Galaxy Image Classification Based on Citizen Science Data: A Comparative Study.
IEEE ACCESS(2020)
摘要
Many research fields are now faced with huge volumes of data automatically generated by specialised equipment. Astronomy is a discipline that deals with large collections of images difficult to handle by experts alone. As a consequence, astronomers have been relying on the power of the crowds, as a form of citizen science, for the classification of galaxy images by amateur people. However, the new generation of telescopes that will produce images at a higher rate highlights the limitations of this approach, and the use of machine learning methods for automatic classification is considered essential. The goal of this paper is to shed light on the automated classification of galaxy images exploring two distinct machine learning strategies. First, following the classical approach consisting of feature extraction together with a classifier, we compare the state-of-the-art feature extractor for this problem, the WND-CHARM, with our proposal based on autoencoders for feature extraction on galaxy images. We then compare these results with an end-to-end classification using convolutional neural networks. To better leverage the available citizen science data, we also investigate a pre-training scheme that exploits both amateur- and expert-labelled data. Our experiments reveal that autoencoders greatly speed up feature extraction in comparison with WND-CHARM and both classification strategies, either using convolutional neural networks or feature extraction, reach comparable accuracy. The use of pre-training in convolutional neural networks, however, has allowed us to provide even better results.
更多查看译文
关键词
Astroinformatics,autoencoders,citizen science,convolutional neural networks,deep learning,feature extraction,galaxy morphologies,image classification
AI 理解论文
溯源树
样例
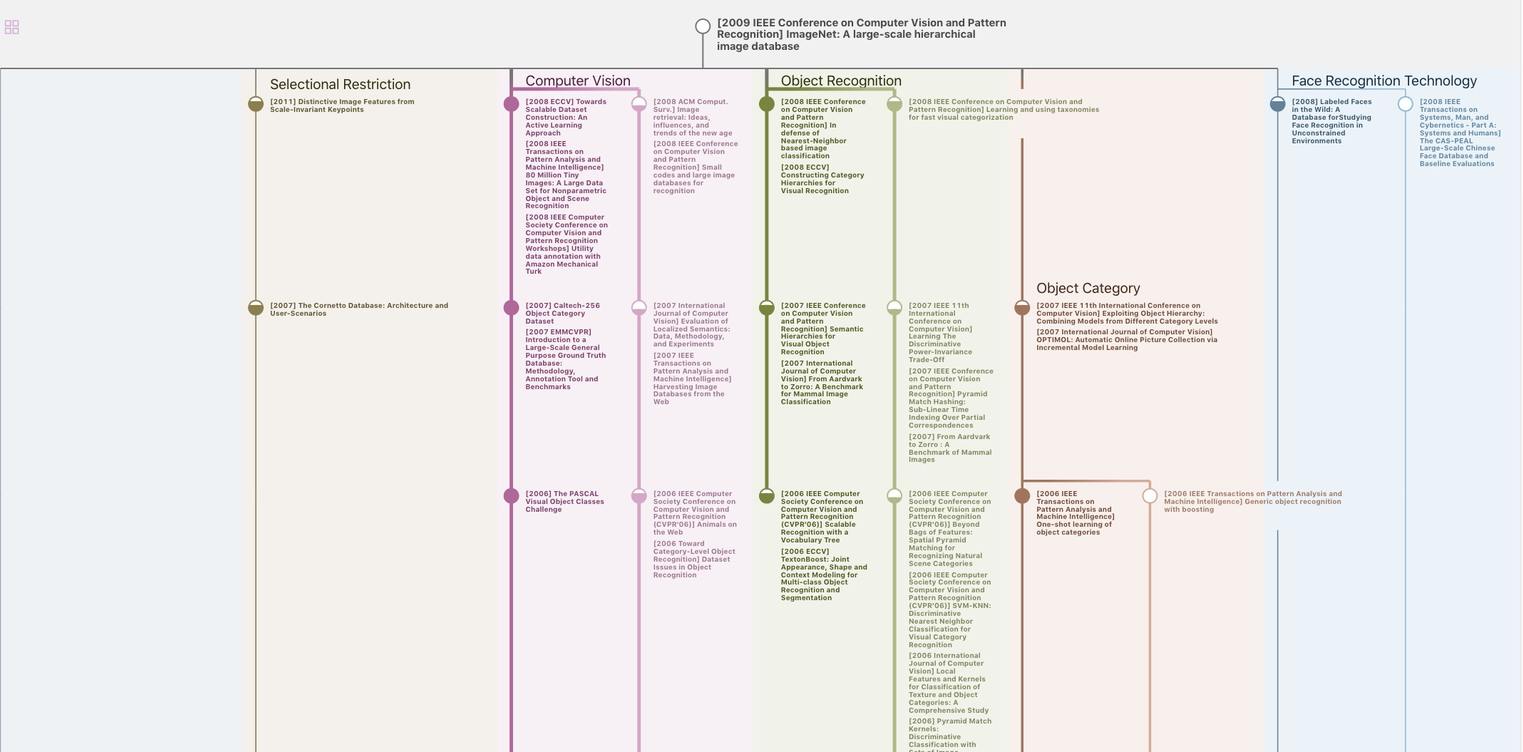
生成溯源树,研究论文发展脉络
Chat Paper
正在生成论文摘要