A Sampling Theorem For Deconvolution In Two Dimensions
SIAM JOURNAL ON IMAGING SCIENCES(2020)
摘要
This work studies the problem of estimating a two-dimensional superposition of point sources or spikes from samples of their convolution with a Gaussian kernel. Our results show that minimizing a continuous counterpart of the l(1)-norm exactly recovers the true spikes if they are sufficiently separated, and the samples are sufficiently dense. In addition, we provide numerical evidence that our results extend to non-Gaussian kernels relevant to microscopy and telescopy.
更多查看译文
关键词
deconvolution, sampling theory, convex optimization, sparsity, super-resolution, dual certificate, Gaussian convolution
AI 理解论文
溯源树
样例
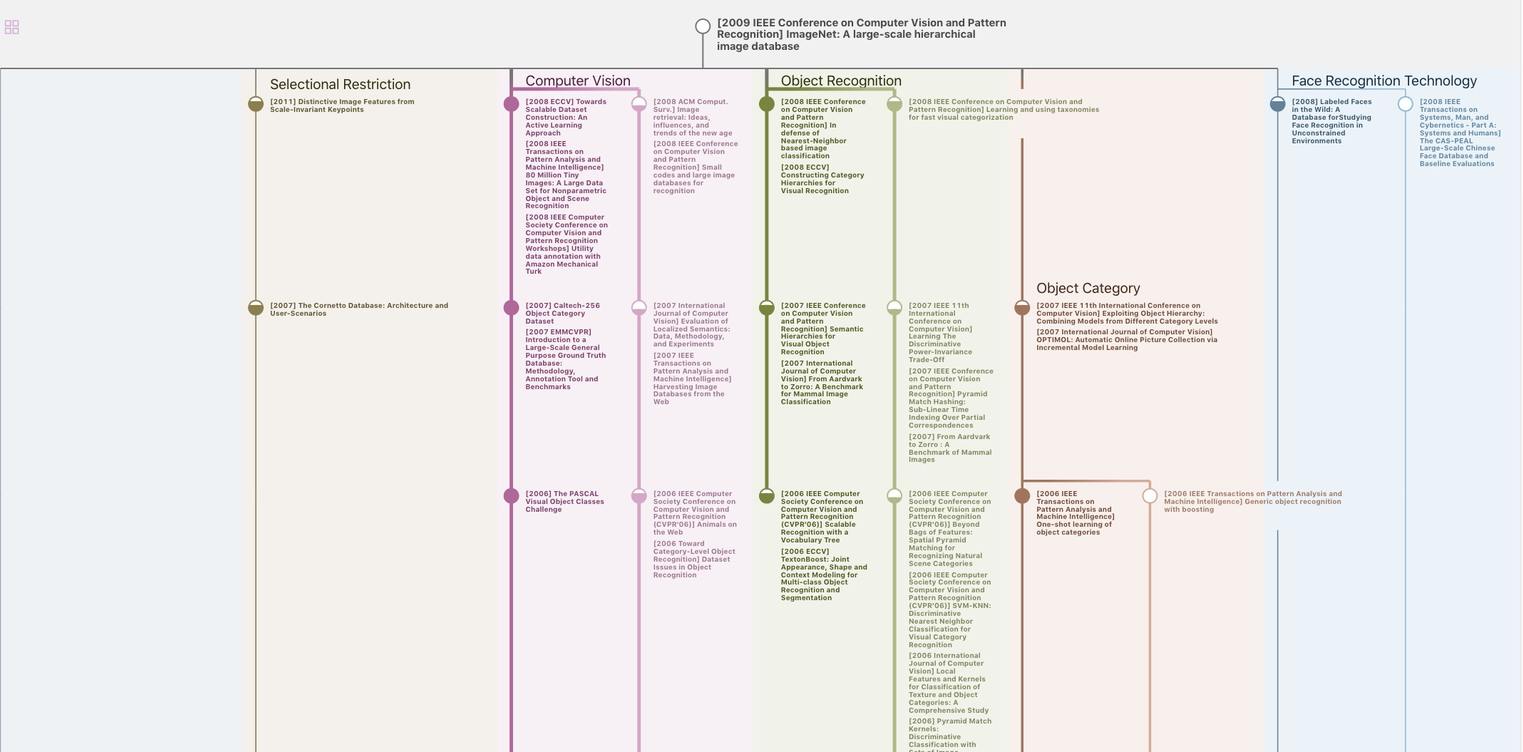
生成溯源树,研究论文发展脉络
Chat Paper
正在生成论文摘要