Cooling-Shrinking Attack: Blinding The Tracker With Imperceptible Noises
2020 IEEE/CVF CONFERENCE ON COMPUTER VISION AND PATTERN RECOGNITION (CVPR)(2020)
摘要
Adversarial attack of CNN aims at deceiving models to misbehave by adding imperceptible perturbations to images. This feature facilitates to understand neural networks deeply and to improve the robustness of deep learning models. Although several works have focused on attacking image classifiers and object detectors, an effective and efficient method for attacking single object trackers of any target in a model-free way remains lacking. In this paper, a cooling-shrinking attack method is proposed to deceive state-of-the-art SiameseRPN-based trackers. An effective and efficient perturbation generator is trained with a carefully designed adversarial loss, which can simultaneously cool hot regions where the target exists on the heatmaps and force the predicted bounding box to shrink, making the tracked target invisible to trackers. Numerous experiments on OTBI00, VOT2018, and LaSOT datasets show that our method can effectively fool the state-of-the-art SiameseRPN++ tracker by adding small perturbations to the template or the search regions. Besides, our method has good transferability and is able to deceive other top-performance trackers such as DaSiamRPN, DaSiamRPN-UpdateNet, and DIME. The source codes are available at https://github.com/MasterBin-IIAU/CSA.
更多查看译文
关键词
tracker,attack,cooling-shrinking
AI 理解论文
溯源树
样例
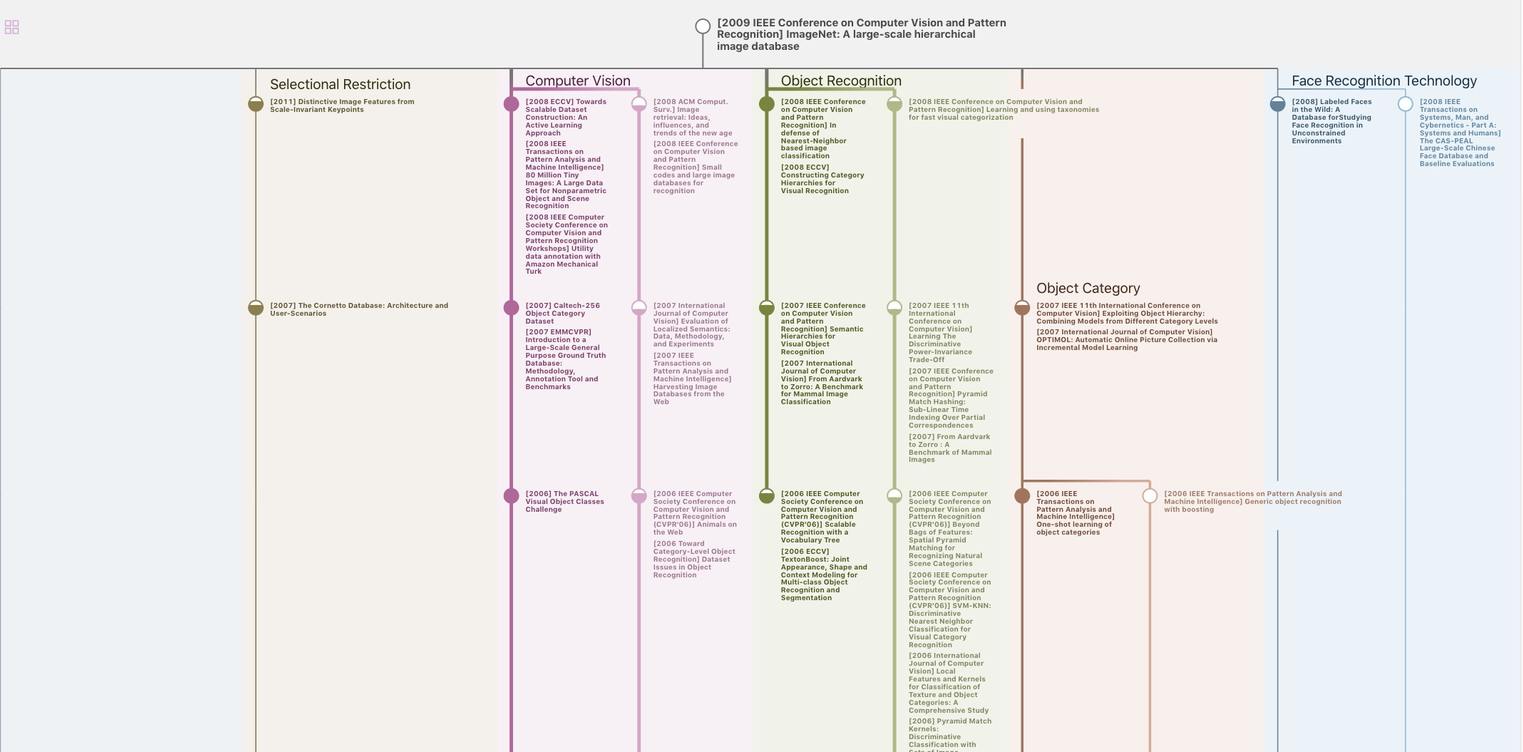
生成溯源树,研究论文发展脉络
Chat Paper
正在生成论文摘要