Shaking Acoustic Spectral Sub-bands Can Better Regularize Learning in Affective Computing
CoRR(2018)
摘要
In this work, we investigate a recently proposed regularization technique based on multi-branch architectures, called Shake-Shake regularization, for the task of speech emotion recognition. In addition, we also propose variants to incorporate domain knowledge into model configurations. The experimental results demonstrate: $1)$ independently shaking sub-bands delivers favorable models compared to shaking the entire spectral-temporal feature maps. $2)$ with proper patience in early stopping, the proposed models can simultaneously outperform the baseline and maintain a smaller performance gap between training and validation.
更多查看译文
AI 理解论文
溯源树
样例
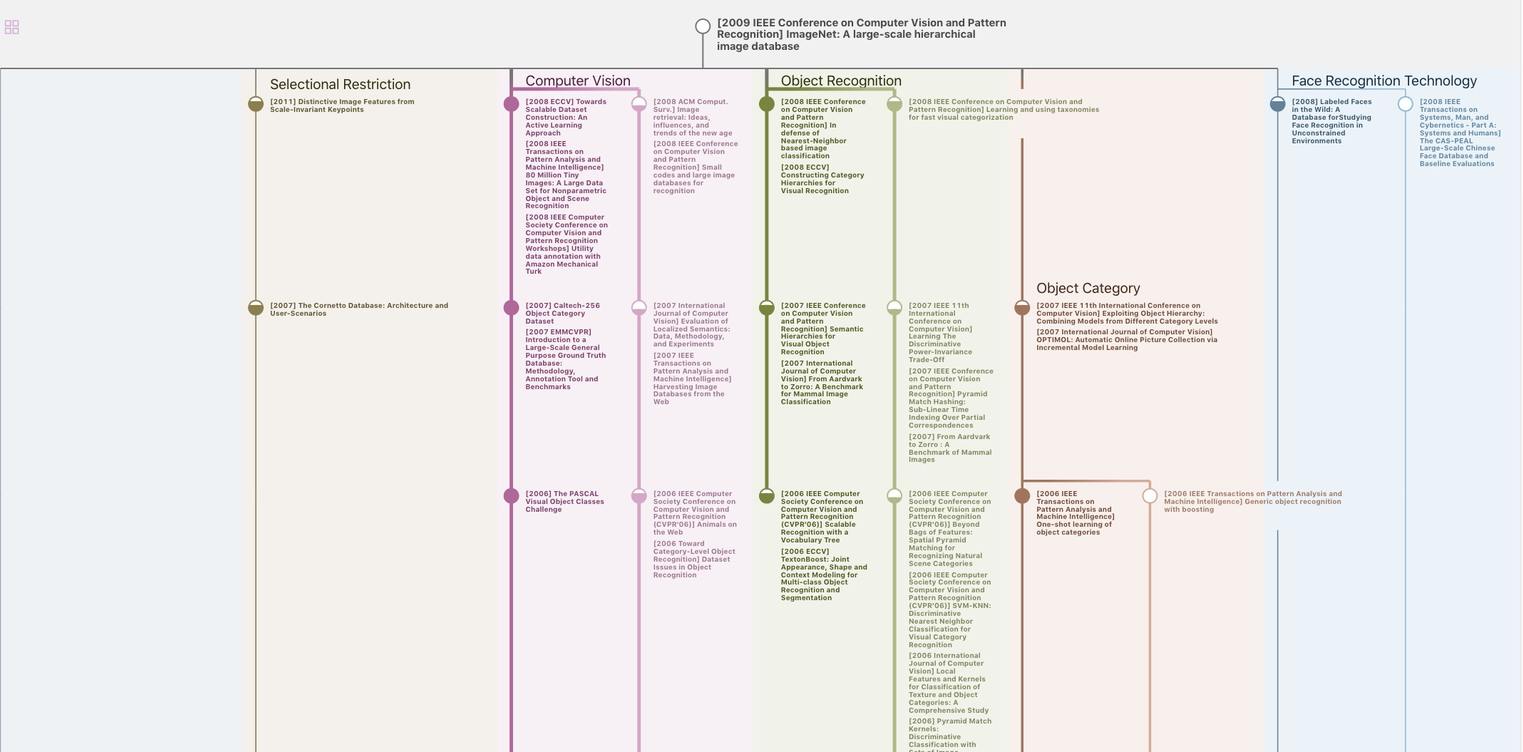
生成溯源树,研究论文发展脉络
Chat Paper
正在生成论文摘要