Fair sequential group recommendations
SAC '20: The 35th ACM/SIGAPP Symposium on Applied Computing Brno Czech Republic March, 2020(2020)
摘要
Recommender systems have been incorporated in our everyday life; from music to health recommendations, recommender systems have enhanced the users' experience. At the same time, with the expansion of social media, it is now easier than ever to form groups of people. As such, group recommenders have become more popular. Often, we consider the interaction between a group and the recommender system as a stand-alone process; the group requests some suggestions from the system and the system answers without any considerations to past interactions. A more realistic scenario is for a system to require access to history logs, and take them into account when recommending items for a group of users. Not only what items the system previously had recommended, but also, how well were these items received by the members of the group. In this work, we propose a sequential group recommender, which is aware of the past interactions of the group with the system. We introduce the notion of satisfaction that describes how relevant are the recommended items to each member of the group. We utilize satisfaction in a novel aggregation method that achieves to make our model fair for all members of the group. We show with experimental results that the typical group recommendation approaches are substandard to our proposed method.
更多查看译文
关键词
Recommender systems, sequential recommendations, group recommendations, fair recommendations.
AI 理解论文
溯源树
样例
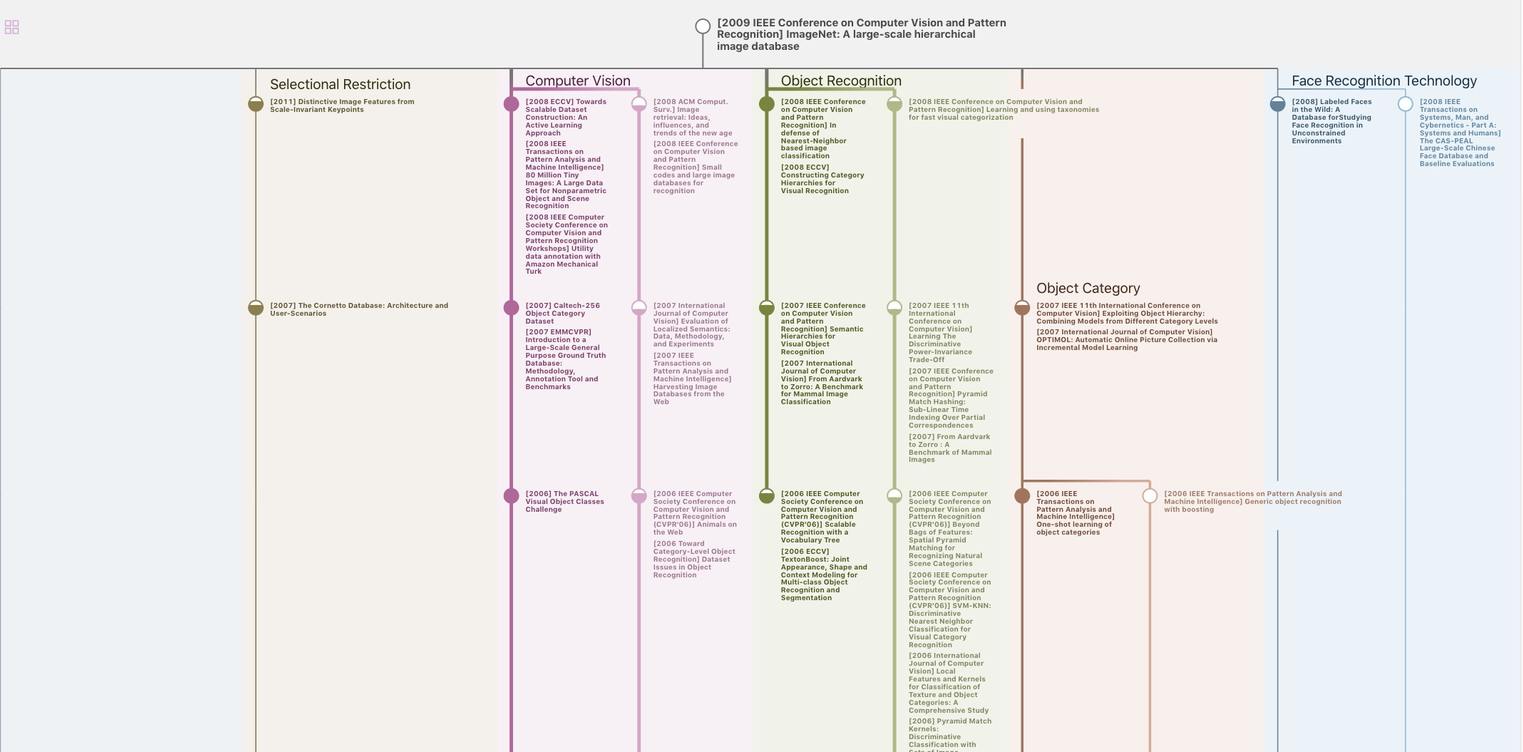
生成溯源树,研究论文发展脉络
Chat Paper
正在生成论文摘要