Soft Dropout Method In Training Of Contextual Neural Networks
INTELLIGENT INFORMATION AND DATABASE SYSTEMS (ACIIDS 2020), PT II(2020)
摘要
Various regularization techniques were developed to prevent many adverse effects that may appear during the training of contextual and non-contextual neural networks. The problems include e.g.: overfitting, vanishing of the gradient and too high increase in weight values. A commonly used solution that limits many of those is the dropout. The goal of this paper is to propose and analyze a new type of dropout - Soft Dropout. Unlike traditional dropout regularization, in Soft Dropout neurons are excluded only partially, what is regulated by additional, continuous muting factor. This change can help to generate classification models with lower overfitting. The paper present results suggesting that Soft Dropout can help to generate classification models with lower overfitting than standard dropout technique. Experiments are performed for selected benchmark and real-life datasets with MLP and Contextual Neural Networks.
更多查看译文
关键词
Dropout, Muting factor, Overfitting
AI 理解论文
溯源树
样例
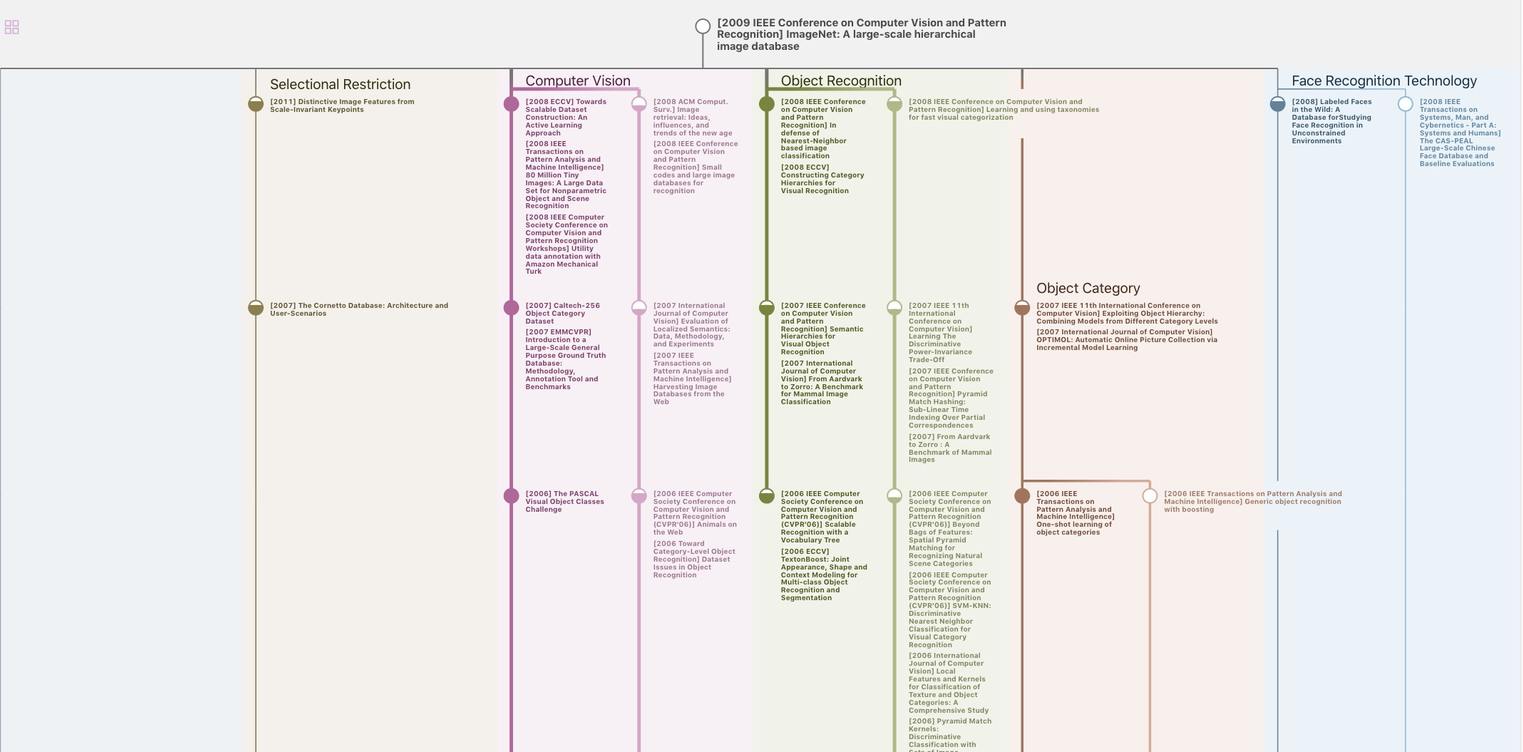
生成溯源树,研究论文发展脉络
Chat Paper
正在生成论文摘要