News-Driven Stock Prediction With Attention-Based Noisy Recurrent State Transition
arxiv(2020)
摘要
We consider direct modeling of underlying stock value movement sequences over time in the news-driven stock movement prediction. A recurrent state transition model is constructed, which better captures a gradual process of stock movement continuously by modeling the correlation between past and future price movements. By separating the effects of news and noise, a noisy random factor is also explicitly fitted based on the recurrent states. Results show that the proposed model outperforms strong baselines. Thanks to the use of attention over news events, our model is also more explainable. To our knowledge, we are the first to explicitly model both events and noise over a fundamental stock value state for news-driven stock movement prediction.
更多查看译文
关键词
stock prediction,noisy recurrent state transition,news-driven,attention-based
AI 理解论文
溯源树
样例
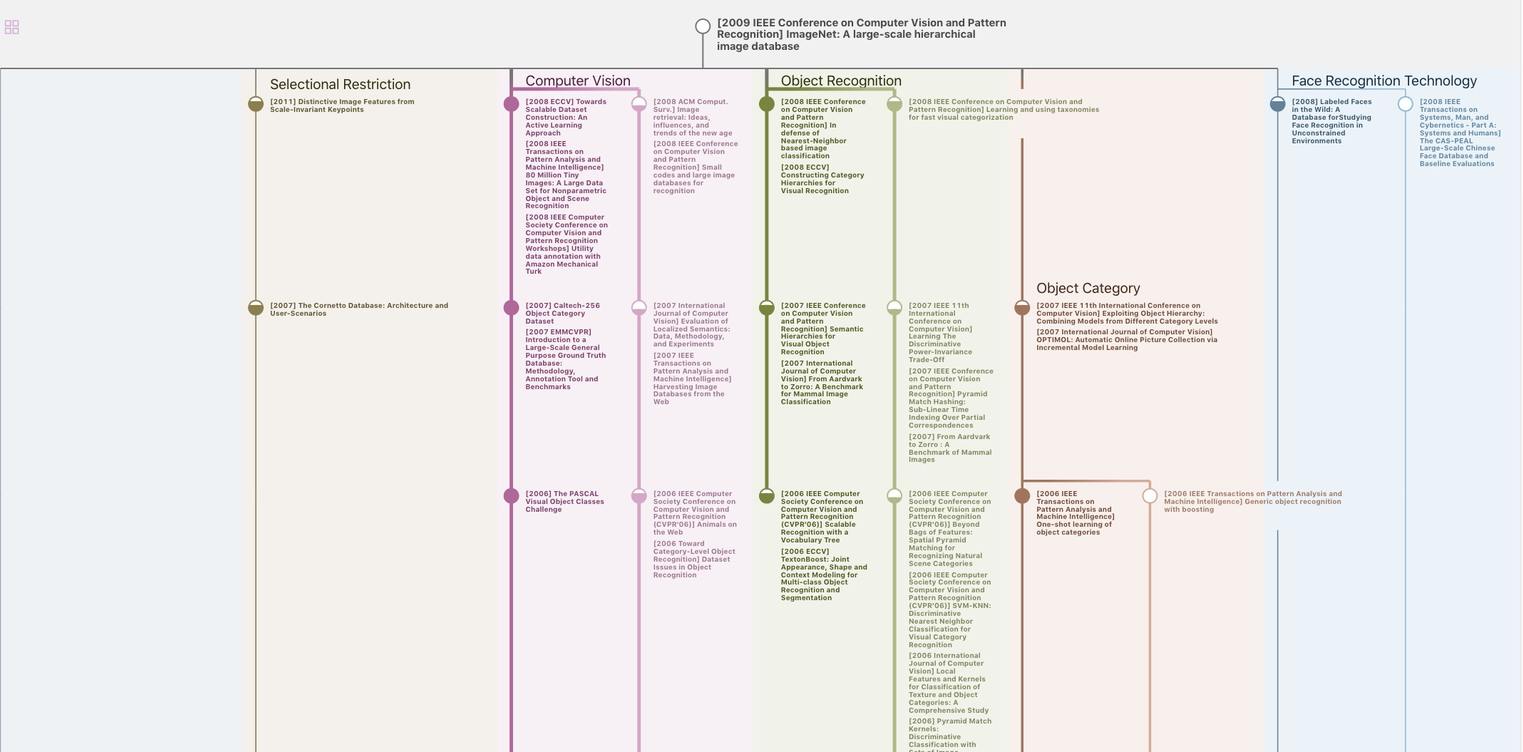
生成溯源树,研究论文发展脉络
Chat Paper
正在生成论文摘要