Sparsest Continuous Piecewise-Linear Representation of Data
arxiv(2020)
摘要
We study the problem of interpolating one-dimensional data with total variation regularization on the second derivative, which is known to promote piecewise-linear solutions with few knots. In a first scenario, we consider the problem of exact interpolation. We thoroughly describe the form of the solutions of the underlying constrained optimization problem, including the sparsest piecewise-linear solutions, i.e. with the minimum number of knots. Next, we relax the exact interpolation requirement, and consider a penalized optimization problem with a strictly convex data-fidelity cost function. We show that the underlying penalized problem can be reformulated as a constrained problem, and thus that all our previous results still apply. We propose a simple and fast two-step algorithm to reach a sparsest solution of this constrained problem. Our theoretical and algorithmic results have implications in the field of machine learning, more precisely for the study of popular ReLU neural networks. Indeed, it is well known that such networks produce an input-output relation that is a continuous piecewise-linear function, as does our interpolation algorithm.
更多查看译文
关键词
data,piecewise-linear
AI 理解论文
溯源树
样例
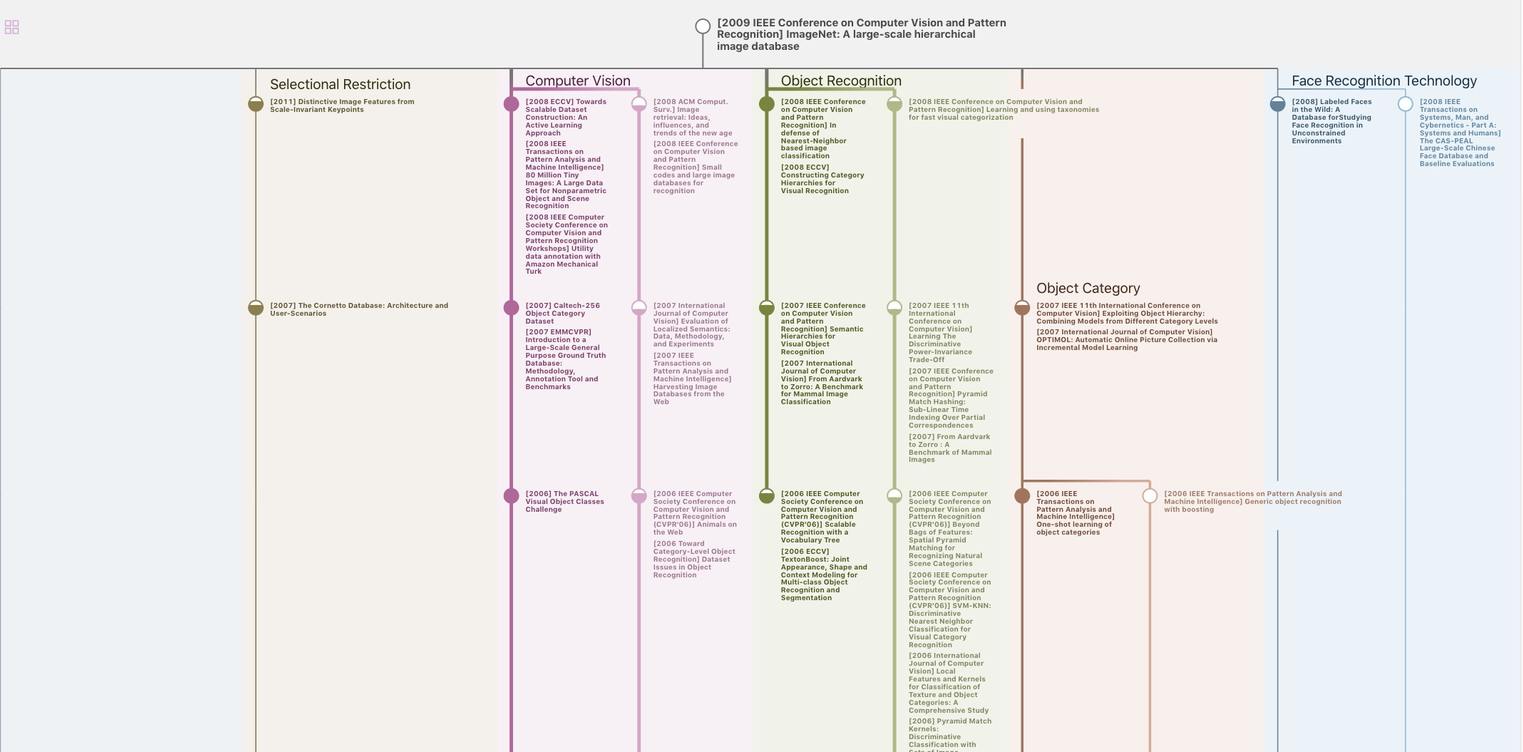
生成溯源树,研究论文发展脉络
Chat Paper
正在生成论文摘要