A Discriminator Improves Unconditional Text Generation without Updating the Generator
arxiv(2020)
摘要
We propose a novel mechanism to improve a text generator with a discriminator, which is trained to estimate the probability that a sample comes from real or generated data. In contrast to recent discrete language generative adversarial networks (GAN) which update the parameters of the generator directly, our method only retains generated samples which are determined to come from real data with relatively high probability by the discriminator. This not only detects valuable information, but also avoids the mode collapse introduced by GAN.This new mechanism is conceptually simple and experimentally powerful. To the best of our knowledge, this is the first method which improves the neural language models (LM) trained with maximum likelihood estimation (MLE) by using a discriminator. Experimental results show that our mechanism improves both RNN-based and Transformer-based LMs when measuring in sample quality and sample diversity simultaneously at different softmax temperatures (a previously noted deficit of language GANs). Further, by recursively adding more discriminators, more powerful generators are created.
更多查看译文
关键词
unconditional text generation,discriminator
AI 理解论文
溯源树
样例
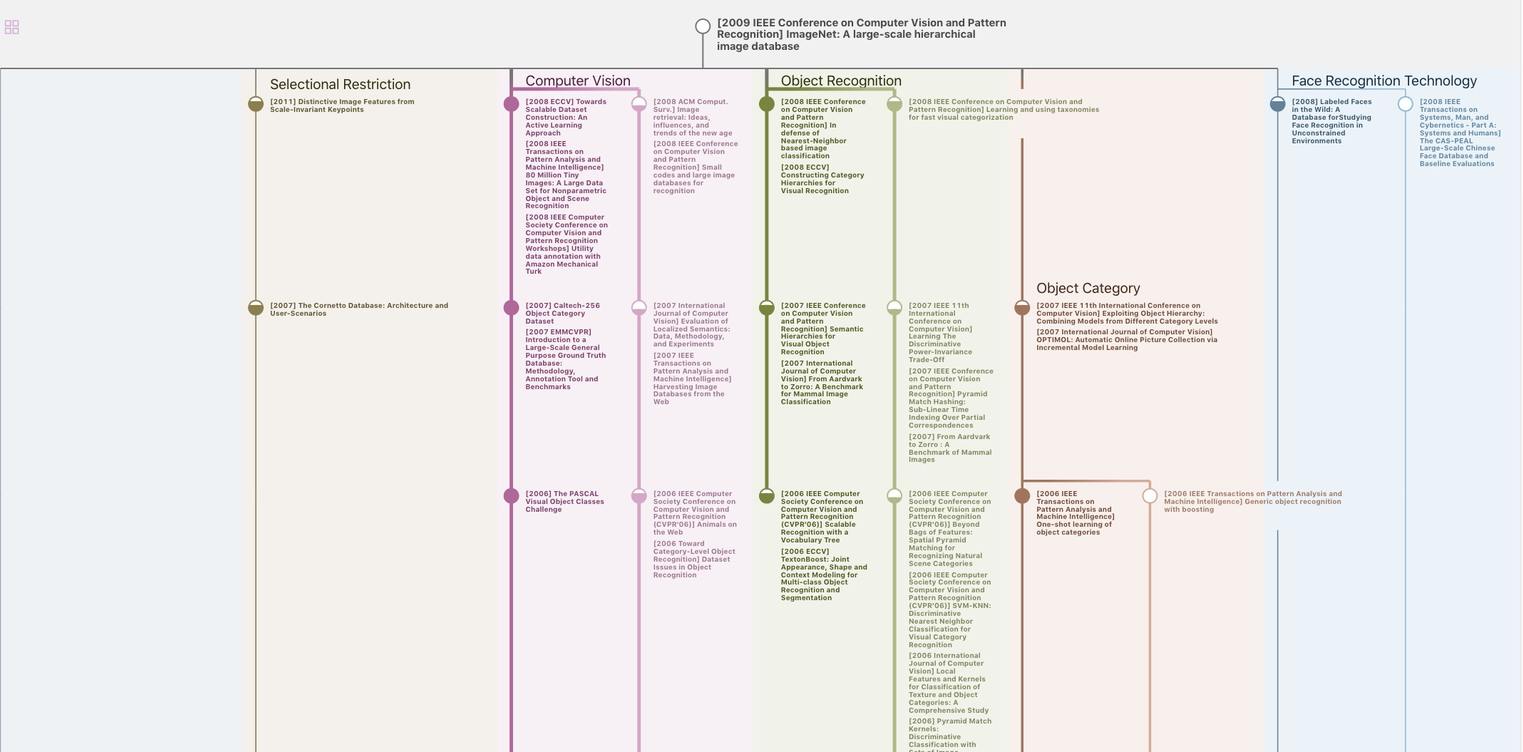
生成溯源树,研究论文发展脉络
Chat Paper
正在生成论文摘要