Improving Code-mixed POS Tagging Using Code-mixed Embeddings
ACM Transactions on Asian and Low-Resource Language Information Processing(2020)
摘要
Social media data has become invaluable component of business analytics. A multitude of nuances of social media text make the job of conventional text analytical tools difficult. Code-mixing of text is a phenomenon prevalent among social media users, wherein words used are borrowed from multiple languages, though written in the commonly understood roman script. All the existing supervised learning methods for tasks such as Parts Of Speech (POS) tagging for code-mixed social media (CMSM) text typically depend on a large amount of training data. Preparation of such large training data is resource-intensive, requiring expertise in multiple languages. Though the preparation of small dataset is possible, the out of vocabulary (OOV) words pose major difficulty, while learning models from CMSM text as the number of different ways of writing non-native words in roman script is huge. POS tagging for code-mixed text is non-trivial, as tagging should deal with syntactic rules of multiple languages. The important research question addressed by this article is whether abundantly available unlabeled data can help in resolving the difficulties posed by code-mixed text for POS tagging. We develop an approach for scraping and building word embeddings for code-mixed text illustrating it for Bengali-English, Hindi-English, and Telugu-English code-mixing scenarios. We used a hierarchical deep recurrent neural network with linear-chain CRF layer on top of it to improve the performance of POS tagging in CMSM text by capturing contextual word features and character-sequence–based information. We prepared a labeled resource for POS tagging of CMSM text by correcting 19% of labels from an existing resource. A detailed analysis of the performance of our approach with varying levels of code-mixing is provided. The results indicate that the F1-score of our approach with custom embeddings is better than the CRF-based baseline by 5.81%, 5.69%, and 6.3% in Bengali, Hindi, and Telugu languages, respectively.
更多查看译文
关键词
Parts of speech tagging,code-mixed (multi-lingual) text,deep neural networks
AI 理解论文
溯源树
样例
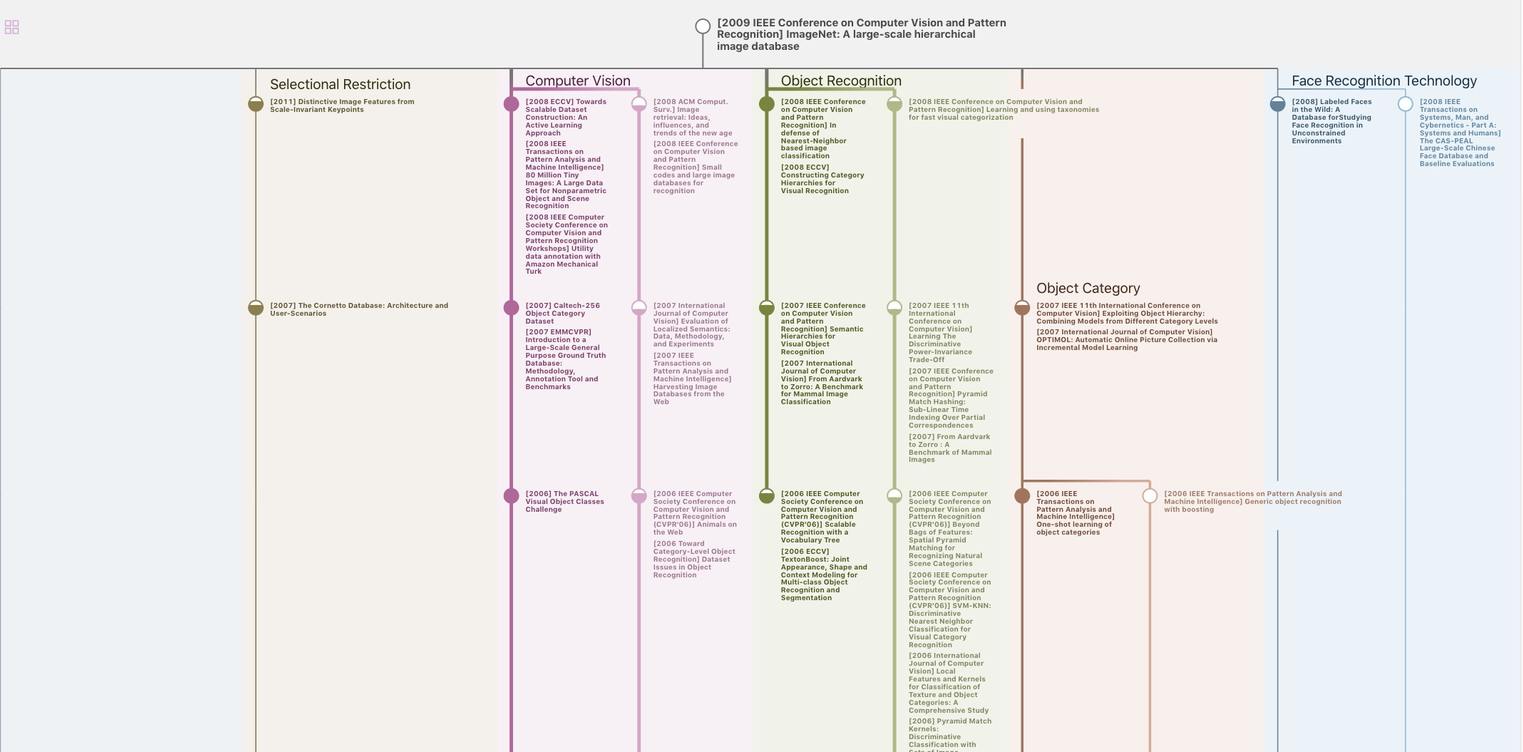
生成溯源树,研究论文发展脉络
Chat Paper
正在生成论文摘要