Goldilocks: Learning Pattern-Based Task Assignment In Mobile Crowdsensing
QUALITY, RELIABILITY, SECURITY AND ROBUSTNESS IN HETEROGENEOUS SYSTEMS(2020)
摘要
Mobile crowdsensing (MCS) depends on mobile users to collect sensing data, whose quality highly depends on the expertise/experience of the users. It is critical for MCS to identify right persons for a given sensing task. A commonly-used strategy is to "teach-before-use", i.e., training users with a set of questions and selecting a subset of users who have answered the questions correctly the most of times. This method has large room for improvement if we consider users' learning curve during the training process. As such, we propose an interactive learning pattern recognition framework, Goldilocks, that can filter users based on their learning patterns. Goldilocks uses an adaptive teaching method tailored for each user to maximize her learning performance. At the same time, the teaching process is also the selecting process. A user can thus be safely excluded as early as possible from the MCS tasks later on if her performance still does not match the desired learning pattern after the training period. Experiments on real-world datasets show that compared to the baseline methods, Goldilocks can identify suitable users to obtain more accurate and more stable results for multi-categories classification problems.
更多查看译文
关键词
Mobile crowdsensing, Learning pattern recognition, Task assignment
AI 理解论文
溯源树
样例
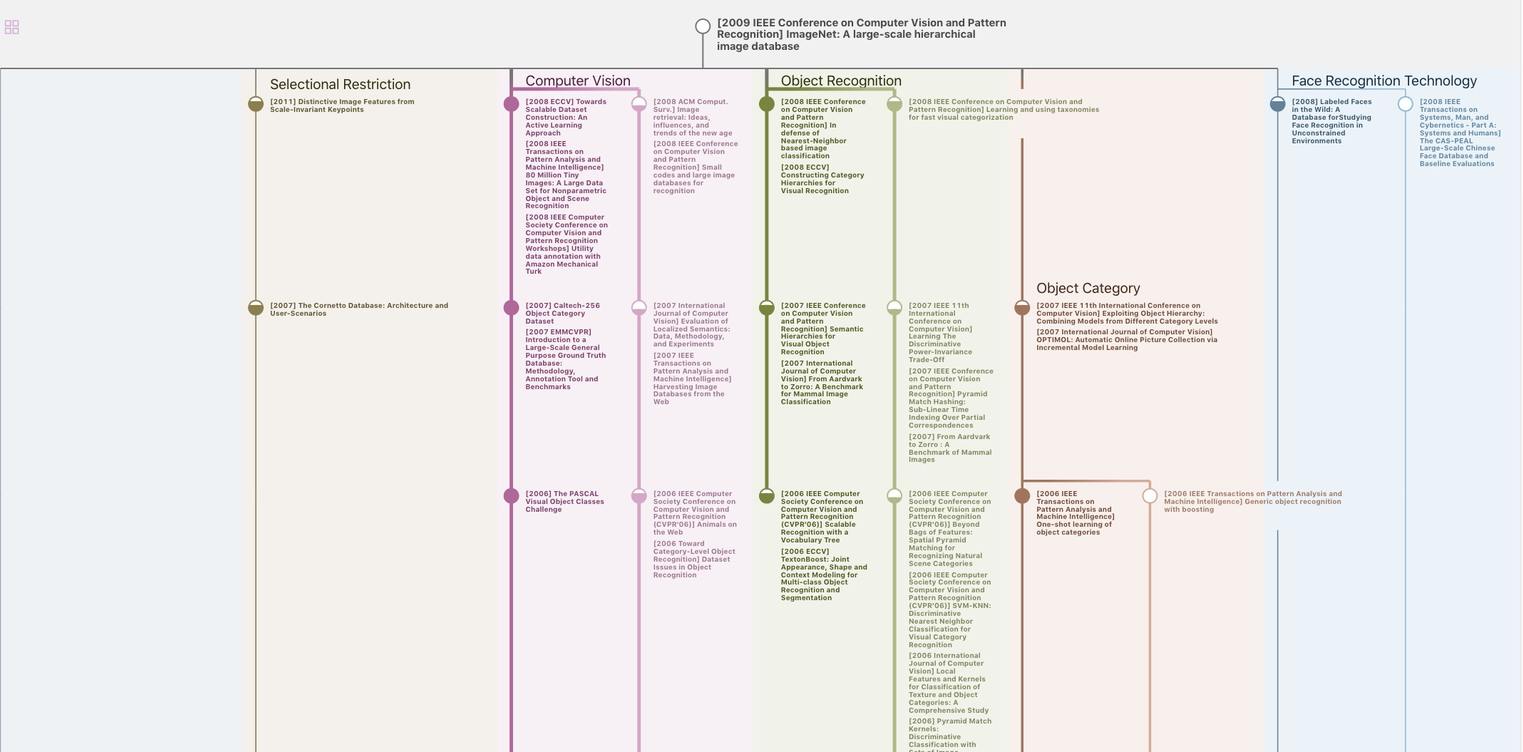
生成溯源树,研究论文发展脉络
Chat Paper
正在生成论文摘要