Neural Network Assisted Compact Model for Accurate Characterization of Cycle-to-cycle Variations in 2-D $h$ -BN based RRAM devices
2019 Device Research Conference (DRC)(2019)
摘要
Resistive random-access memory (RRAM) is one of the most promising candidates for realizing the next generation of non-volatile memories and neuromorphic computing architectures. Although these devices have been extensively researched; accurate modeling of non-ideal effects such as cycle-to-cycle (C2C) variation and resistance drift remain challenging due to the stochastic nature of filament formation and filament evolution [1]-[3]. In this paper, resistive switching (RS) in 2-dimensional (2D) hexagonal Boron Nitride ( h -BN) is demonstrated, and techniques for modeling C2C variations are presented. Using an autoregressive neural network, a VerilogA model for RRAM is created to accurately reproduce experimentally observed C2C variations. This modeling approach is intended to aid circuit designers in evaluating stochastic phenomenon at the large array level and model emerging devices using advanced materials in their early phase of technology development.
更多查看译文
关键词
nonideal effects,resistance drift,filament formation,filament evolution,resistive switching,2-dimensional hexagonal Boron Nitride,autoregressive neural network,VerilogA model,neural network assisted compact model,cycle-to-cycle variations,resistive random-access memory,nonvolatile memories,neuromorphic computing architectures,2D h-BN based RRAM devices,C2C variations,BN
AI 理解论文
溯源树
样例
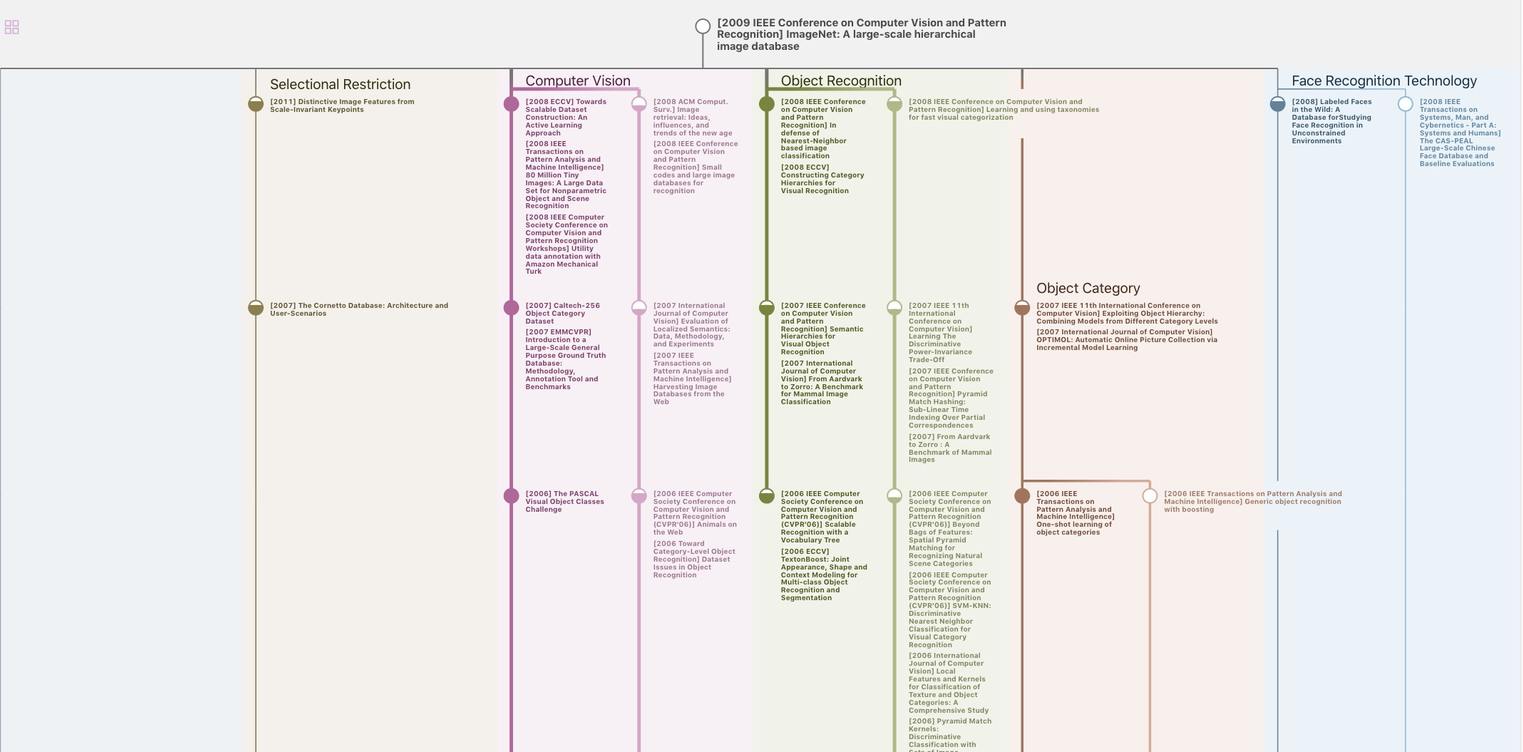
生成溯源树,研究论文发展脉络
Chat Paper
正在生成论文摘要