MRAttractor: Detecting Communities from Large-Scale Graphs
CoRR(2018)
摘要
Detecting groups of users, who have similar opinions, interests, or social behavior, has become an important task for many applications. A recent study showed that dynamic distance based Attractor, a community detection algorithm, outperformed other community detection algorithms such as Spectral clustering, Louvain and Infomap, achieving higher Normalized Mutual Information (NMI) and Adjusted Rand Index (ARI). However, Attractor often takes long time to detect communities, requiring many iterations. To overcome the drawback and handle large-scale graphs, in this paper we propose MRAttractor, an advanced version of Attractor to be runnable on a MapReduce framework. In particular, we (i) apply a sliding window technique to reduce the running time, keeping the same community detection quality; (ii) design and implement the Attractor algorithm for a MapReduce framework; and (iii) evaluate MRAttractor's performance on synthetic and real-world datasets. Experimental results show that our algorithm significantly reduced running time and was able to handle large-scale graphs.
更多查看译文
关键词
MRAttractor,large-scale graphs,community detection algorithm,MapReduce framework,community detection quality,Attractor algorithm,Normalized Mutual Information,sliding window technique
AI 理解论文
溯源树
样例
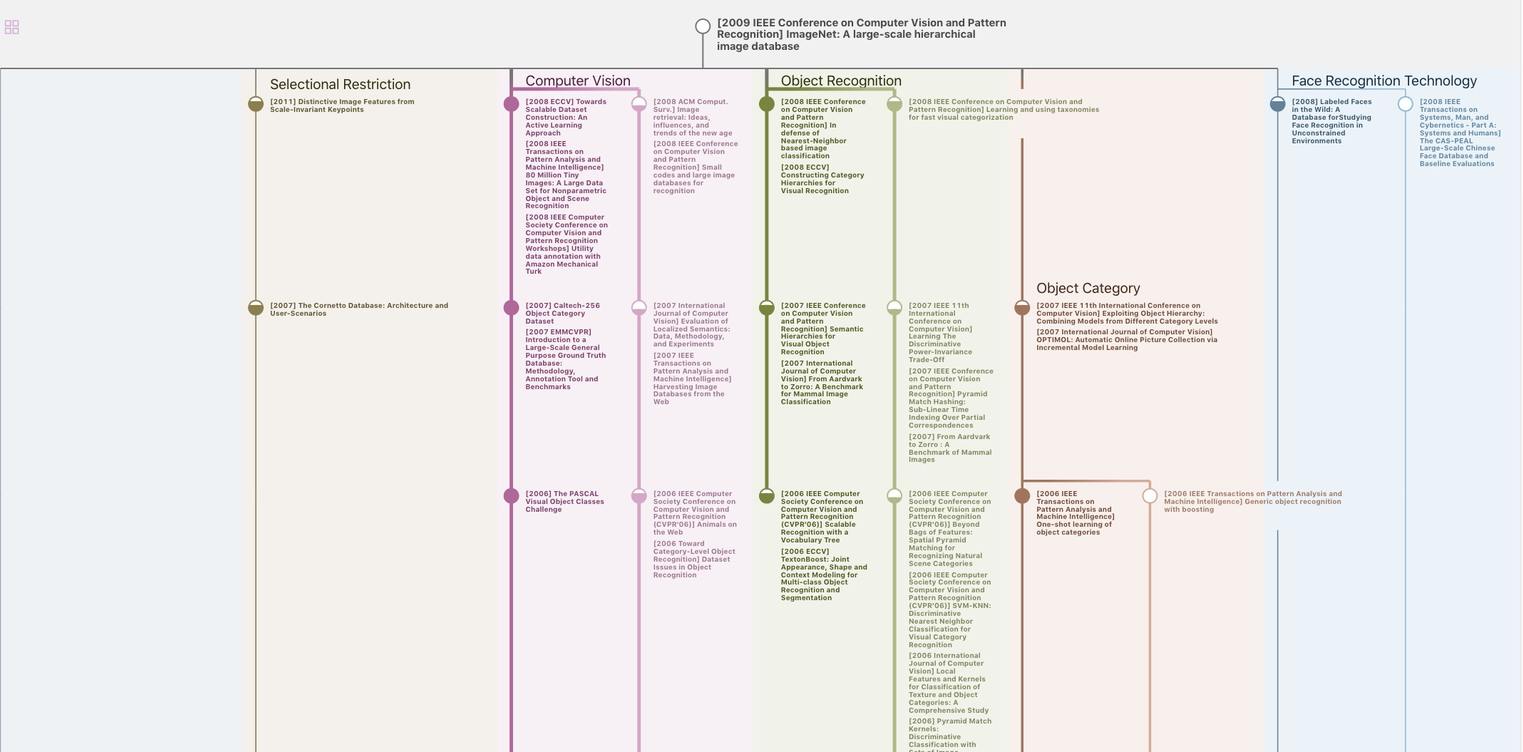
生成溯源树,研究论文发展脉络
Chat Paper
正在生成论文摘要