Assessing parameter uncertainty in small-n pharmacometric analyses: value of the log-likelihood profiling-based sampling importance resampling (LLP-SIR) technique
Journal of Pharmacokinetics and Pharmacodynamics(2020)
摘要
Assessing parameter uncertainty is a crucial step in pharmacometric workflows. Small datasets with ten or fewer subjects appear regularly in drug development and therapeutic use, but it is unclear which method to assess parameter uncertainty is preferable in such situations. The aim of this study was to (i) systematically evaluate the performance of standard error (SE), bootstrap (BS), log-likelihood profiling (LLP), Bayesian approaches (BAY) and sampling importance resampling (SIR) to assess parameter uncertainty in small datasets and (ii) to evaluate methods to provide proposal distributions for the SIR. A simulation study was conducted and the 0–95% confidence interval (CI) and coverage for each parameter was evaluated and compared to reference CIs derived by stochastic simulation and estimation (SSE). A newly proposed LLP-SIR, combining the proposal distribution provided by LLP with SIR, was included in addition to conventional SE-SIR and BS-SIR. Additionally, the methods were applied to a clinical dataset. The determined CIs differed substantially across the methods. The CIs of SE, BS, LLP and BAY were not in line with the reference in datasets with ≤ 10 subjects. The best alignment was found for the LLP-SIR, which also provided the best coverage results among the SIR methods. The best overall results regarding the coverage were provided by LLP and BAY across all parameters and dataset sizes. To conclude, the popular SE and BS methods are not suitable to derive parameter uncertainty in small datasets containing ≤ 10 subjects, while best performances were observed with LLP, BAY and LLP-SIR.
更多查看译文
关键词
Parameter uncertainty,Small datasets,Sampling importance resampling,Bootstrap,Log-likelihood profiling,LLP-SIR
AI 理解论文
溯源树
样例
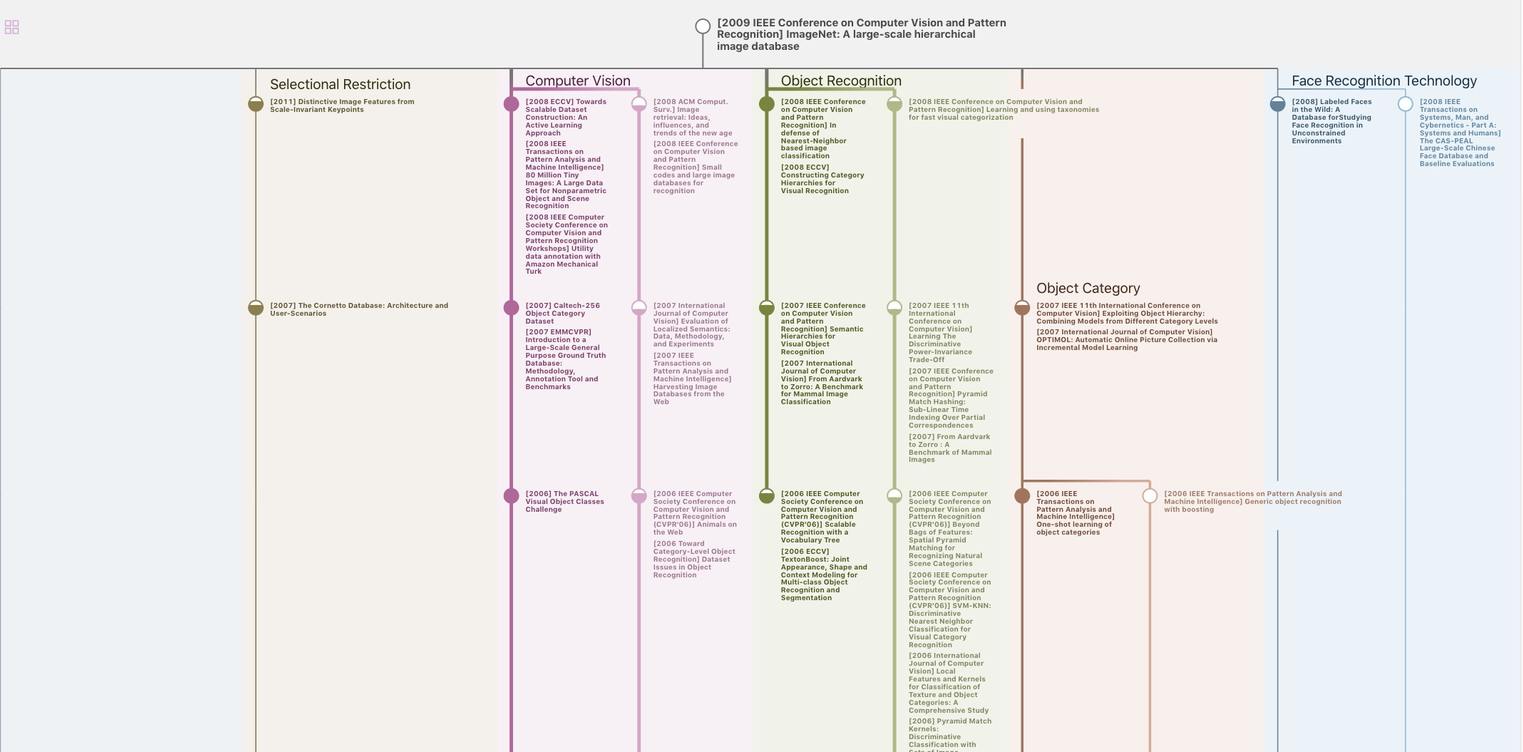
生成溯源树,研究论文发展脉络
Chat Paper
正在生成论文摘要