Fiber: A Platform for Efficient Development and Distributed Training for Reinforcement Learning and Population-Based Methods
arxiv(2020)
摘要
Recent advances in machine learning are consistently enabled by increasing amounts of computation. Reinforcement learning (RL) and population-based methods in particular pose unique challenges for efficiency and flexibility to the underlying distributed computing frameworks. These challenges include frequent interaction with simulations, the need for dynamic scaling, and the need for a user interface with low adoption cost and consistency across different backends. In this paper we address these challenges while still retaining development efficiency and flexibility for both research and practical applications by introducing Fiber, a scalable distributed computing framework for RL and population-based methods. Fiber aims to significantly expand the accessibility of large-scale parallel computation to users of otherwise complicated RL and population-based approaches without the need to for specialized computational expertise.
更多查看译文
关键词
reinforcement learning,fiber,training,distributed,population-based
AI 理解论文
溯源树
样例
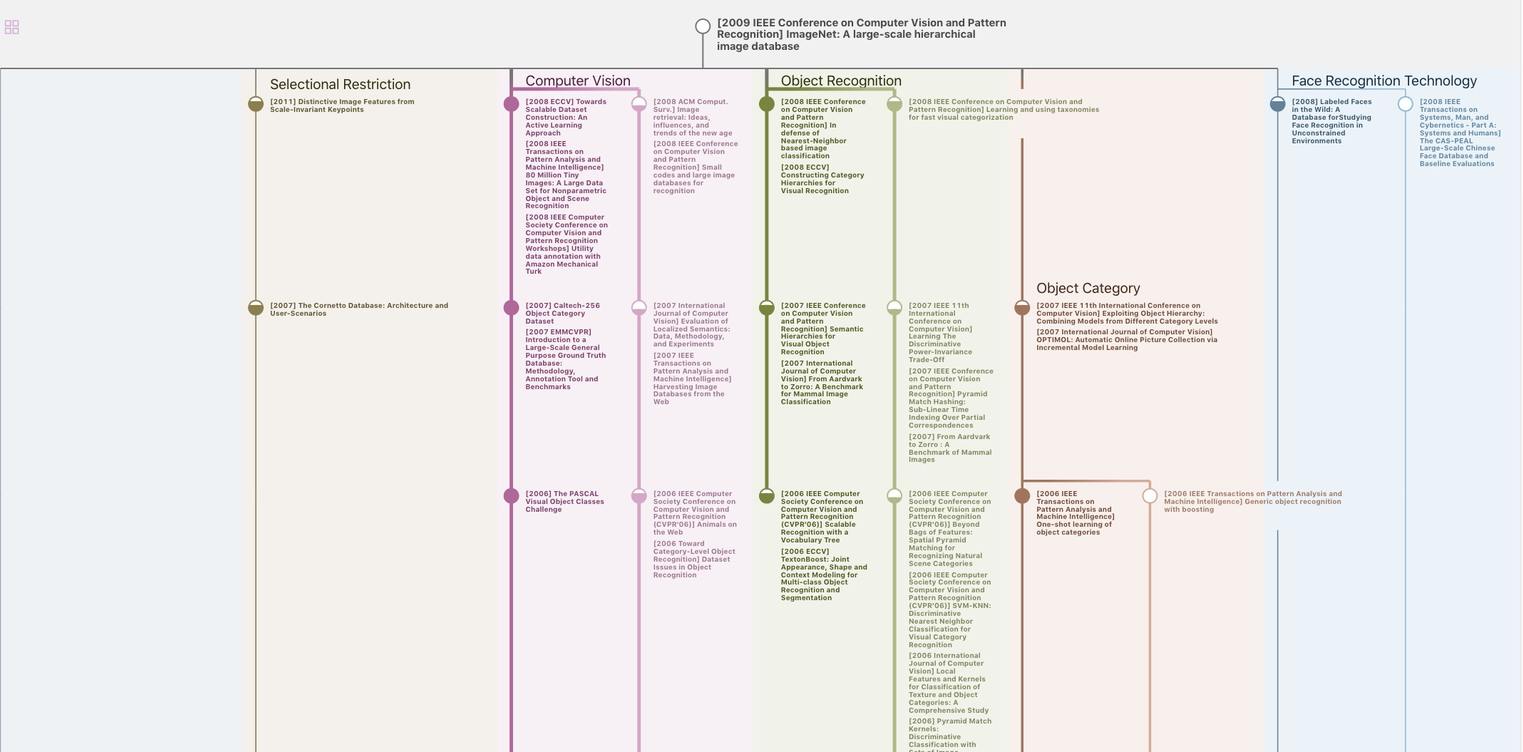
生成溯源树,研究论文发展脉络
Chat Paper
正在生成论文摘要