Advances and Perspectives in Applying Deep Learning for Drug Design and Discovery.
FRONTIERS IN ROBOTICS AND AI(2019)
摘要
Discovering (or planning) a new drug candidate involves many parameters, which makes this process slow, costly, and leading to failures at the end in some cases. In the last decades, we have witnessed a revolution in the computational area (hardware, software, large-scale computing, etc.), as well as an explosion in data generation (big data), which raises the need for more sophisticated algorithms to analyze this myriad of data. In this scenario, we can highlight the potentialities of artificial intelligence (AI) or computational intelligence (CI) as a powerful tool to analyze medicinal chemistry data. According to IEEE, computational intelligence involves the theory, the design, the application, and the development of biologically and linguistically motivated computational paradigms. In addition, CI encompasses three main methodologies: neural networks (NN), fuzzy systems, and evolutionary computation. In particular, artificial neural networks have been successfully applied in medicinal chemistry studies. A branch of the NN area that has attracted a lot of attention refers to deep learning (DL) due to its generalization power and ability to extract features from data. Therefore, in this mini-review we will briefly outline the present scope, advances, and challenges related to the use of DL in drug design and discovery, describing successful studies involving quantitative structure-activity relationships (QSAR) and virtual screening (VS) of databases containing thousands of compounds.
更多查看译文
关键词
artificial intelligence,deep learning,medicinal chemistry,drug design,drug discovery
AI 理解论文
溯源树
样例
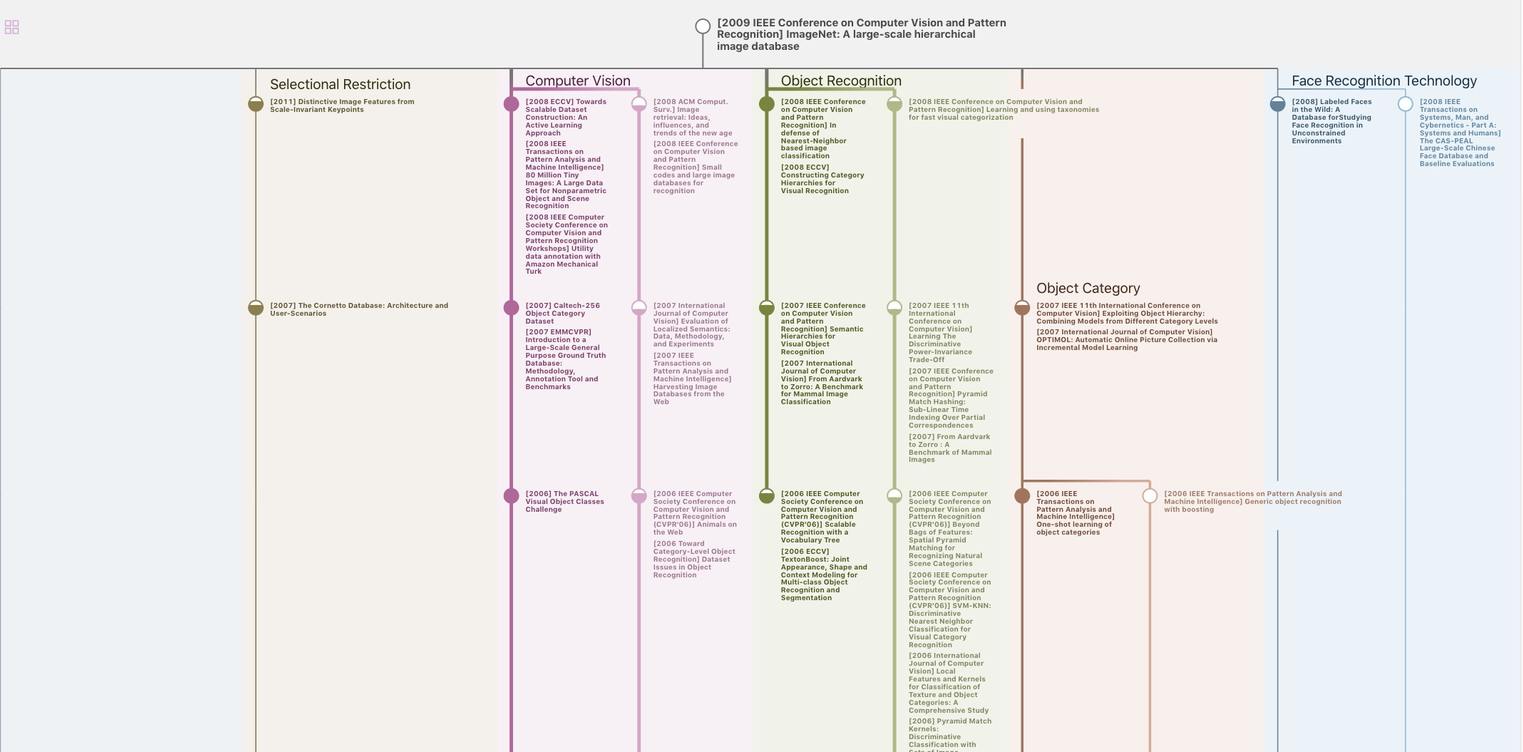
生成溯源树,研究论文发展脉络
Chat Paper
正在生成论文摘要