Exploring Common and Label-Specific Features for Multi-Label Learning With Local Label Correlations.
IEEE ACCESS(2020)
摘要
In multi-label learning, instances can be associated with a set of class labels. The existing multi-label feature selection (MLFS) methods generally adopt either of these two strategies, namely, selecting a subset of features that is shared by all labels (common features) or exploring the most discriminative features for each label (label-specific features). However, both of them can play a key role in the discrimination of different labels. For example, common features can distinguish all labels, and label-specific features contribute to discriminating label's differences. They are important for the discriminability of selected features. On the other hand, it is well-known that exploiting label correlations can advance the performance of MLFS, and label correlations are local and only shared by a data subset in most cases. How to effectively learn and exploit local label correlations in the selection process is significant. In this paper, to address these problems, we propose a novel MLFS framework. Specially, common and label-specific features are simultaneously considered by introducing both l(2,1)-norm and l(1)-norm regularizers, local label correlations are automatically learned with probability and learned correlation information is efficiently exploited to help feature selection by constraining label correlations on the output of labels. A comparative study with seven state-of-the-art methods manifests the efficacy of our framework.
更多查看译文
关键词
Correlation,Feature extraction,Training data,Optimization,Licenses,Image color analysis,Frequency modulation,Multi-label,feature selection,label correlations,label-specific features
AI 理解论文
溯源树
样例
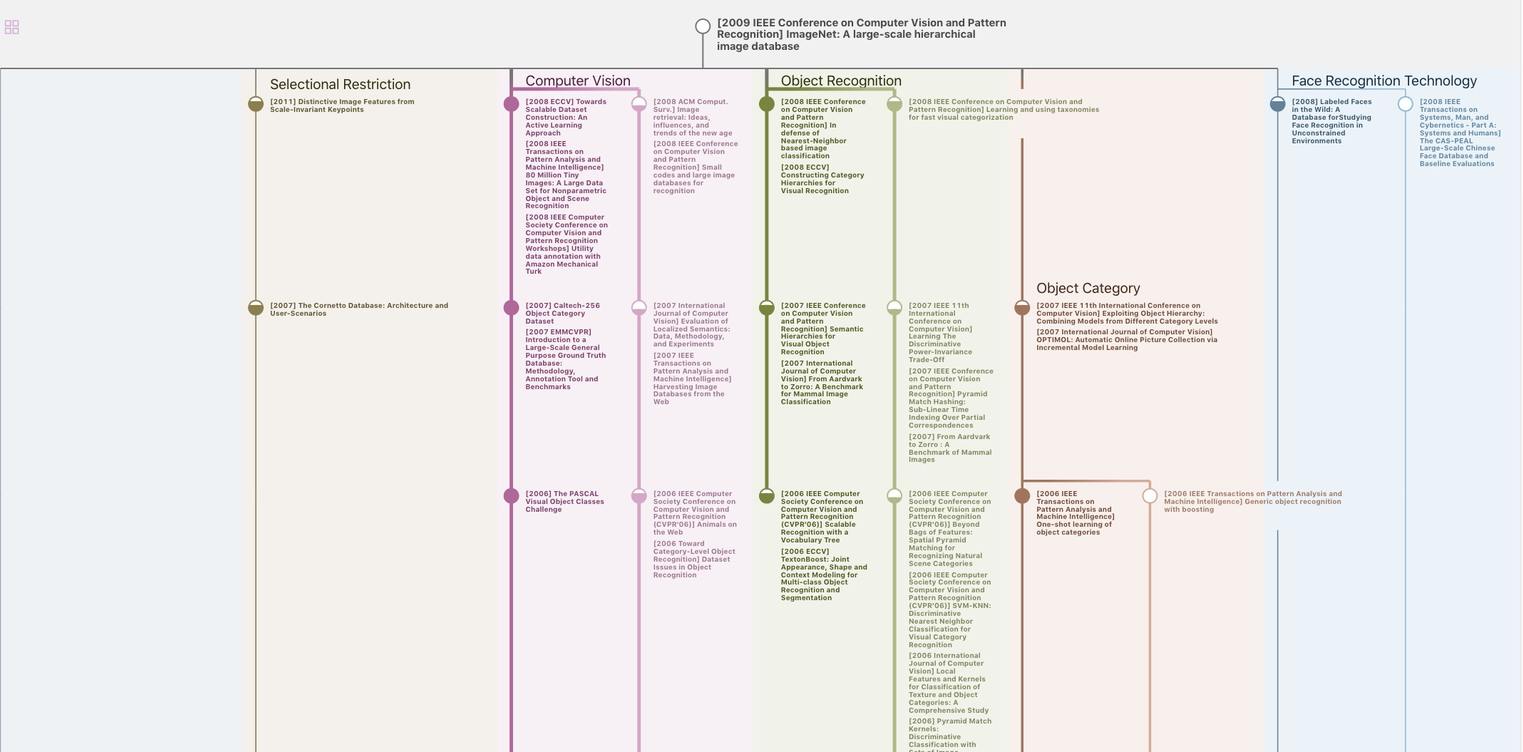
生成溯源树,研究论文发展脉络
Chat Paper
正在生成论文摘要