Enhancing Topical Word Semantic for Relevance Feature Selection.
SML@IJCAI(2017)
摘要
Unsupervised topic models, such as Latent Dirichlet Allocation (LDA), are widely used as automated feature engineering tools for textual data. They model words semantics based on some latent topics on the basis that semantically related words occur in similar documents. However, words weights that are assigned by these topic models do not represent the semantic meaning of these words to user information needs. In this paper, we present an innovative and effective extended random sets (ERS) model to enhance the semantic of topical words. The proposed model is used as a word weighting scheme for relevance feature selection (FS). It accurately weights words based on their appearance in the LDA latent topics and the relevant documents. The experimental results, based on 50 collections of the standard RCV1 dataset and TREC topics for information filtering, show that the proposed model significantly outperforms eight, state-of-the-art, baseline models in five standard performance measures.
更多查看译文
AI 理解论文
溯源树
样例
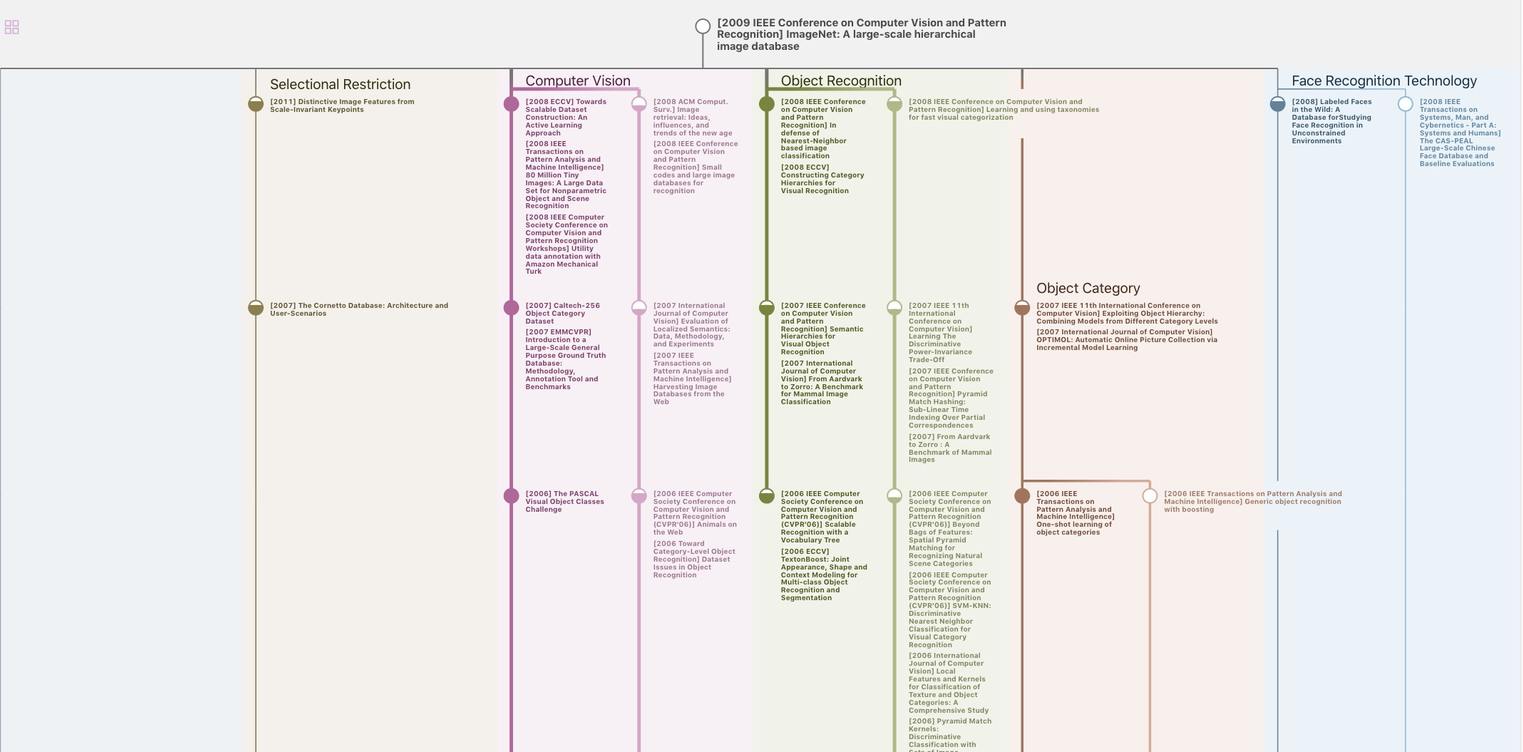
生成溯源树,研究论文发展脉络
Chat Paper
正在生成论文摘要