A Systematic Evaluation: Fine-Grained CNN vs. Traditional CNN Classifiers
ELECTRONICS(2023)
摘要
Fine-grained classifiers collect information about inter-class variations to best use the underlying minute and subtle differences. The task is challenging due to the minor differences between the colors, viewpoints, and structure in the same class entities. The classification becomes difficult and challenging due to the similarities between the differences in viewpoint with other classes and its own. This work investigates the performance of landmark traditional CNN classifiers, presenting top-notch results on large-scale classification datasets and comparing them against state-of-the-art fine-grained classifiers. This paper poses three specific questions. (i) Do the traditional CNN classifiers achieve comparable results to fine-grained classifiers? (ii) Do traditional CNN classifiers require any specific information to improve fine-grained ones? (iii) Do current traditional state-of-the-art CNN classifiers improve the fine-grained classification while utilized as a backbone? Therefore, we train the general CNN classifiers throughout this work without introducing any aspect specific to fine-grained datasets. We show an extensive evaluation on six datasets to determine whether the fine-grained classifier can elevate the baseline in their experiments. We provide ablation studies regarding efficiency, number of parameters, flops, and performance.
更多查看译文
关键词
fine-grained visual classification,traditional classification,systematic evaluation,deep learning,experimental review,baselines
AI 理解论文
溯源树
样例
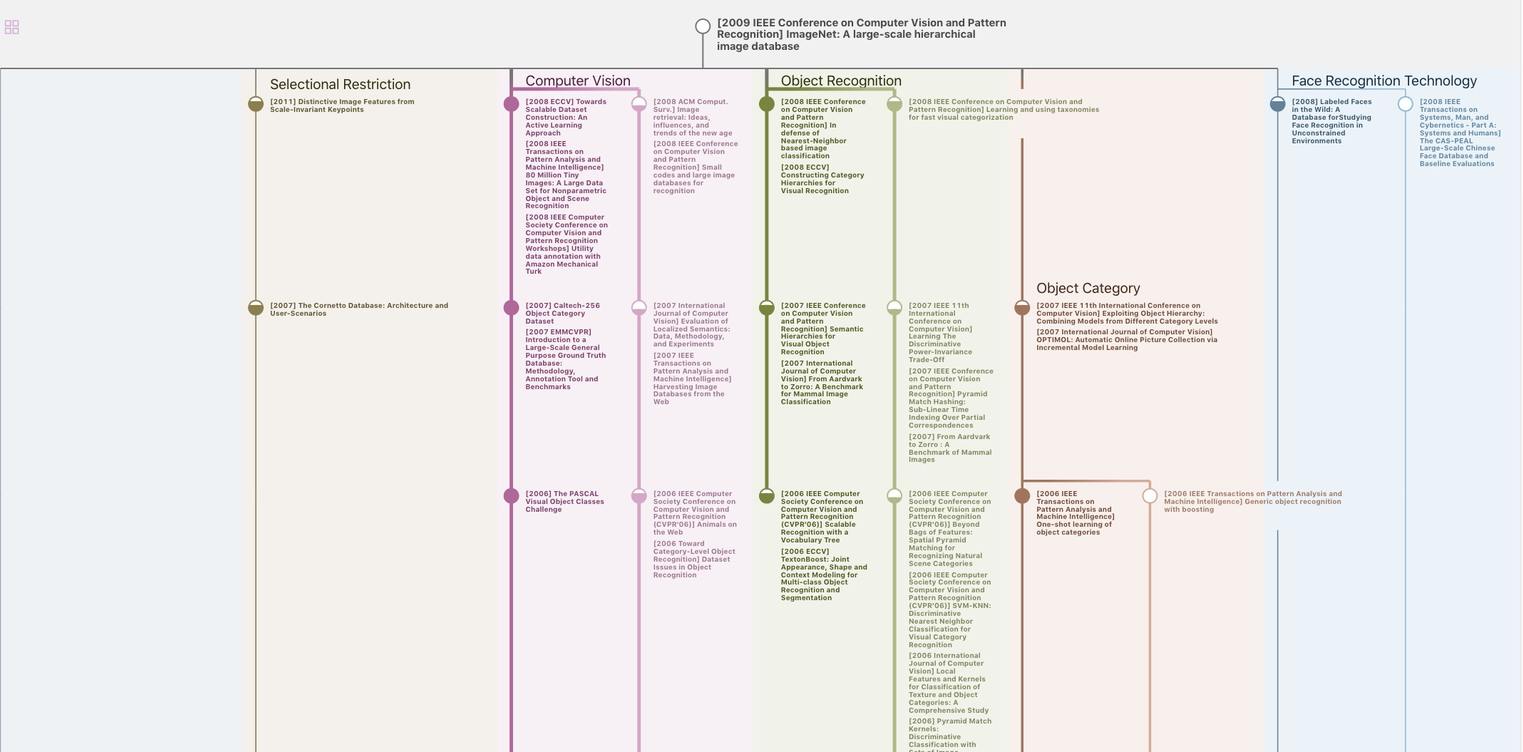
生成溯源树,研究论文发展脉络
Chat Paper
正在生成论文摘要