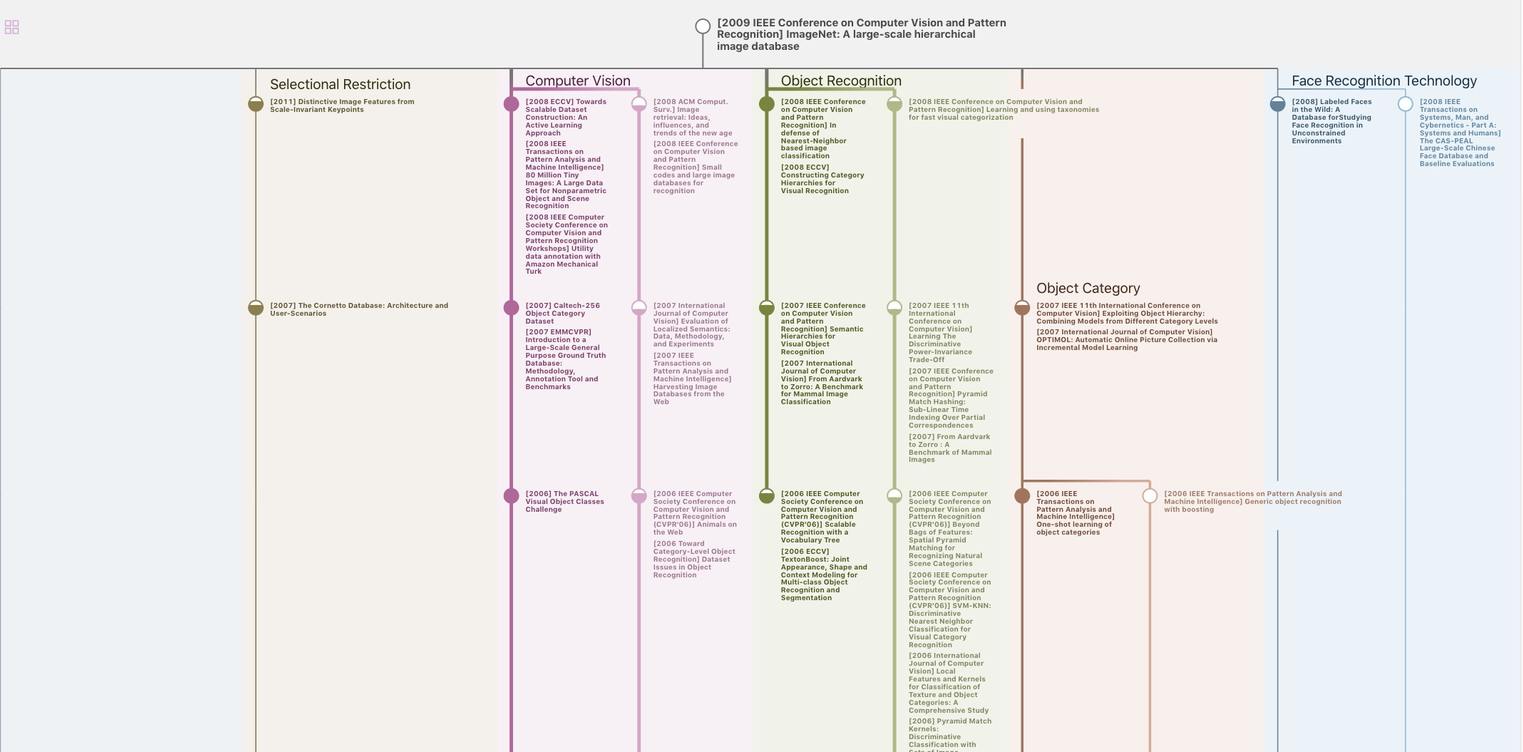
生成溯源树,研究论文发展脉络
Chat Paper
正在生成论文摘要