Multiple Machine Learning Algorithms Comparison for Modulation Type Classification for Efficient Cognitive Radio
IEEE Military Communications Conference(2019)
摘要
In this paper the potential of improving channel utilization by signal modulation type classification based on machine learning algorithms has been studied. The classification has been performed between two popular digital modulations: BPSK and FSK in target application. Classification was based on three features available on a popular software defined radio transceiver AD9361: in-phase and quadrature components of the digital time domain signal and signal-to-noise ratio (SNR), measured as RSSI value. Data used for network training, validation and testing was generated by the Simulink model consisting mainly of modulator, transceiver AD9361 and AWGN to generate the signal with SNR ranging from 1 to 30 dB. Twenty-three supervised machine learning algorithms including K-nearest neighbor, Support Vector Machines, Decision Trees and Ensembles have been studied, evaluated and verified against the target application's requirements in terms of classification accuracy and speed. The highest average classification accuracy of 86.9% was achieved by Support Vector Machines with Fine Gaussian kernel, however with demonstrated classification speed of 790 objects per second it was considered unable to meet target application's real-time operation requirement of 2000 objects per second. Fine Decision Trees and Ensemble Boosted Trees have shown optimal performance in terms of both reaching classification speed of 1200000 objects per second and average classification accuracy of 86.0% and 86.3% respectively. Classification accuracy has been also studied as a function of SNR to determine the most accurate classifier for each SNR level. At the target application's demodulation threshold of 12 dB 87.0% classification accuracy has been observed for the Fine Decision Trees, 87.5% for both Fine Gaussian SVM and Coarse KNN. At SNR higher than 27 dB Fine Trees, Coarse KNN have reached 97.5% classification accuracy. The effects of data set size and number of classification features on classification speed and accuracy have been studied too.
更多查看译文
关键词
machine learning,modulation classification,signal-to-noise ratio,software defined radio
AI 理解论文
溯源树
样例
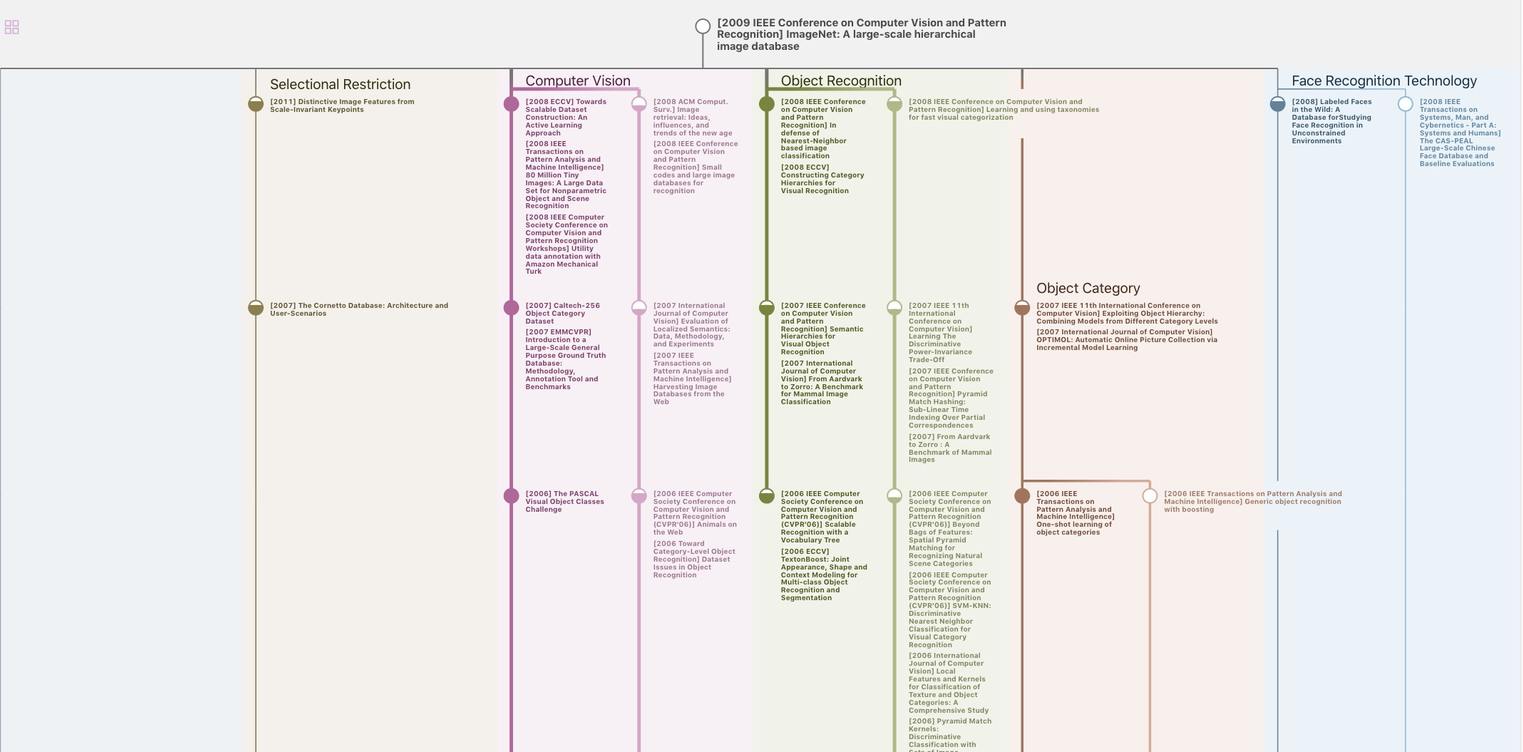
生成溯源树,研究论文发展脉络
Chat Paper
正在生成论文摘要