Enhancing Generalization of Wafer Defect Detection by Data Discrepancy-aware Preprocessing and Contrast-varied Augmentation
2020 25th Asia and South Pacific Design Automation Conference (ASP-DAC)(2020)
摘要
Wafer inspection locates defects at early fabrication stages and traditionally focuses on pixel-level defects. However, there are very few solutions that can effectively detect largescale defects. In this work, we leverage Convolutional Neural Networks (CNNs) to automate the wafer inspection process and propose several techniques to preprocess and augment wafer images for enhancing our model's generalization on unseen wafers (e.g., from other fabs). Cross-fab experimental results of both wafer-level and pixel-level detections show that the F1 score increases from 0.09 to 0.77 and the Precision-Recall area under curve (PR AUC) increases from 0.03 to 0.62 using our proposed method.
更多查看译文
关键词
convolutional neural networks,wafer inspection process,cross-fab experimental results,wafer defect detection,data discrepancy-aware preprocessing,contrast-varied augmentation,CNNs,precision-recall area under curve,PR AUC,wafer images processing
AI 理解论文
溯源树
样例
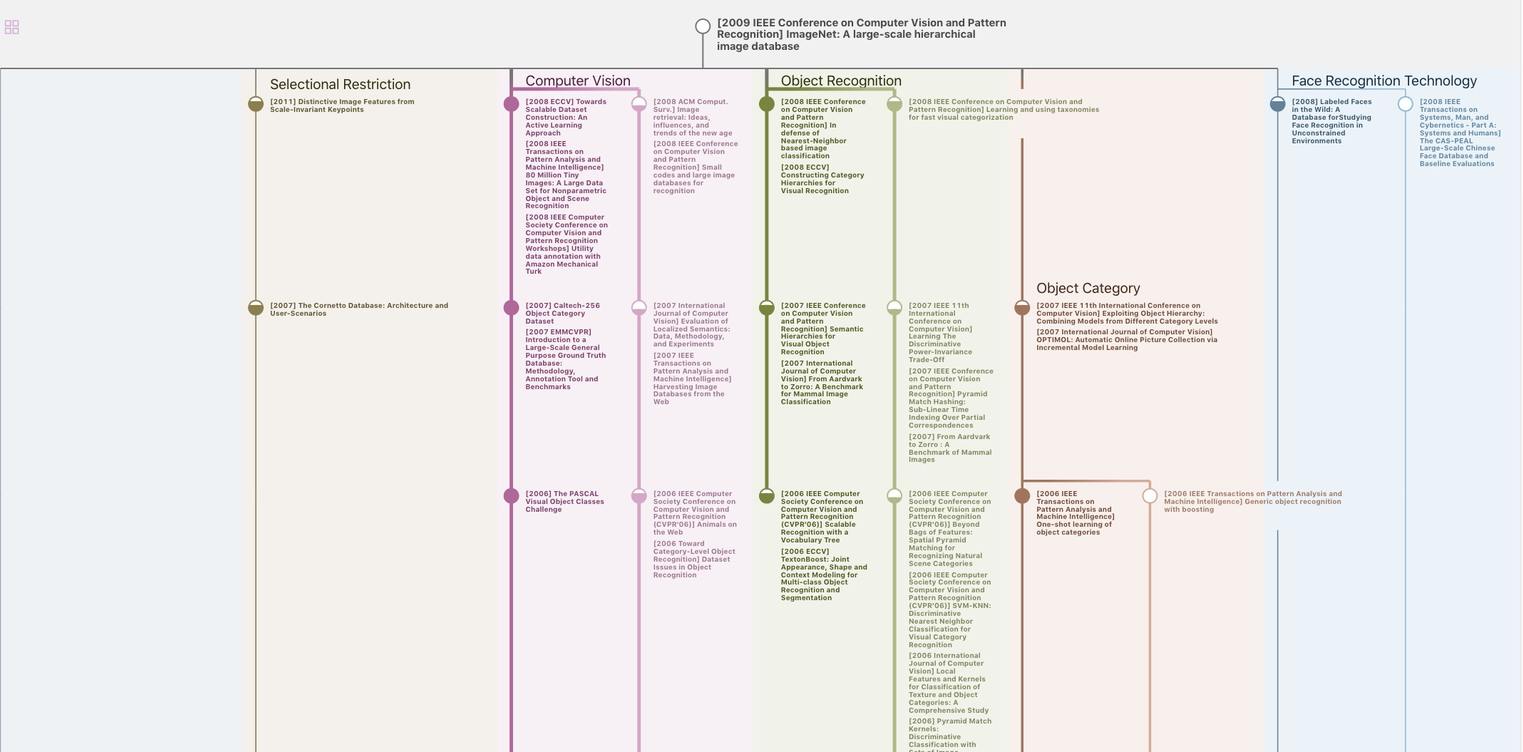
生成溯源树,研究论文发展脉络
Chat Paper
正在生成论文摘要