Privacy As A Service: Anonymisation Of Netflow Traces
ADVANCES IN E-BUSINESS ENGINEERING FOR UBIQUITOUS COMPUTING(2020)
摘要
Effective data anonymisation is the key to unleashing the full potential of big data analytics while preserving privacy. An organization needs to be able to share and consolidate the data it collects across its departments and in its network of collaborating organizations. Some of the data collected and the cross-references made in its aggregation is private. Effective data anonymisation attempts to maintain the confidentiality and privacy of the data while maintaining its utility for the purpose of analytics. Preventing re-identification is also of particular importance. The main purpose of this paper is to provide a definition of an original data anonymisation paradigm in order to render the re-identification of related users impossible. Here, we consider the case of a NetFlow Log. The solution includes a privacy risk analysis process to classify the data based on its privacy level. We use a dynamic K-anonymity paradigm while taking into consideration the privacy risk assessment output. Finally, we empirically evaluate the performance and data partition of the proposed solution.
更多查看译文
关键词
Privacy, Anonymisation, Risk analysis, NetFlow
AI 理解论文
溯源树
样例
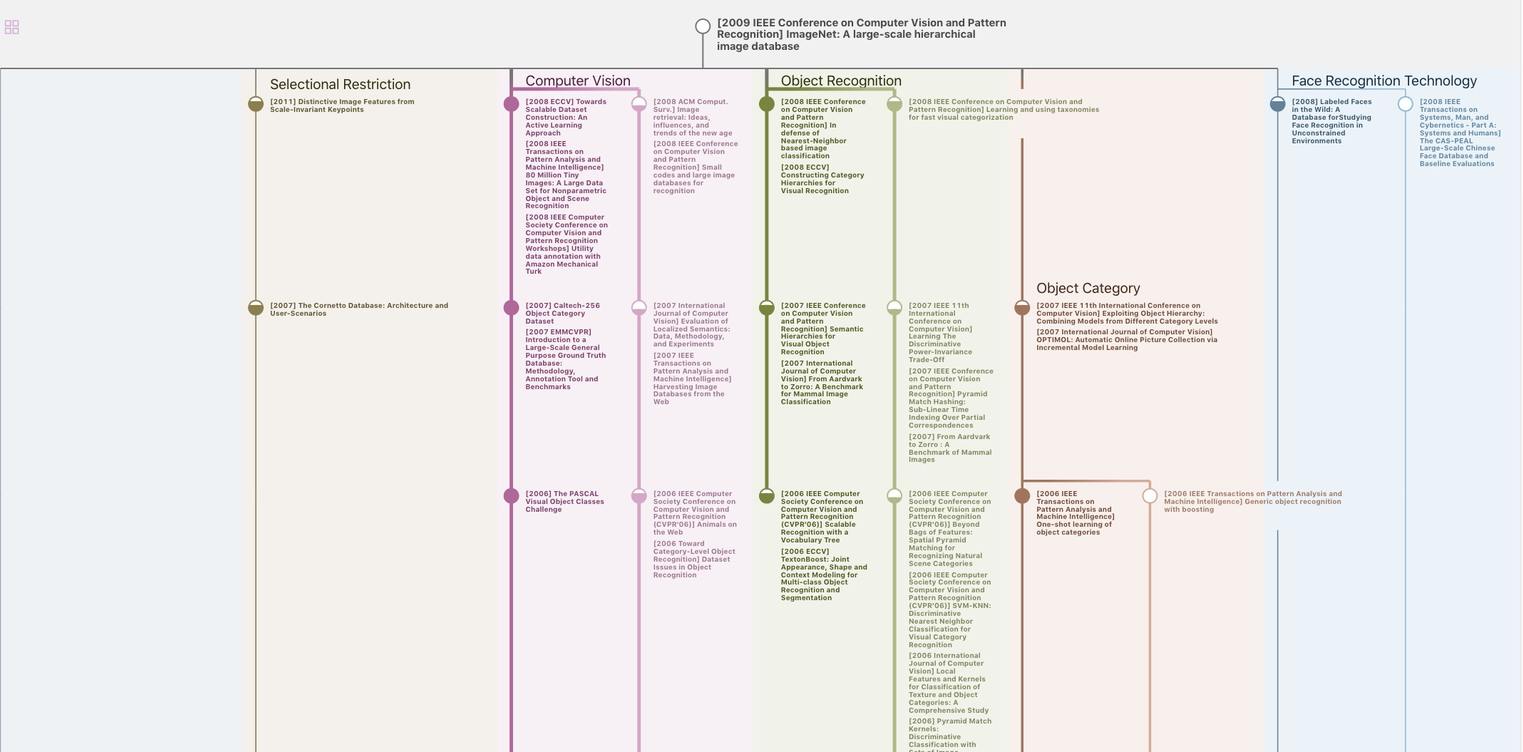
生成溯源树,研究论文发展脉络
Chat Paper
正在生成论文摘要