Optimal Number Of Seed Point Selection Algorithm Of Unknown Dataset
PROCEEDINGS OF 3RD INTERNATIONAL CONFERENCE ON COMPUTER VISION AND IMAGE PROCESSING, CVIP 2018, VOL 2(2020)
摘要
In the present world, clustering is considered to be the most important data mining tool which is applied to huge data to help the futuristic decision-making processes. It is an unsupervised classification technique by which the data points are grouped to form the homogeneous entity. Cluster analysis is used to find out the clusters from a unlabeled data. The position of the seed points primarily affects the performances of most partitional clustering techniques. The correct number of clusters in a dataset plays an important role to judge the quality of the partitional clustering technique. Selection of initial seed of K-means clustering is a critical problem for the formation of the optimal number of the cluster with the benefit of fast stability. In this paper, we have described the optimal number of seed points selection algorithm of an unknown data based on two important internal cluster validity indices, namely, Dunn Index and Silhouette Index. Here, Shannon's entropy with the threshold value of distance has been used to calculate the position of the seed point. The algorithm is applied to different datasets and the results are comparatively better than other methods. Moreover, the comparisons have been done with other algorithms in terms of different parameters to distinguish the novelty of our proposed method.
更多查看译文
关键词
Clustering, Cluster validity indices, Data mining, Seed point, K-means, Shannons entropy
AI 理解论文
溯源树
样例
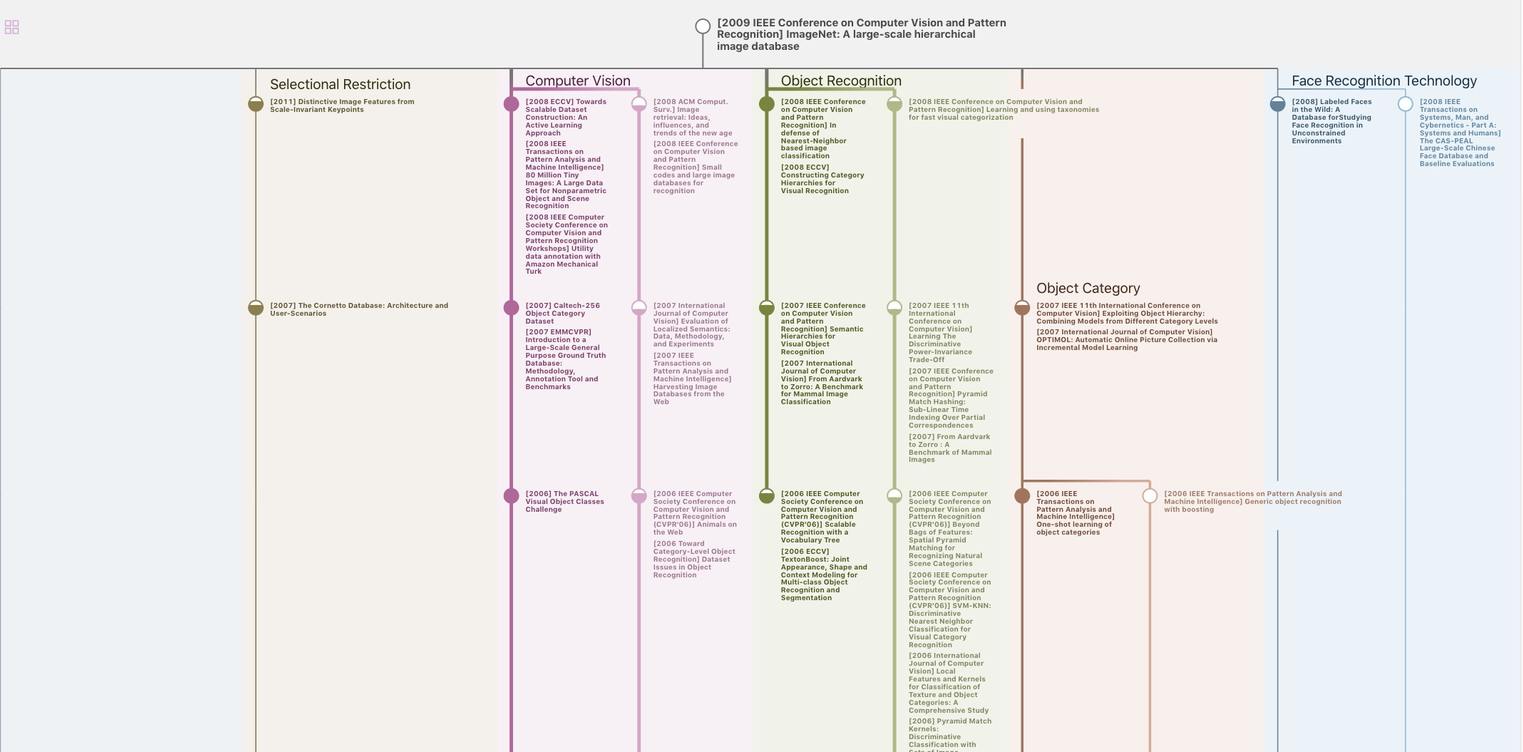
生成溯源树,研究论文发展脉络
Chat Paper
正在生成论文摘要