Robust Poisson Multi-Bernoulli Mixture Filter With Inaccurate Process and Measurement Noise Covariances.
IEEE ACCESS(2020)
摘要
This paper proposes a robust Poisson multi-Bernoulli mixture (PMBM) filter with inaccurate process and measurement noise covariances. A derivation of the robust PMBM filter is provided for jointly estimating the kinematic state, the predicted state covariance, and the measurement noise covariance. By modeling the augmented state as a Gaussian inverse Wishart inverse Wishart (GIWIW) distribution, a computationally feasible implementation of the robust PMBM (GIWIW-PMBM) filter is given for linear Gaussian systems. To guarantee the conjugacy of the GIWIW distribution, the variational Bayesian (VB) approach is employed to approximate the posterior density. Finally, simulation results show that the GIWIW-PMBM filter has the best overall performance compared to existing state-of-the-art filters regarding computational cost and filtering performance.
更多查看译文
关键词
Robust Poisson multi-Bernoulli mixture filter,Gaussian inverse Wishart inverse Wishart,conjugate prior,variational Bayesian,inaccurate process and measurement noise covariances
AI 理解论文
溯源树
样例
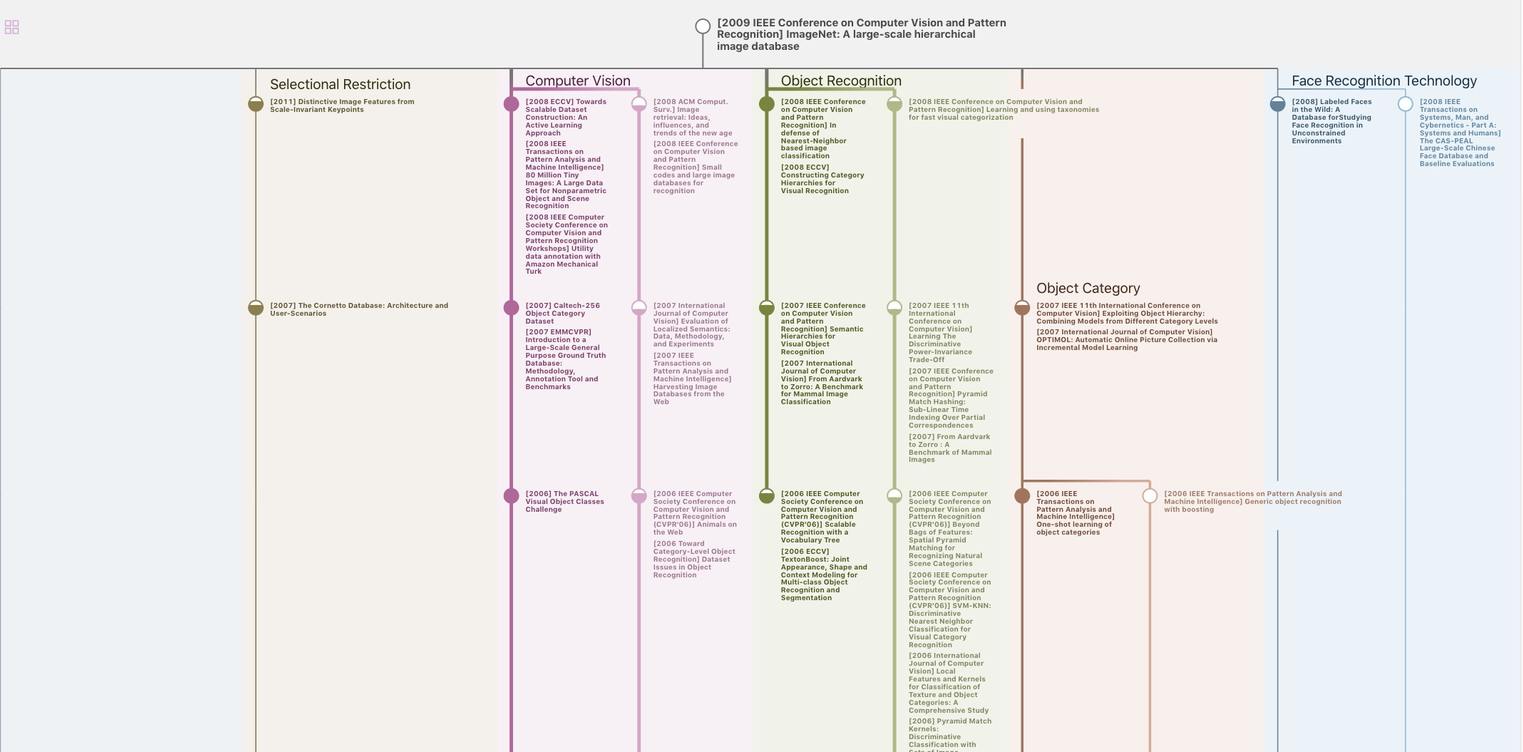
生成溯源树,研究论文发展脉络
Chat Paper
正在生成论文摘要