Per-Object Systematics Using Deep-Learned Calibration
SCIPOST PHYSICS(2020)
摘要
We show how to treat systematic uncertainties using Bayesian deep networks for regression. First, we analyze how these networks separately trace statistical and systematic uncertainties on the momenta of boosted top quarks forming fat jets. Next, we propose a novel calibration procedure by training on labels and their error bars. Again, the network cleanly separates the different uncertainties. As a technical side effect, we show how Bayesian networks can be extended to describe non-Gaussian features.
更多查看译文
关键词
calibration,per-object,deep-learned
AI 理解论文
溯源树
样例
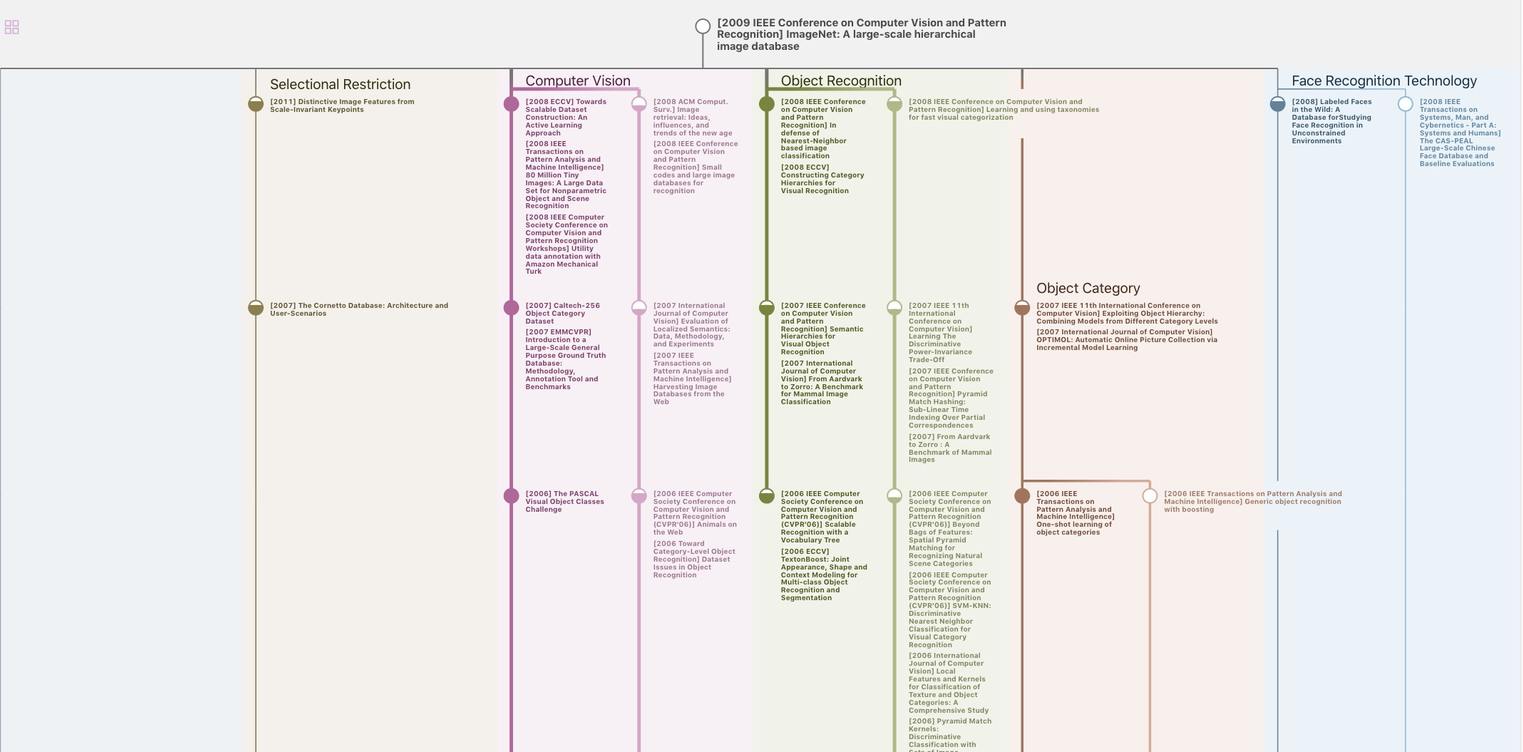
生成溯源树,研究论文发展脉络
Chat Paper
正在生成论文摘要