Latency-conscious dataflow reconfiguration
SIGMOD/PODS '18: International Conference on Management of Data Houston TX USA June, 2018(2018)
摘要
We propose a prototype incremental data migration mechanism for stateful distributed data-parallel dataflow engines with latency objectives. When compared to existing scaling mechanisms, our prototype has the following differentiating characteristics: (i) the mechanism provides tunable granularity for avoiding latency spikes, (ii) reconfigurations can be prepared ahead of time to avoid runtime coordination, and (iii) the implementation only relies on existing dataflow APIs and need not require system modifications.
We demonstrate our proposal on example computations with varying amounts of state that needs to be migrated, which is a non-trivial task for systems like Dhalion and Flink. Our implementation, prototyped on Timely Dataflow, provides a scalable stateful operator template compatible with existing APIs that carefully reorganizes data to minimize migration overhead. Compared to naïve approaches we reduce service latencies by orders of magnitude.
更多查看译文
AI 理解论文
溯源树
样例
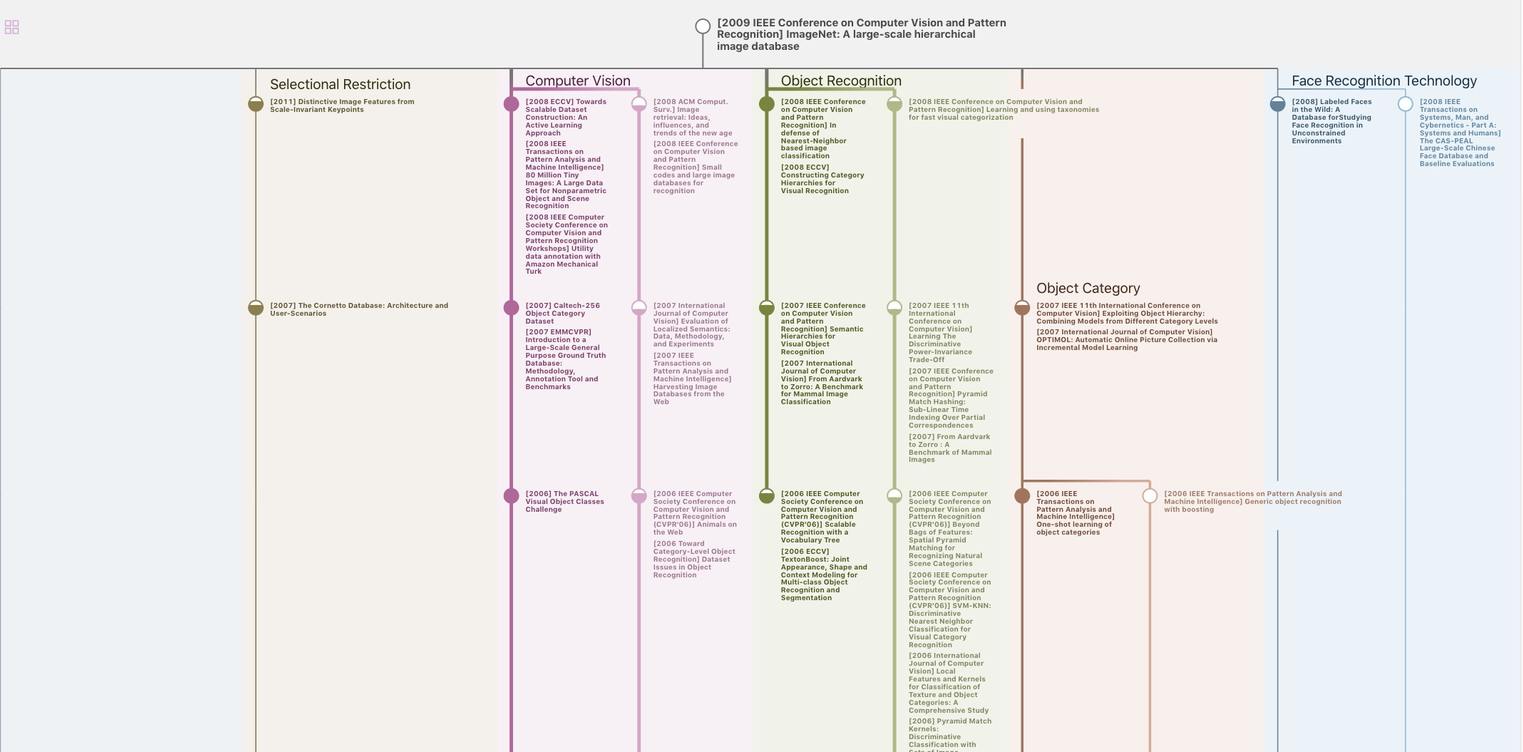
生成溯源树,研究论文发展脉络
Chat Paper
正在生成论文摘要