Zero-Assignment Constraint For Graph Matching With Outliers
2020 IEEE/CVF CONFERENCE ON COMPUTER VISION AND PATTERN RECOGNITION (CVPR)(2020)
摘要
Graph matching (GM), as a longstanding problem in computer vision and pattern recognition, still suffers from numerous cluttered outliers in practical applications. To address this issue, we present the zero-assignment constraint (ZAC) for approaching the graph matching problem in the presence of outliers. The underlying idea is to suppress the matchings of outliers by assigning zero-valued vectors to the potential outliers in the obtained optimal correspondence matrix. We provide elaborate theoretical analysis to the problem, i.e., GM with ZAC, and figure out that the GM problem with and without outliers are intrinsically different, which enables us to put forward a sufficient condition to construct valid and reasonable objective function. Consequently, we design an efficient outlier-robust algorithm to significantly reduce the incorrect or redundant matchings caused by numerous outliers. Extensive experiments demonstrate that our method can achieve the stateof-the-art performance in terms of accuracy and efficiency, especially in the presence of numerous outliers.
更多查看译文
关键词
graph matching,zero-assignment
AI 理解论文
溯源树
样例
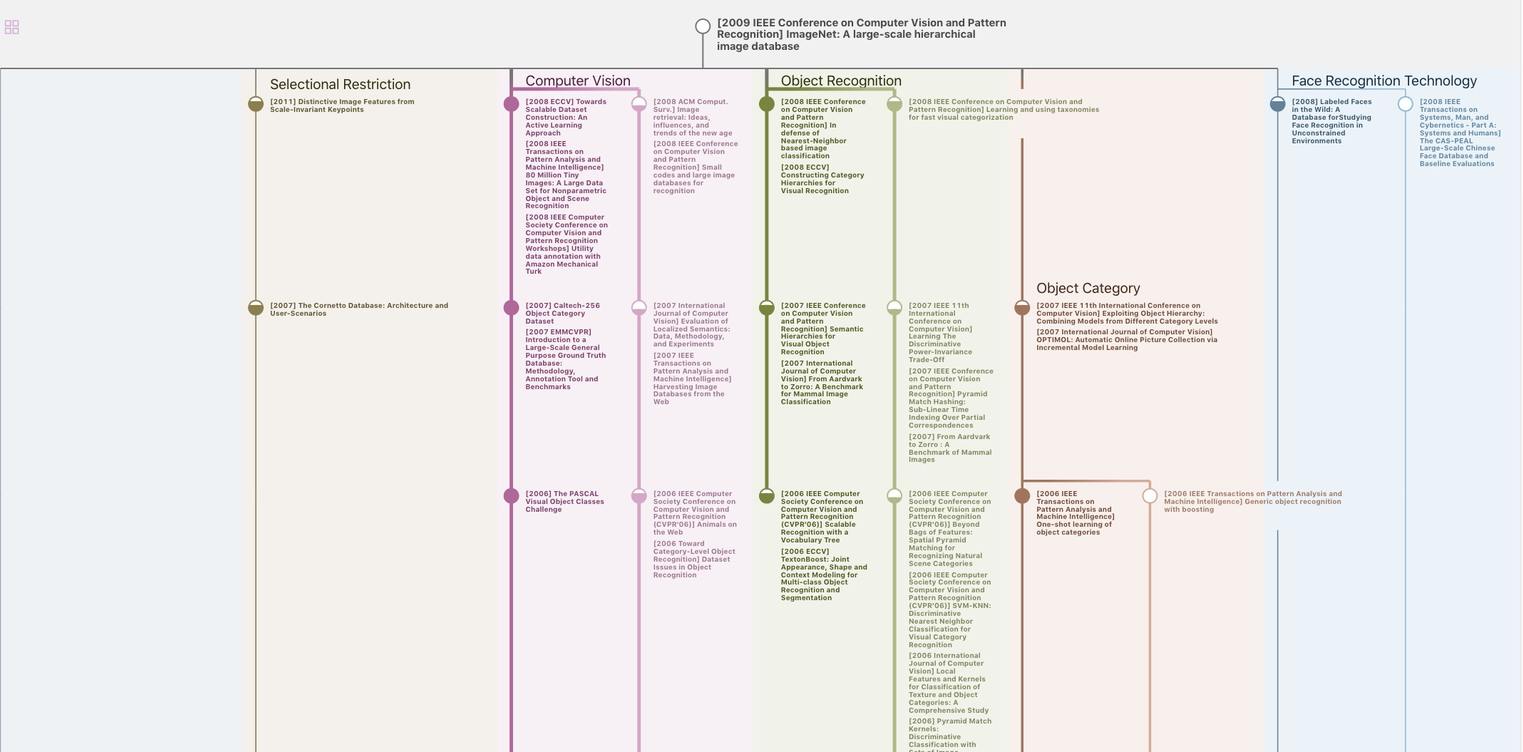
生成溯源树,研究论文发展脉络
Chat Paper
正在生成论文摘要